It won’t be long before there are more devices listening to us than there are people. Right now there are already over three billion devices in the world that you can talk into. At this point, every self-respecting tech giant has its own voice assistant. But behind each sleek, magic box is a gigantic infrastructure, as well as vast amounts of invisible labour. Last year alone, Amazon doubled the amount of employees dedicated to its Alexa products from 5,000 to 10,000.
The concept of the voice assistant is simple: you talk to your phone, computer, car, or other device, and it does what you tell it to do. But behind that friendly exterior of your “smart” device, your voice disappears into a labyrinth of invisible AI algorithms. It’s these algorithms that respond to your instruction, and enable your assistant to follow your commands – whether that’s calling mum or dimming the lights.
But make no mistake: this is just the beginning. Because what the tech giants are really interested in is combing our voices and our habits for data to learn what we want and predict what we need. To do that, the listening devices have to infiltrate deeper and deeper into our private lives and our daily interactions. They all do that as inconspicuous, obedient “assistants”, at your service, awaiting your command. Except that these assistants are wired. All the time. Because even if those microphones are only on when you speak to them, as the big manufacturers claim, those microphones are still active, and increasingly surrounding us all.
How much of our day-to-day auditory environment we’re willing to give away is something that we largely get to decide ourselves – for now. But in a world that will soon have more artificial ears than human ears, it’s going to get very hard to keep track of who’s listening.
Right now, we can still question the design choices behind these devices, the way they direct our interactions, or how they embed themselves into our personal spaces. But many of us already spend our days within constant earshot of something that listens to us, all day, every day, from the moment we wake up until the moment we go to sleep. So now is a good time to start thinking about what that “something” is, how you talk to it, and how it listens.
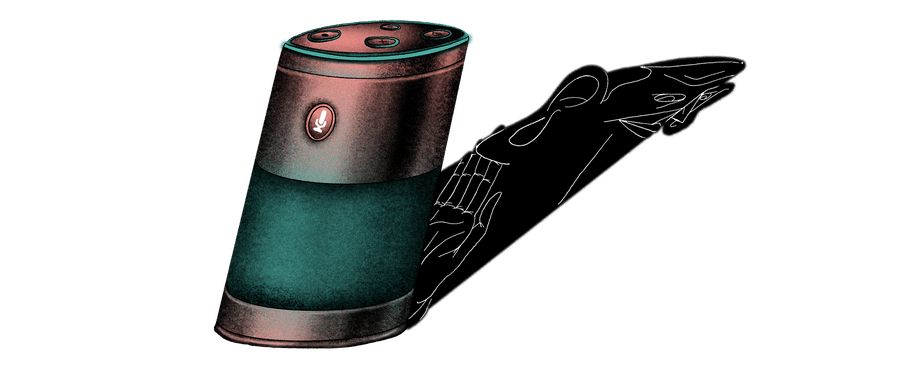
OK Google, how exactly are you listening to me?
To understand what happens with your voice when you talk to devices like these, you first need to know how these machines listen.
Let’s say I can’t be bothered to type right now, so I say something simple to my laptop: “What is The Correspondent?”
When I speak, I make sounds that get picked up by an internal microphone in the computer. There, the sound of my voice is converted into a digital signal, and then broken down into bite-sized chunks: w-u-t i-z th-uh k-oh-r-eh-s-p-AU-n-d-e-n-t.
But digital audio is unwieldy and difficult to analyse. So these speech chunks are converted into a much more efficient, standardised data format: text. You can see this process in action when you talk to a voice assistant like Siri on your phone: the words you’re saying appear on the screen as the voice assistant recognises them.
For a long time, the almost infinite complexity of speech variation stumped speech recognition developers
But no one talks exactly like anyone else. Dialect, pronunciation, speed, accent, intonation and vocabulary vary enormously between individuals – and that’s not even to get into things like sarcasm and nonverbal communication, a whole different layer of complexity in the ways we use language. For a long time, the almost infinite complexity of this variation stumped speech recognition developers. But then they came up with a clever solution: instead of trying to teach the computer every aspect of language in all its complexity, they started using statistics.
In the case of voice assistants, this primarily comes down to two systems, both of which are driven largely by artificial intelligence (AI). One recognises speech and links speech chunks to their most likely letters, sounds and words. The other interprets said speech by calculating the highest probable sequence of words.
All voice assistants use a form of pattern recognition like this. It’s similar to the way that YouTube can predict things that you’ll probably like on the basis of what you (or all the many others who watch things like you do) have watched before; speech recognition devices try to predict what you’re most likely to say on the basis of what you (or all the many others who say things like you do) have said already.
And your responses, just like your clicks and your viewing time on YouTube, are also data, which is then used to make even better predictions. This process as a whole is also referred to as machine learning: the idea is that if you give the program enough correct data – enough different “cor” sounds – it will eventually be able to improve itself until it is ultimately able to recognise your “cor” sound out of thousands, or even millions.
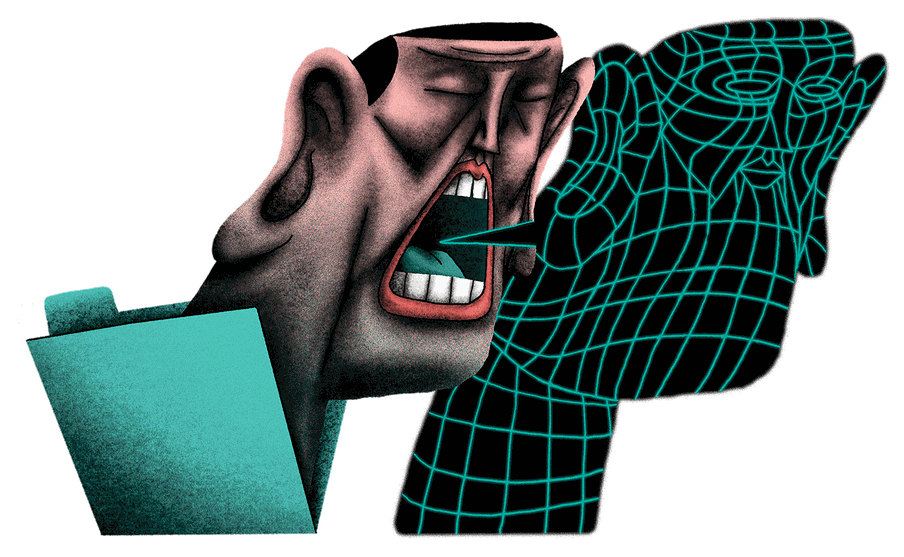
What happens to the speech chunks that you send into Alexa, Siri and Google?
But there’s another question: where does all this happen?
Serf Doesborgh and Jurriën Hamer, researchers with the Rathenau Instituut, in The Hague, explain this to me in a video call. Doesborgh says that voice assistants rely on what he calls “wake words”: “Hey Siri”, “OK Google”. “The voice assistant is always on, although it throws away all the recordings that don’t include a wake word. When you activate the assistant, your speech recording goes straight to the cloud, to the server of the company that made the software, and that’s where all the analysis happens.”
This also answers the question of where your voice goes when you talk to your voice assistant. That voice recording gets ground down into readable data, before ending up somewhere on a server, where the unique features of your voice are connected and compared to as many other speech chunks as possible to make the whole process run even more smoothly.
Doesborgh explains that the way the technology works now, there is still a very important role reserved for people, who train the software – but that inevitably means listening in. “The best algorithms and the smartest AIs have to be fed,” says Hamer. “And controlled.”
My voice recordings might also end up in the headphones of some ordinary person, in a dreary office cubicle
So my voice recordings might not just disappear into the endless labyrinth of a server somewhere. They might also end up in the headphones of some ordinary person, in a dreary office cubicle, who listens to and corrects hundreds of voice recordings just like mine every day.
In 2019, it was revealed that Amazon, Apple and Google were all listening to voice recordings of their users without clear consent – often even if those users had not spoken the "wake word". There was a case of a temporary worker in Belgium, grading recordings outsourced by Google, who had eavesdropped on recordings of domestic violence, while others had heard medical histories, financial information, sex sessions and drug deals. What followed was predictable: users shocked, tech giants going into apology mode, privacy settings frantically being updated.
Now, here we are, one year later: Siri is up to 25 billion requests per month and hundreds of millions of so-called smart devices have entered homes around the world, despite a global pandemic. Devices that, at least for the moment, are still dependent on people who help them sort through and classify their recordings of the human voice.
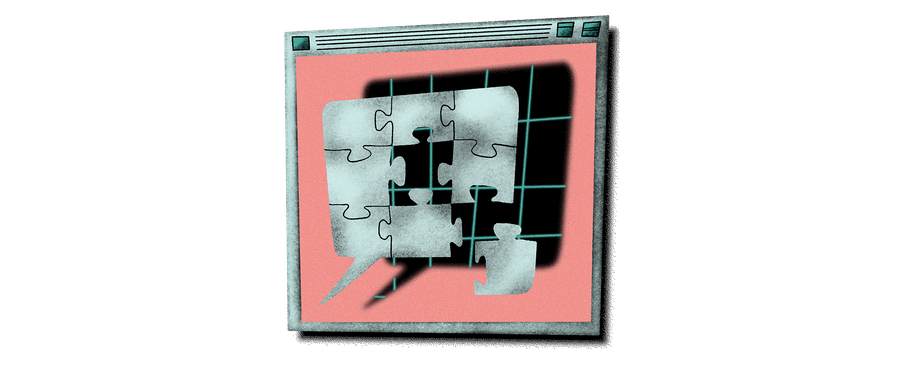
What’s in a voice?
Doesborgh and Hamer say that the more privacy-friendly developments are a step in the right direction but are still based too much on a hypothetical “ideal user” who is both well-informed and actively engaged in protecting their own privacy. “And whether people really understand the privacy considerations or not,” continues Doesborgh, “if people feel like they’re being monitored, they are always going to end up adjusting their behaviour, which means some freedom of self-expression will be lost.”
But voice technology also introduces an entirely new privacy risk. “What’s very exciting, and worrying at the same time, is that soon they’ll be able to collect not just text information, but also the sound of my voice,” says Hamer. “And all the things they can get out of that.”
Tech companies are working on figuring out all the possible things they might be able to glean from the sound of your voice
Right now, tech companies are working on figuring out all the possible things they might be able to glean from the sound of your voice: your sex, age, body size, whether you’re angry or frustrated (for now, only Amazon’s Alexa can do that, and only in English). Whether you’re suffering from dementia, Parkinson’s disease, depression, PTSD, a brain tumour, or even coronavirus. The potential applications of this kind of biometric analysis of the voice in healthcare, education, tourism, retail, call centres, advertising and identification processes are endless.
Hamer is quick to add that we are by no means there yet but cautions: “We are well on our way towards a society in which big tech companies want to know all this about all of us. And it’s entirely possible that in just a few years, they will be able to.”
Now what?
The researchers call for stronger governmental regulation, which could take many forms: for example, only allowing specific institutions like hospitals or banks to perform biometric analysis, and only if the institution can prove that it can handle this responsibility.
They also suggest that national governments invest in freely accessible voice databases for the languages spoken in their territories, which would enable smaller companies and private users to train their speech software in their own language. This might also provide something of a response to the current dominance of big tech companies over speech technology infrastructure, as well as enable higher quality research.
For Doesborgh and Hamer, the bottom line is calling attention to the big question about the future of talking devices: at that point in the future when every human interaction has to pass through a computer, what will we have lost?
Even though robots aren’t yet able to accurately analyse our deepest feelings, Hamer says that the use of these technologies may already be having a substantial impact on our society. “Let’s say that in a job interview, an employer uses emotion recognition on a voice clip. That will have real consequences if it means that someone does or does not get the job. And many companies who sell the software aren’t particularly keen on disclosing what the margin for error in their method is.”
Whatever information is stored within the sound of our voices, and whatever it may say about us, remains to be seen. What we do know is that there are many areas in which major efforts are being made to “humanise” technology, and these depend on human choices and, more importantly, a great many human examples.
Anything that helps me use my phone more efficiently is all well and good, but we need to know what these devices are doing with our voices. For now, we would do well to remember that they’re designed as a listening ear, always alert, always awaiting our next command. And always hungry for more.
Translated from the Dutch by Kyle Wohlmut.
Dig deeper
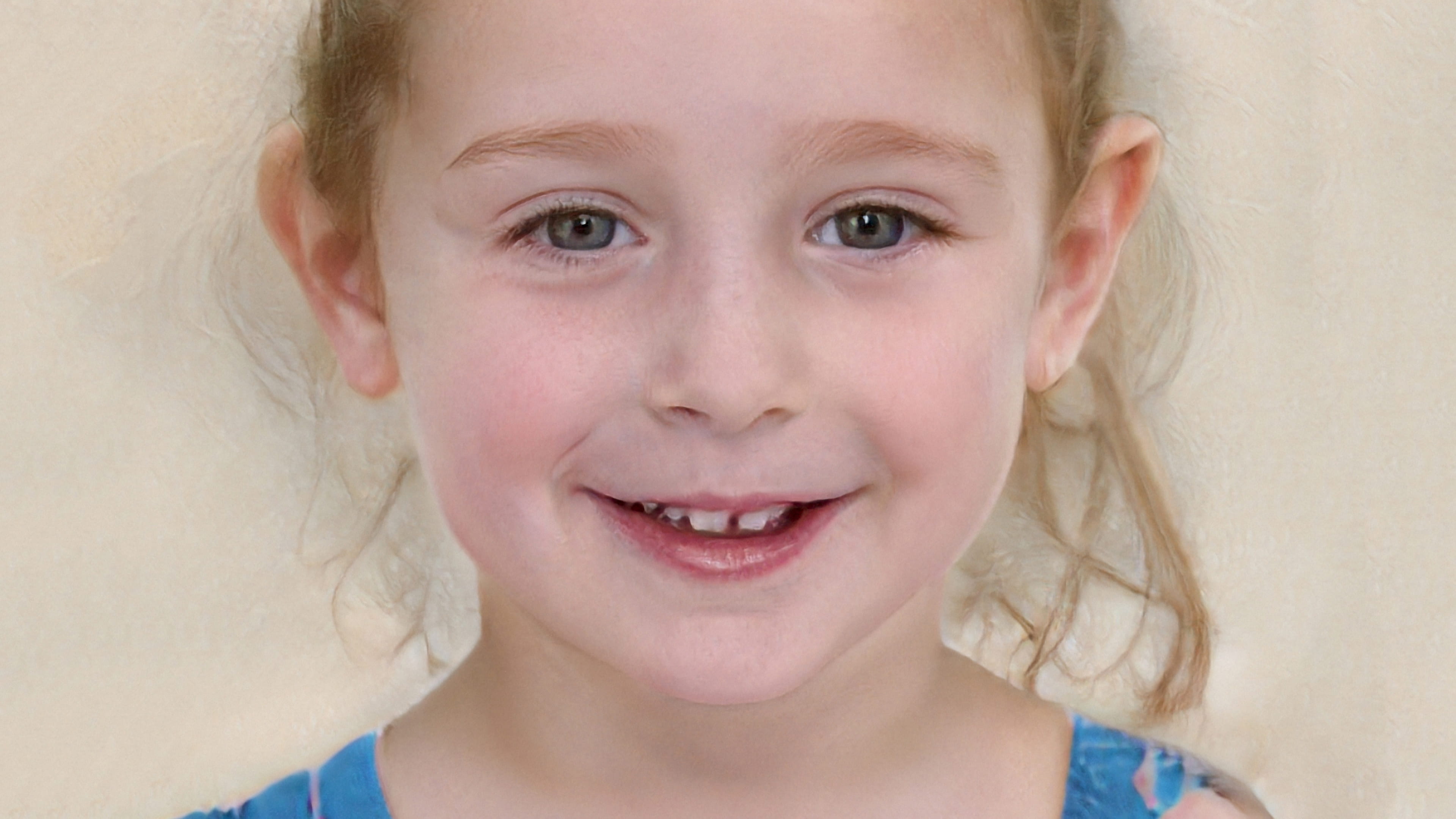