The tech magnate Elon Musk wants to achieve ‘a sort of symbiosis between human and artificial intelligence’, he said recently in a presentation of his company Neuralink.
The idea: wires with electrodes thinner than a human hair are implanted in the brain to steer an artificial limb, for instance, or to heal a neurological disease. Neuralink is testing the technology on rats and wants to start using it for people at the end of 2020, says Musk.
Musk’s story – no surprise here – is rather extreme. It seems optimistic to expect to get the go-ahead for human experiments from the Food and Drug Administration as early as next year. But this news is certainly part of a larger trend: you hear a lot about artificial intelligence these days.
AI startups are attracting funding like never before, and governments are falling over each other with their ambitions. If the hype is to be believed, AI is going to penetrate every facet of our lives. It’s going to detect our tumours, drive our cars and fight our wars. Some say it can solve our major problems – from climate change to death – others but that it might also be our ‘last invention’.
So everyone is talking about it. But do we really know what it is we are talking about?
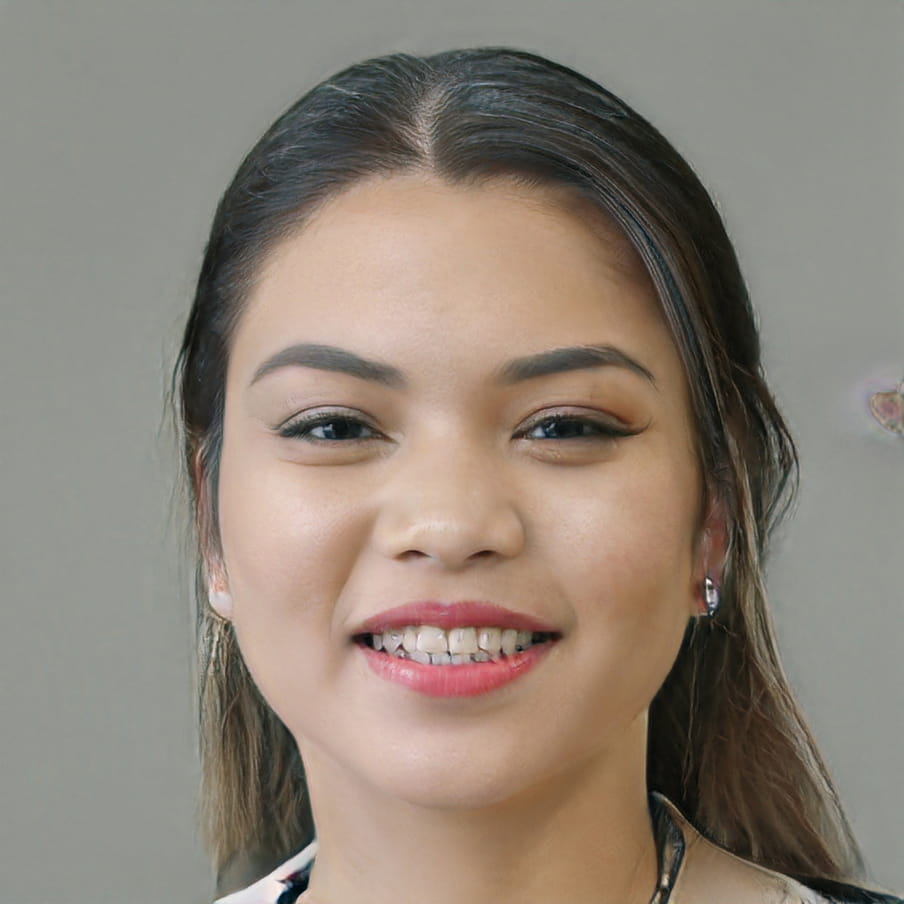
What is artificial intelligence?
Opinions vary as to what we mean by ‘artificial intelligence’. Some define it so narrowly that it only covers self-learning robots, while other definitions are so broad that even very simple statistical models are called AI.
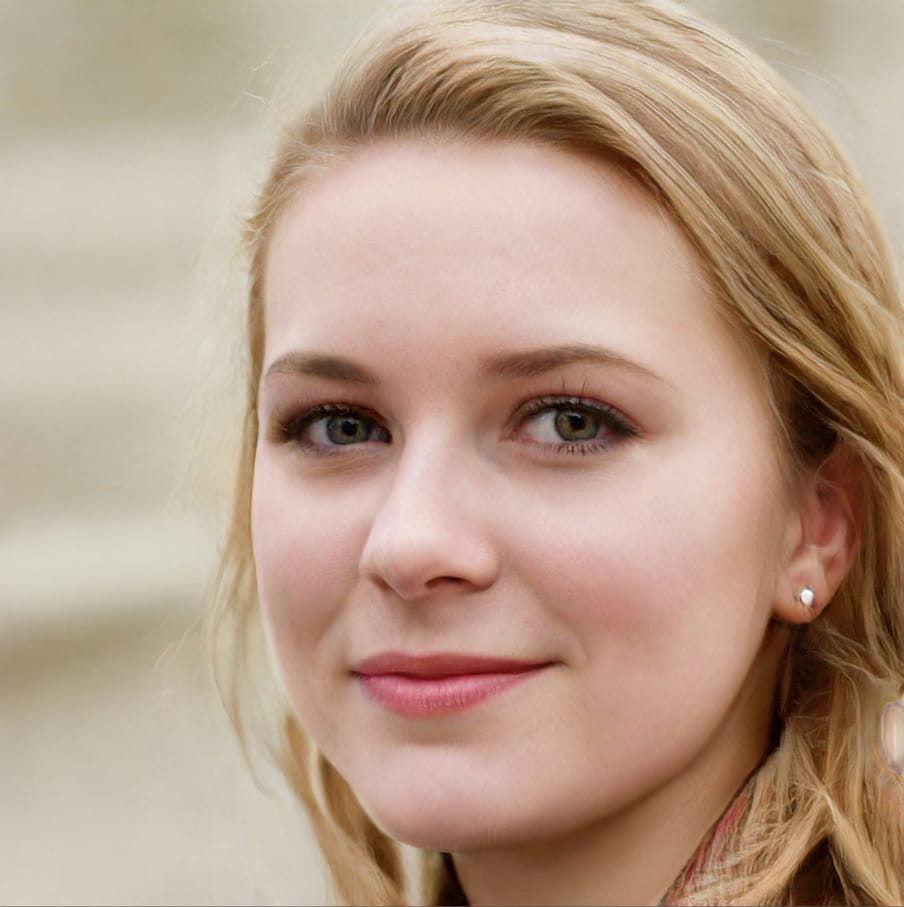
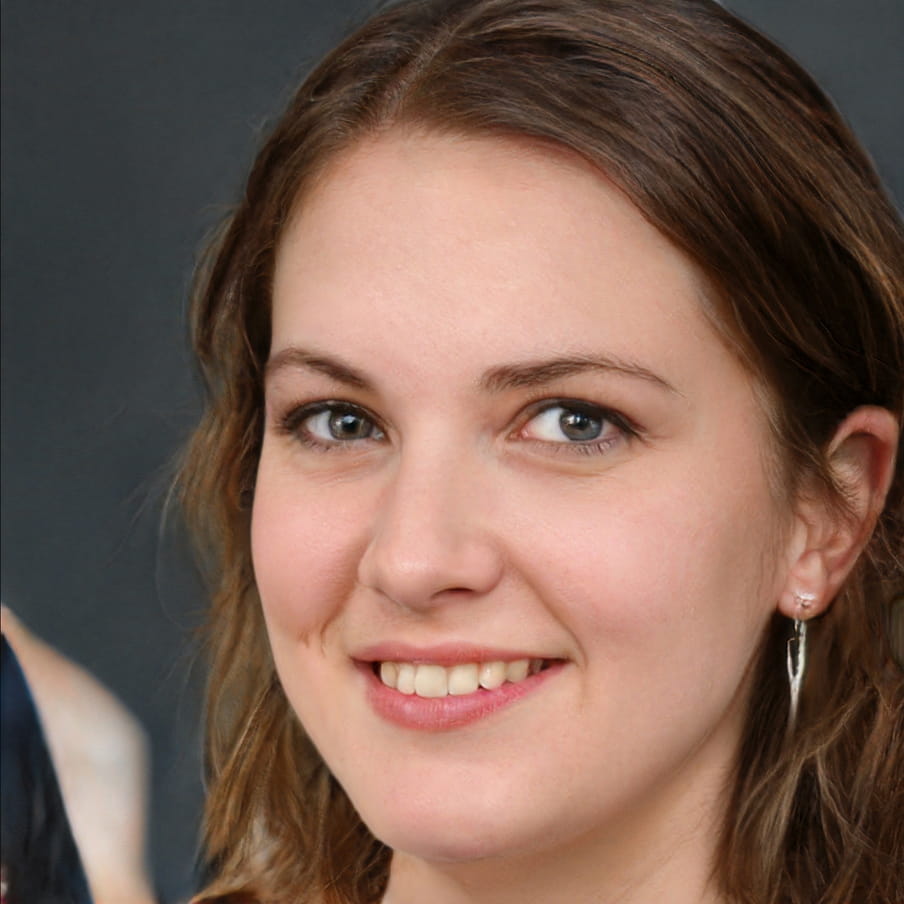
There is a lot of hype around AI, so the term gets used for a wide range of things. AI generates research grants, investments and media attention. And when there’s money to be made, people tend to exaggerate.
One example is Mike Mallazzo, who recently wrote about his job at the company Dynamic Yield, a self-styled ‘AI-powered Personalization Anywhere™ platform’ that was bought by McDonald’s for 300 million dollars. But what Dynamic Yield does is not AI at all, says Mallazzo. It is in the business of ‘personalisation’ – when you are recommended a McFlurry at McDrive on a hot day, or when the self-service kiosk knows about your penchant for a McChicken.
All this means is that the startup is good at solving the “very dull and ubiquitous problem of personalising data management”.
The company was perfectly aware of this, but they kept getting the AI label slapped on what they do. “The market wanted us to be an AI company so we chuckled and decided to call ourselves one.”
Is there really no definition of AI out there?
Definitions certainly exist. There is this one is on Wikipedia, for instance: “intelligence demonstrated by machines, in contrast to the natural intelligence displayed by humans.” Another description is “The capacity of a machine to imitate intelligent human behaviour.”
And that’s where the problem lies: what is “intelligent human behaviour”? There was a time when we thought it was amazing that a calculator could do a difficult sum. And then, that a computer could win a game of chess. But not everyone would call that AI nowadays.
This is the ‘AI effect’: once we know how to get a computer to do something, the magic is gone. It’s normal; what’s so intelligent about it? As Douglas Hofstadter wrote in Gödel, Escher, Bach: “AI is whatever hasn’t been done yet.”
You can question the term ‘artificial’ too. It suggests a clear difference between human and machine, but what if a human being was no more than a complex computer? Where is the border between artificial and human intelligence?
One way or another, the attempt to define AI raises several philosophical issues and doesn’t provide a clear-cut answer.
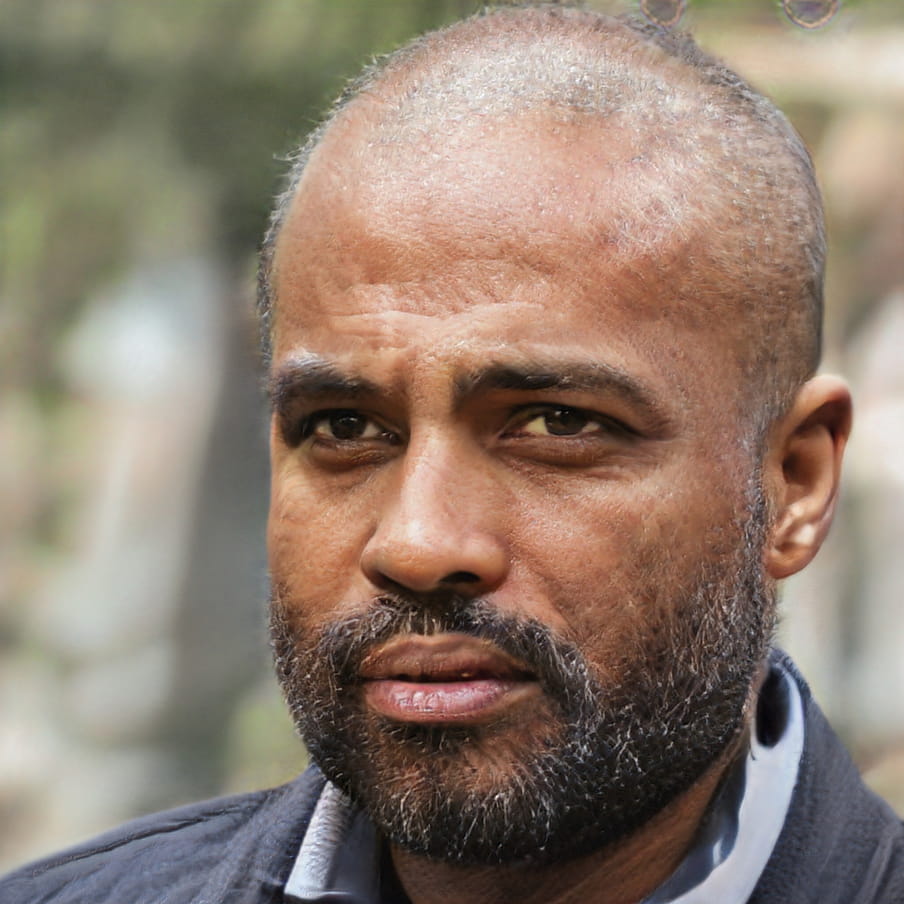
So when I read something about AI, what does it mean?
Most of the AI you hear about is machine learning: the use of algorithms and statistical models to get a machine to carry out a task without giving it explicit rules. Imagine you’re teaching a computer to recognise a cat. You can make a number of rules. Does this object have four legs? Fur? Pointy ears? Tricky, because even after a ‘yes’ to all three questions, it could still be a dog. And some cats are three-legged, bald or have folded ears.
These are things young children pick up easily, but computers find quite difficult. And this is where machine learning comes in: instead of giving the computer endless ‘if this, then that’ options, you get it to learn by itself. With a neural network, for example.
A what?
Neural networks are all the rage in the AI world. A lot of what you read about AI is about neural networks. They are used in self-driving cars, in the speech recognition on your phone, and in Google Translate.
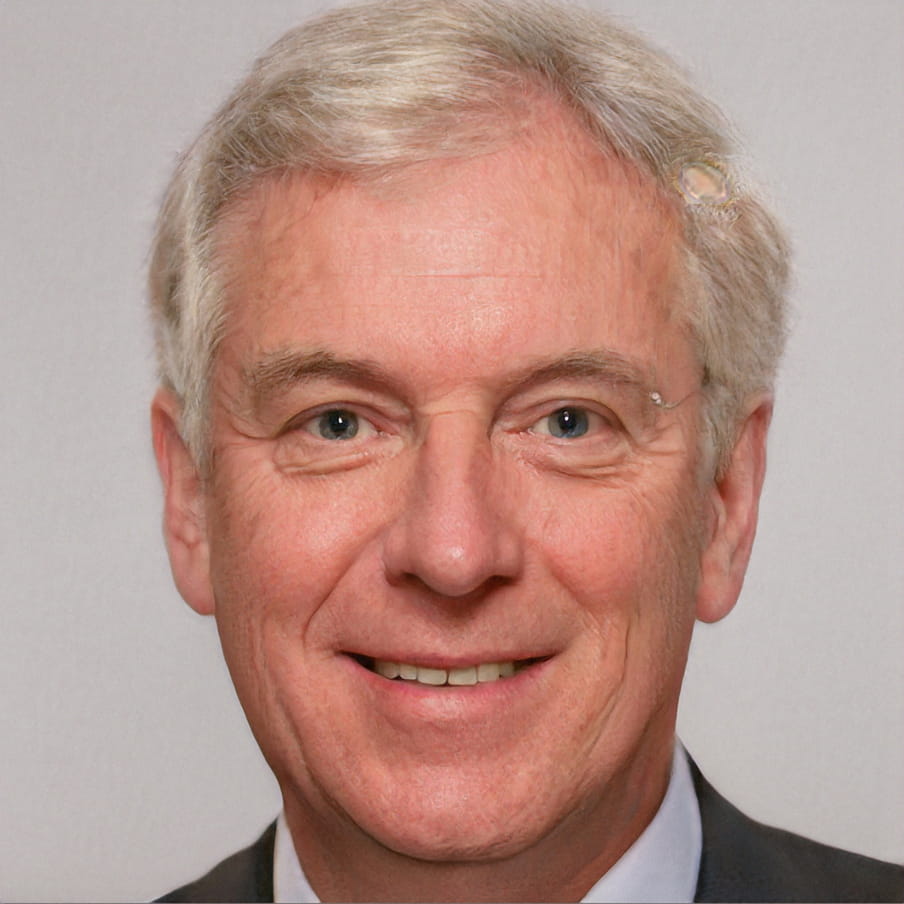
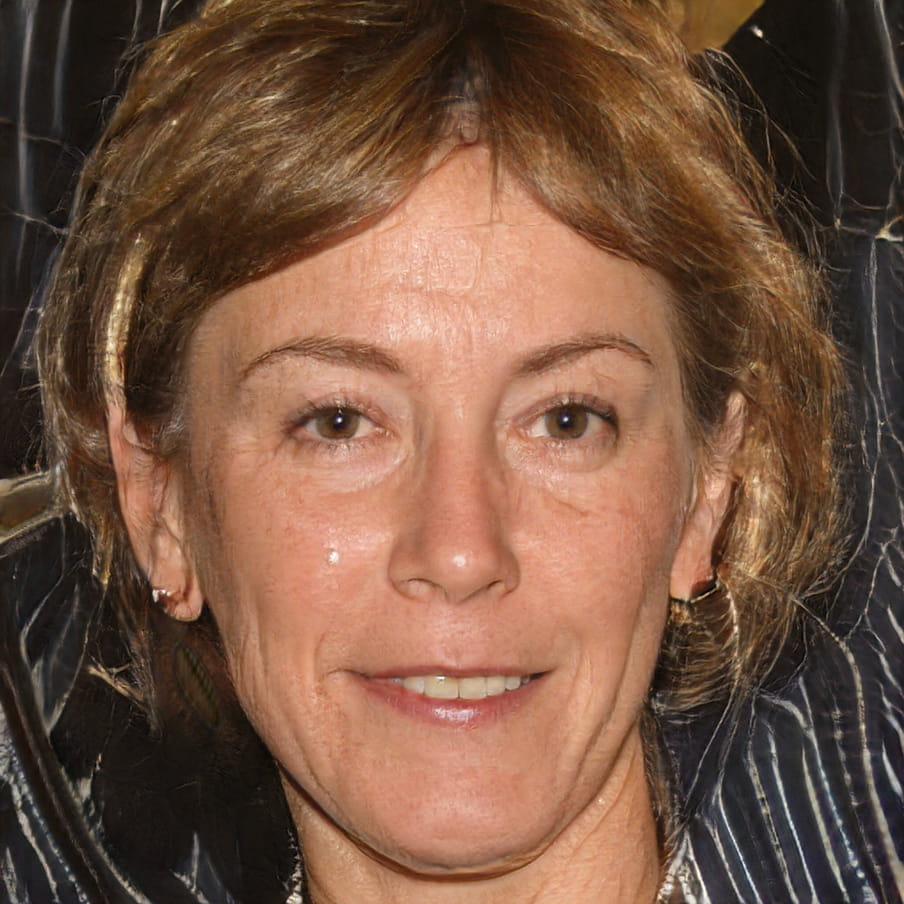
And do take a look at This Person Does Not Exist, where the illustrations for this article are taken from. On this website, you’ll find photos of people who don’t exist, generated instantly using artificial intelligence. Courtesy, again, of those neural networks.
(If you’re enjoying yourself, try This Cat Does Not Exist as well: it doesn’t work as well but it’s very funny.)
This might all seem very 21st century, but the foundations for neural networks were actually laid back in 1943, when neurophysiologist Warren McCulloch and logician Walter Pitts created the first artificial neuron, inspired by the brain. Rather like a switch in an electric circuit, a neuron could turn on and off, passing on or withholding information.
The idea of a neural network has been further developed in the decades since the 1940s. But it is only in the past few years that we have really been able to do something with those neural networks, as until recently we didn’t have enough calculating power and data.
How does a neural network work?
Imagine we want to use a neural network to recognise whether there is a cat in a black-and-white photo.
The first building block is the input, in this case the photo — or more precisely, all the pixels in the photo. They form the input for the ‘neurons’ in the first layer of our network. They are no longer either on or off, as they were for McCullough and Pitts, but some are more switched-on than others. In mathematical terms: each neuron has a number between 0 and 1, a code which tells us how black (0) or white (1) the pixel is.
The second building block is the output: is there a cat in the photo, yes or no? This is a matter of a single neuron that is either on (cat) or off (no cat). Here, too, the neuron can acquire a value, in this case between 0 and 1. If that value is 0.8, the model is 80% sure there is a cat in the photo.
The third building block is the network of layers of neurons between the input and the output. This is why the use of neural networks is also known as deep learning, because the information goes through several layers.
Once again, every neuron is ‘on’ to a particular extent, which determines the amount of information it passes on to a neuron in the next layer. One neuron might collect all the pixels around the cat’s nose, while the next one looks for lines, and the neuron after that identifies them as whiskers.
But the point is that you don’t have to tell the computer what each layer should examine. It figures that out for itself by ‘training’. You feed it a whole lot of labelled photos (cat/no cat), and let this system test the networks to see which one gives the best results. If it gets – say – 90% of the photos wrong first time around, it can adjust how the neurons pass on information so that it works better the next time.
This is just an example. If you want to understand neural networking in more detail, the YouTube channel 3Blue1Brown has made an accessible series about the method. Watch the first episode here:
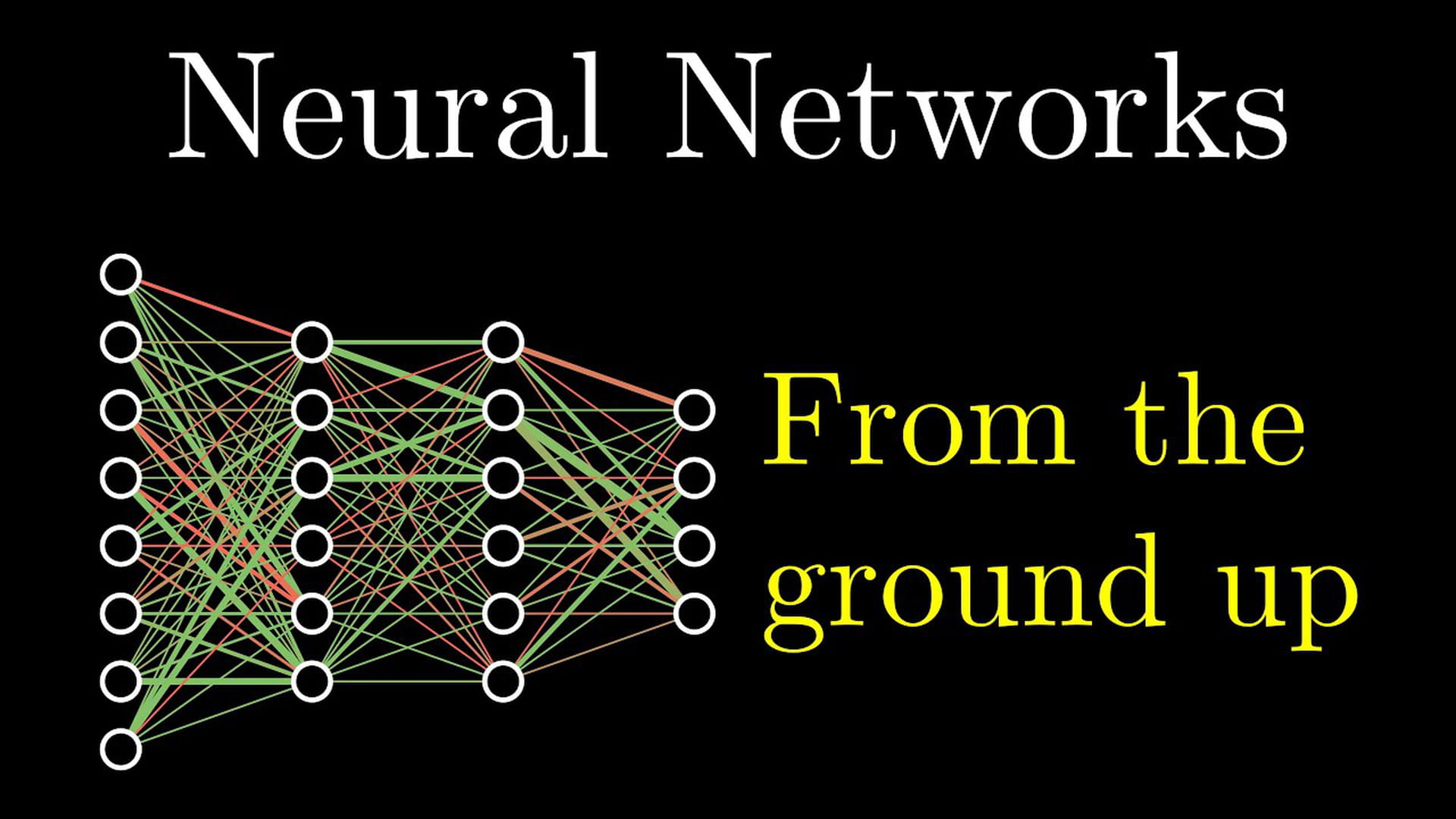
And how well does it work?
In 2015, the European Go champion Fan Hui played an unusual opponent. The board game Go is known for the enormous number of moves that are possible in it: more than there are atoms in the universe. While you can give a chess computer a number of conditions that are likely to help it to win, there is no way you can do that with Go.
Enter neural networks. AlphaGo, the network developed by Google DeepMind, thrashed Hui 5-0 in 2015. When the programme played Lee Sedol, who had been world champion 18 times, it won 4-1.
AlphaGo is probably AI’s best-known success story. But there are others too: models that can detect cancer cells, models that can forecast the weather, and - yes - models that can predict what you want to see next on Netflix.
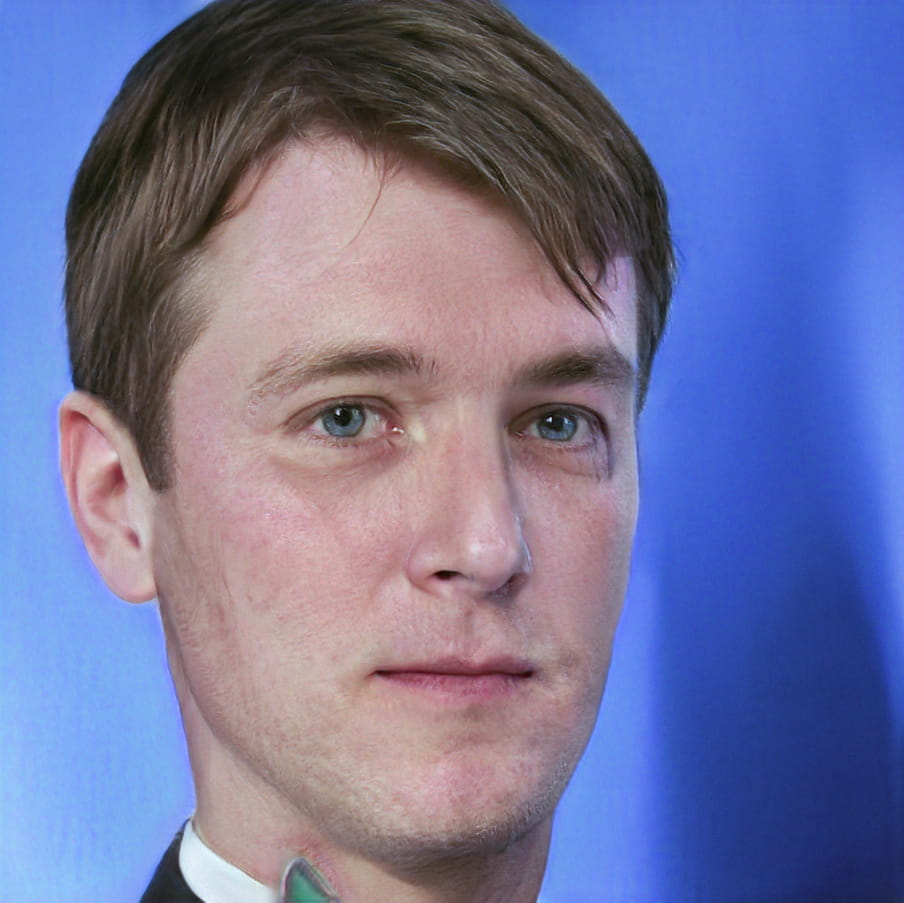
It’s all good news, then?
Not quite. When developer Jacky Alciné was scrolling through Google Photos one evening in 2015, at first he was impressed. Google had tagged his photos incredibly accurately: a photo with a plane in it was labelled ‘plane’, a bike was labelled ‘bike’ and his brother in a mortar board ‘graduation’.
Until he got to a selfie taken with a friend, both of them African American. ‘Gorillas’, said the label. He scrolled on to other photos from that day. Gorillas, gorillas, gorillas.
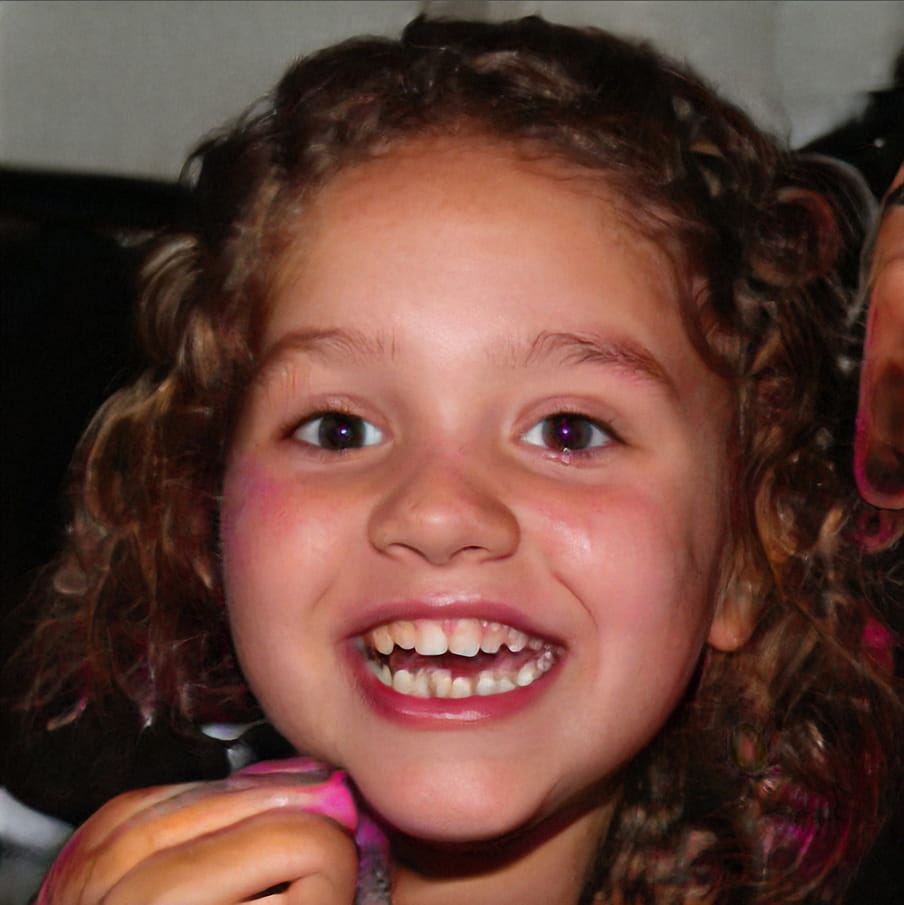
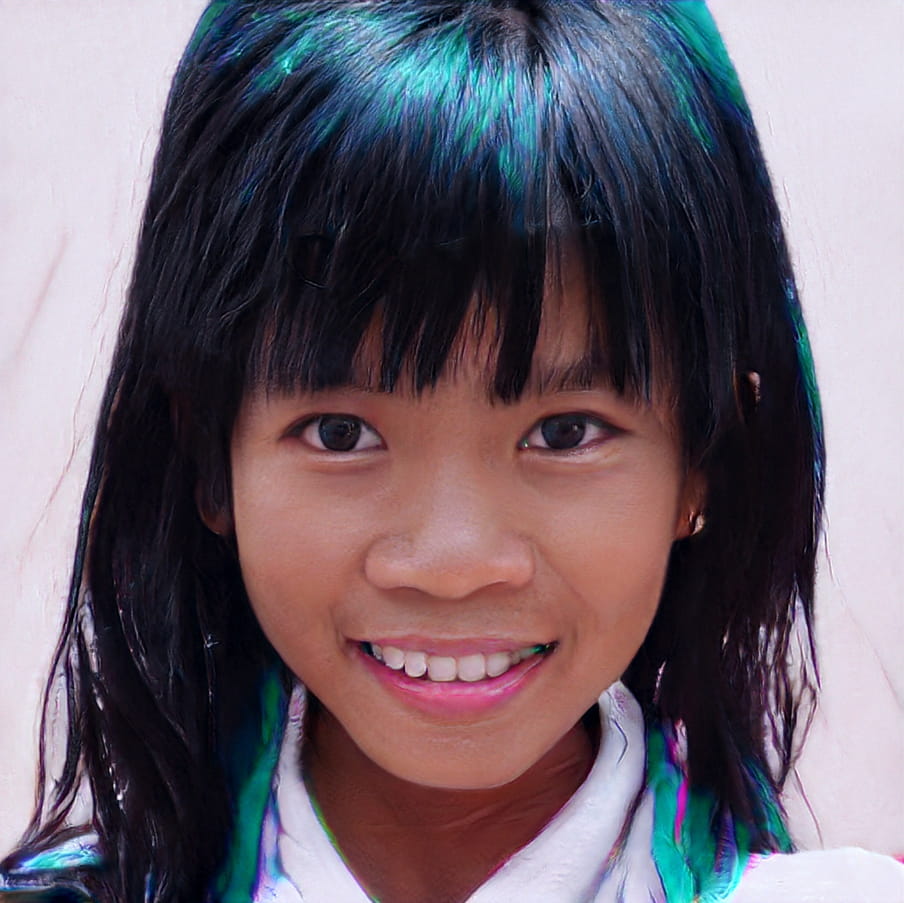
Examples of bias in AI are legion. A Microsoft chatbot on social media, which was meant to be a teenage girl, quickly learned to be a fascist misogynist. A programme for predicting recidivism targeted black men. And the majority of the people shown on This Person Does Not Exist, which is where the illustrations to this article come from, are white.
You can’t blame the models for this. They learn from the data they are fed, and so from us. The data you collect inevitably reflect the way social media are full of hate speech, or the fact that the police use ethnic profiling. The world is full of prejudice, so the models are too. Garbage in, garbage out.
To make matters worse, a model can become so complex that even its creator can no longer follow it. Which is a bit hard to swallow if a decision has a lasting impact on your life. Why can’t I get a loan? Yeah, well, it’s an algorithm. Why didn’t I get that job? Well, AI.
According to the latest European legislation, you have the right to ‘a human assessment’, with a person reviewing a decision and able to change it. But it is not as simple as that, since you don’t always even realise that a model (AI or otherwise) has made a decision about your life.
And, er…
Yes?
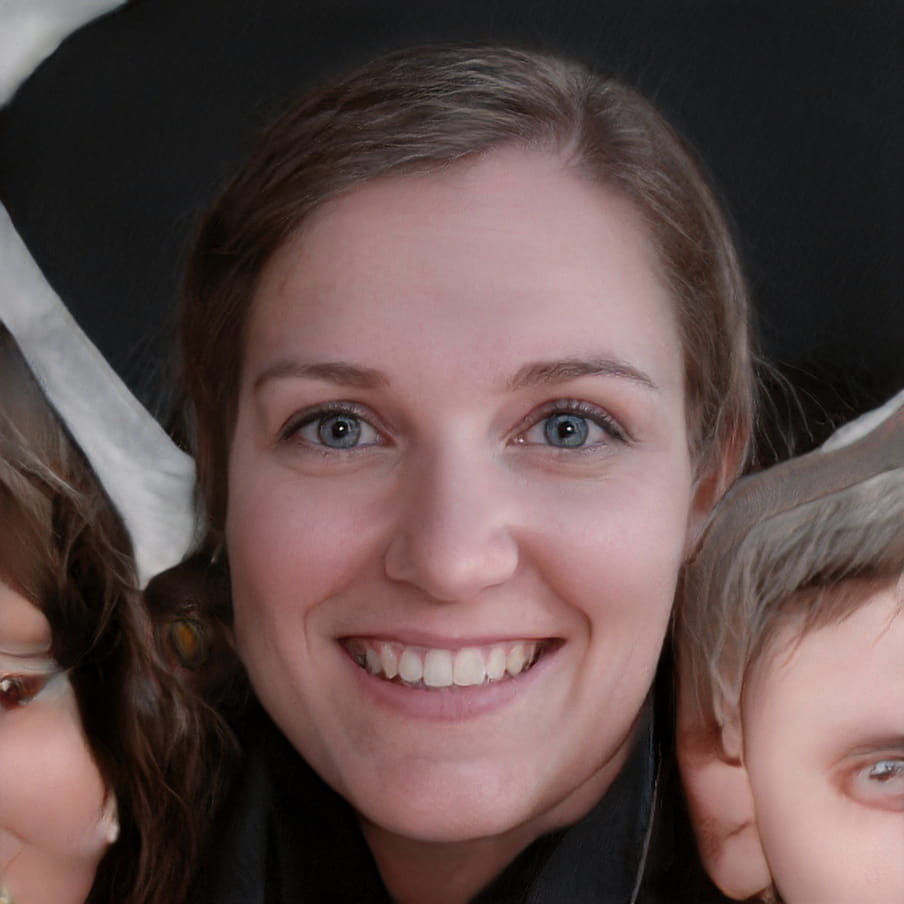
Are robots going to take over the world?
It depends who you ask. People like Stephen Hawking, Nick Bostrom and Elon Musk have said that we have reason to fear ‘superintelligence’, a form of intelligence that far exceeds that of human beings.
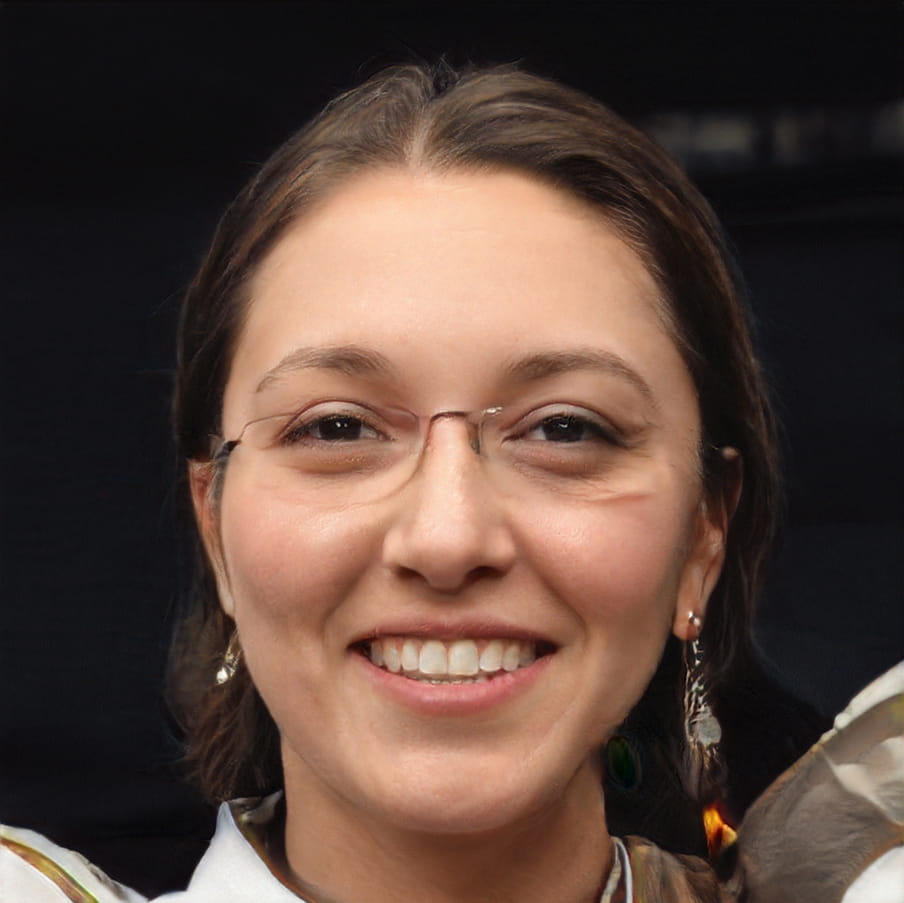
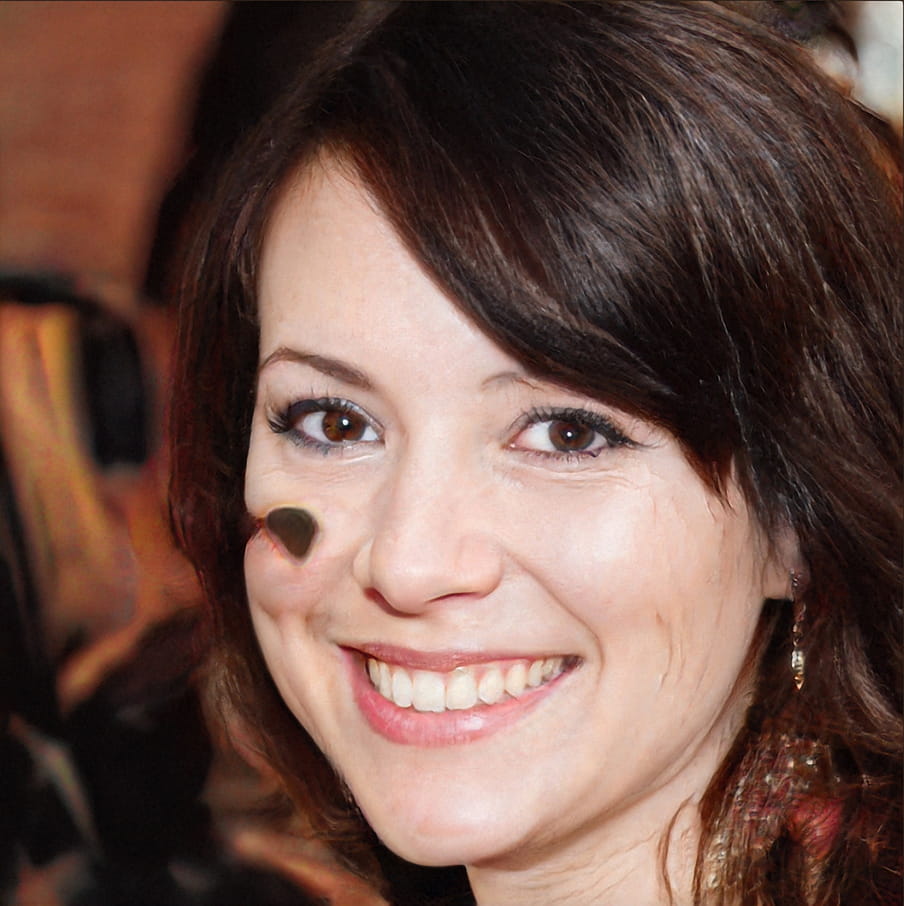
‘The first ultra-intelligent machine is the last invention that man need ever make,’ stated mathematician Irving Good back in 1965. Apple’s co-founder Steve Wozniak is not really worried, but he does think that at some point we will become robots’ pets. Not many AI experts think it will go very fast. “Worrying about murderous AI is like worrying about overpopulation on Mars”, said the outspoken AI researcher Andrew Ng.
Expectations get adjusted along the way as well, as they have been in the case of self-driving cars. Ford and other car manufacturers now admit they had overestimated how quickly autonomous vehicles would become the norm.
Extraordinary things have certainly been done with AI in recent years, but all the successful models had limited goals: winning a board game, recognising a face, translating a sentence. Go a bit further and it soon collapses. Just try asking Siri a surprise question.
Maybe superintelligence will arrive on the scene someday, and maybe it won’t. It’s hard to predict. But what is more worrying is that such science fiction fears are distracting us from the real problems with AI. Problems that are already here.
Such as?
Deepfake, for example: lifelike videos that are created using AI. Fake porn films were made with stars like Scarlett Johansson, Emma Watson and Gal Gadot. Such fake videos can also be used to tarnish the names of political opponents.
Below is an example of how you can put words into a person’s mouth, in this case Barack Obama.
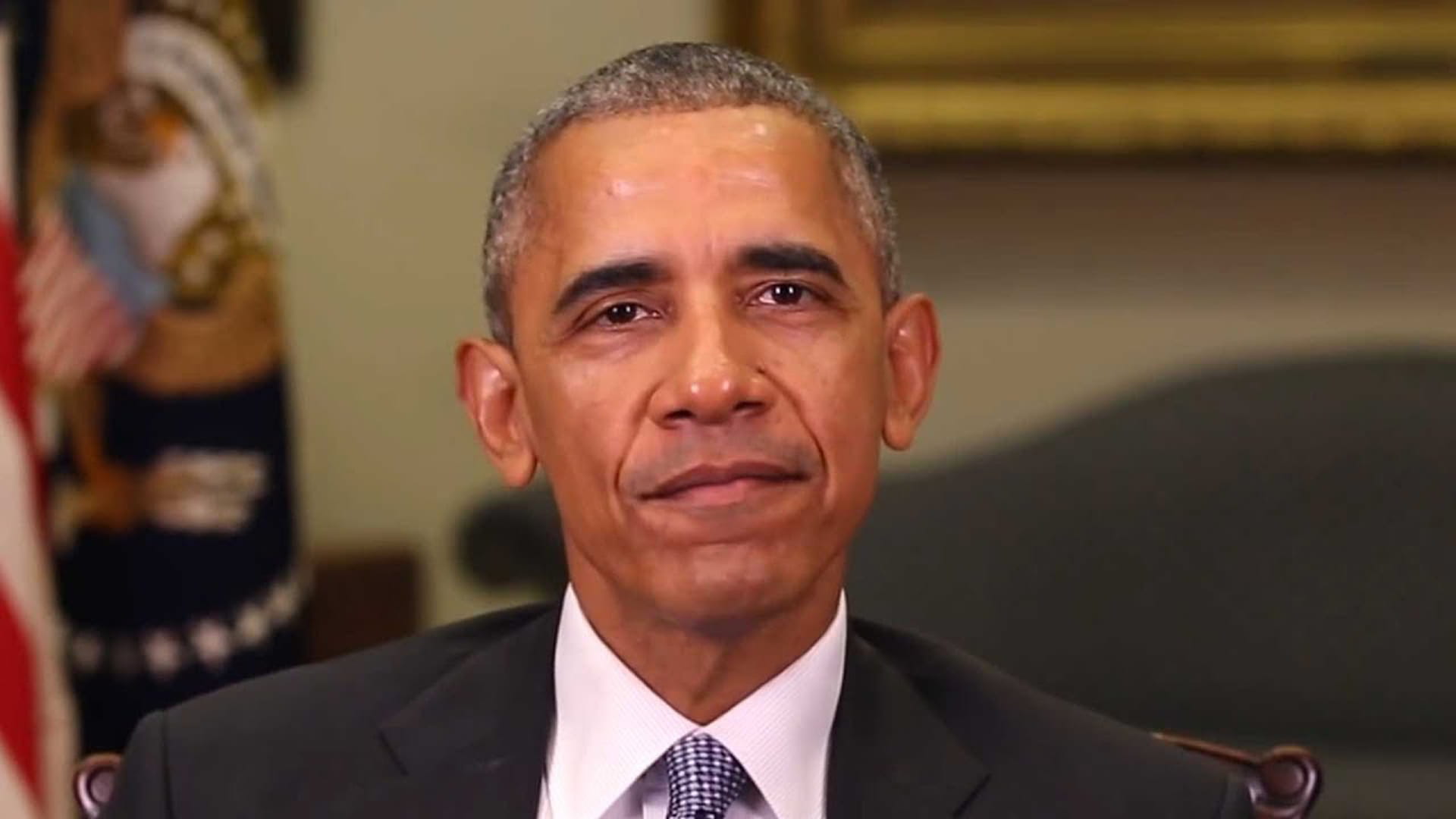
Another example of a problematic use of AI: China is building a surveillance state and one of the technologies it is using is facial recognition. Hotels, airports and even public toilets are already equipped with it. But the Chinese government’s ambitions go further: in 2020 it wants all the estimated 200 million cameras to be connected in a single network.
Facial recognition is in use closer to home, though, too. In London it is being used to track down offenders, although several experiments have shown that the technology missed the mark in 96 per cent of cases.
AI will be under human control for a long time yet. And humans will therefore be deciding what we do with it. The same algorithms that lie behind the deepfake videos can help to detect cancer. But the real progress no longer lies in better and better techniques, but in how we use them and the choices we make. The robots might be coming. But the humans are already here.
This article was translated from Dutch by Clare McGregor.
Thanks to everyone I have talked to about AI in the last few months. A special thank you to Daniel Worrall (Philips Lab, University of Amsterdam), who gave feedback on this article.
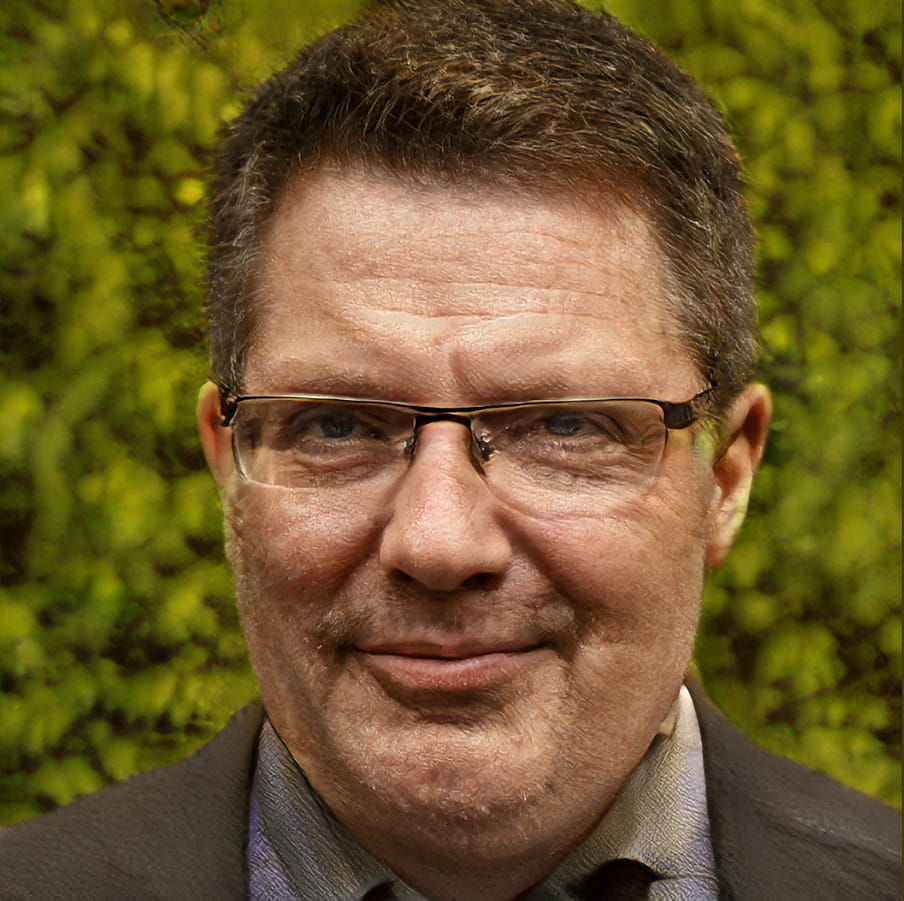
About the images:
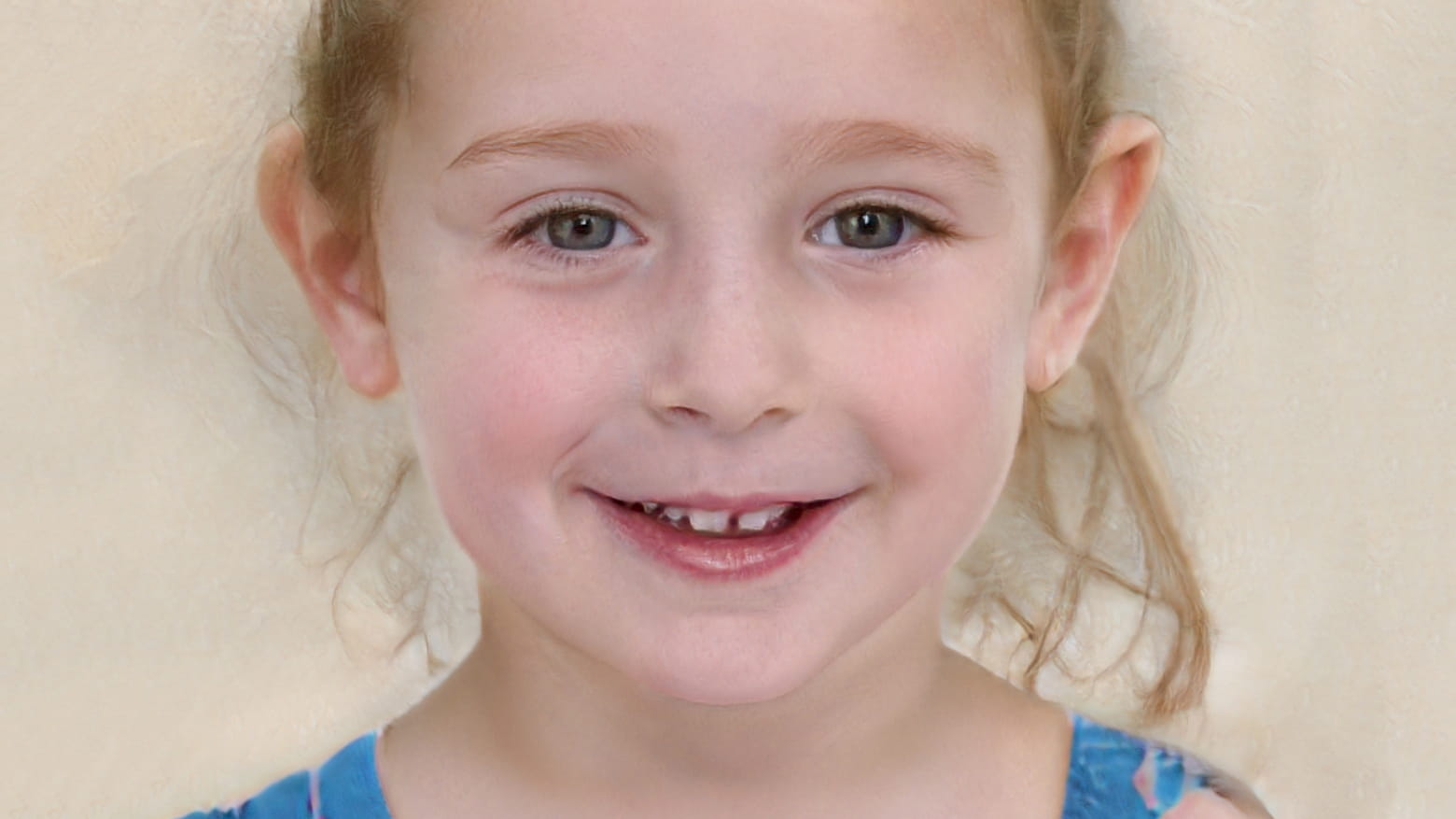
Stay up to date with Sanne:
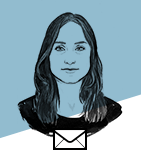