It’s easy to get cynical about artificial intelligence (AI). China is using facial recognition against the Uighurs. Google’s participating in the development of autonomous weapons. And facial recognition programmes are still struggling to recognise black faces.
But last year I also saw another side. At government organisations, start-ups and universities, I spoke to people working in the AI sector (although most of them prefer to talk about machine learning, because "AI" is a hype word that doesn’t appeal to them).
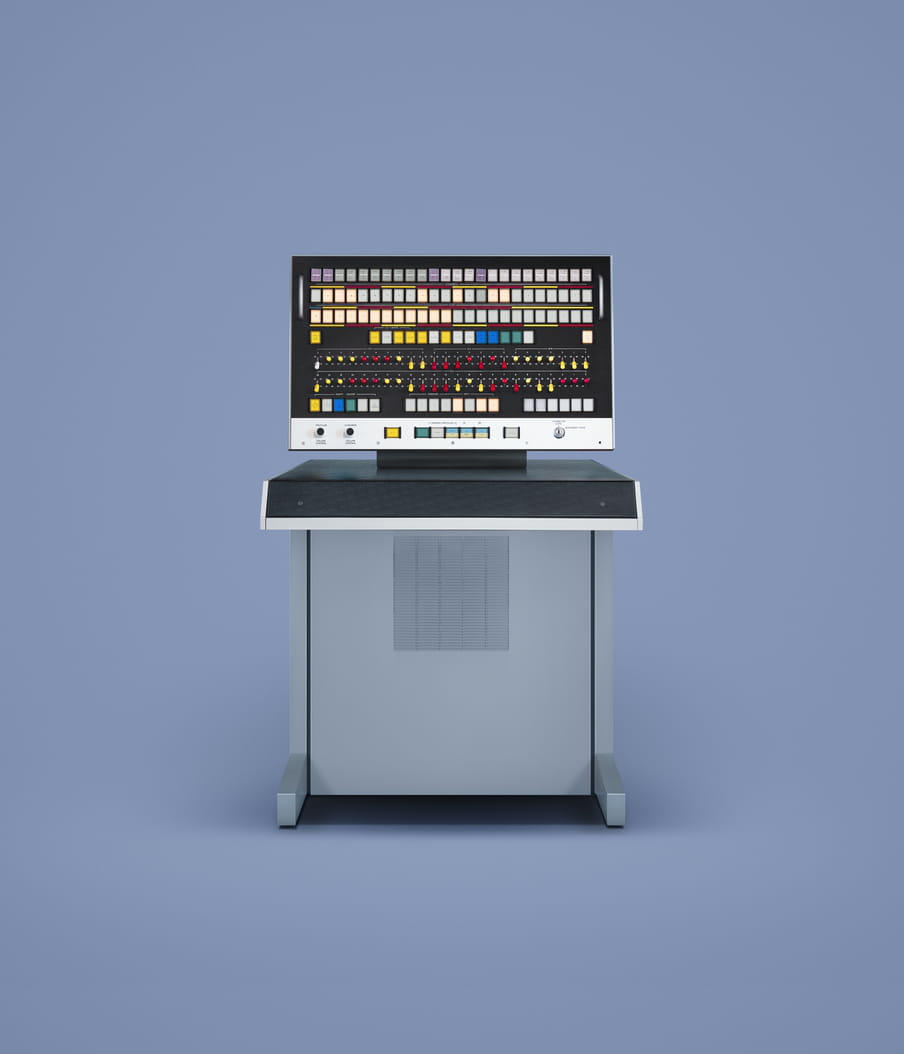
Each time, I learned something new and was sparked by the enthusiasm. But I also saw that many experts are just as concerned about the ethical consequences of these rapid developments. Yes, there is plenty to be cynical about, but also much to be hopeful about.
Another thing that gave me hope: the large number of people who wanted to talk to me. When I published a callout in October – on both The Correspondent and our Dutch-speaking sister site De Correspondent – 230 machine-learning experts let me know that they would like to tell me more about their work.
A few weeks ago I sent them – and my newsletter followers – an e-mail with the following question: What makes you hopeful about artificial intelligence? Today I’m sharing the best reactions I got. With the reasons why they also give me hope.
Time to talk about what is going well in the field of AI.
AI as a means, not an end
Because of the hype, AI sometimes seems to have become a goal in itself. Governments come up with AI strategies, companies stick the label "AI" on something as simple as an Excel spreadsheet and journalists are all too happy to write about a dystopian or utopian future in which AI has the power.
I often wonder: but what for? AI is just a tool. Fierce computational models can certainly do great things, but that’s it. AI is not the solution to all our problems. At most, it can help us out a bit.
Start with the problem, I think, and then look for a solution – technological or not. That’s why I became enthusiastic about examples where machine learning hasn’t been applied at random, but clearly meets a need.
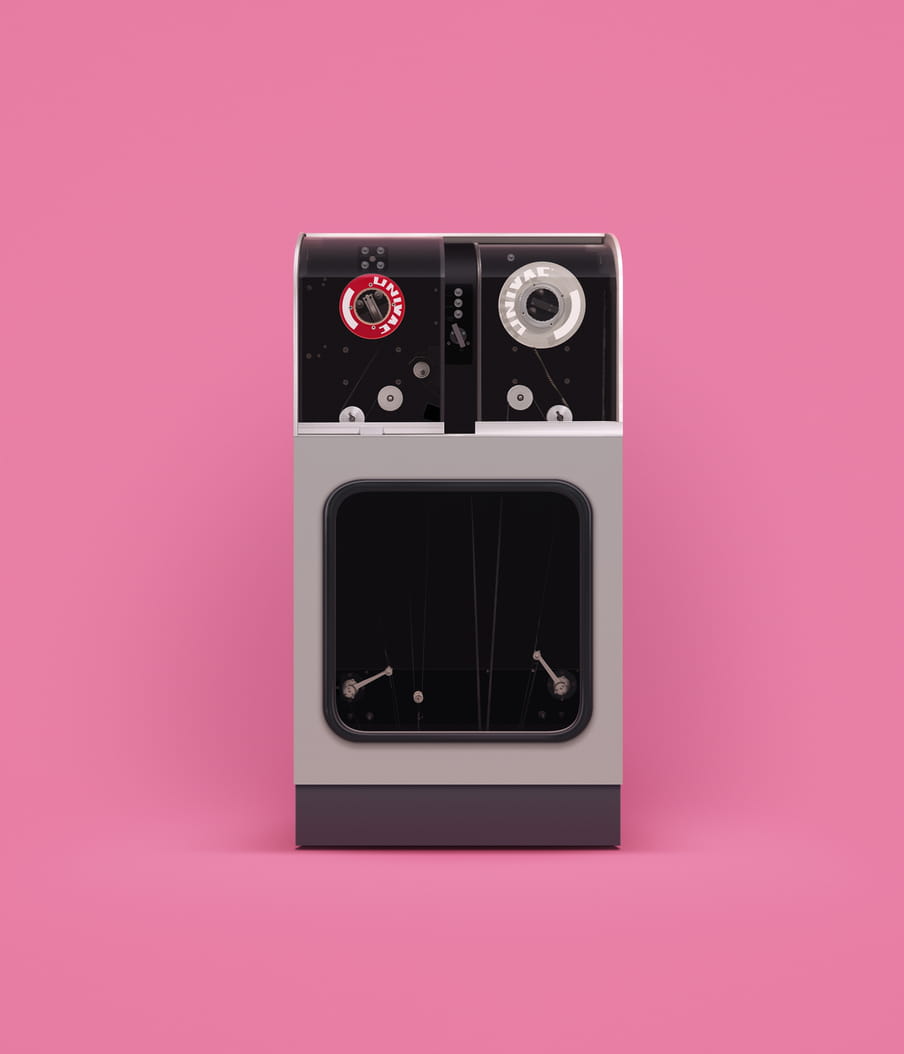
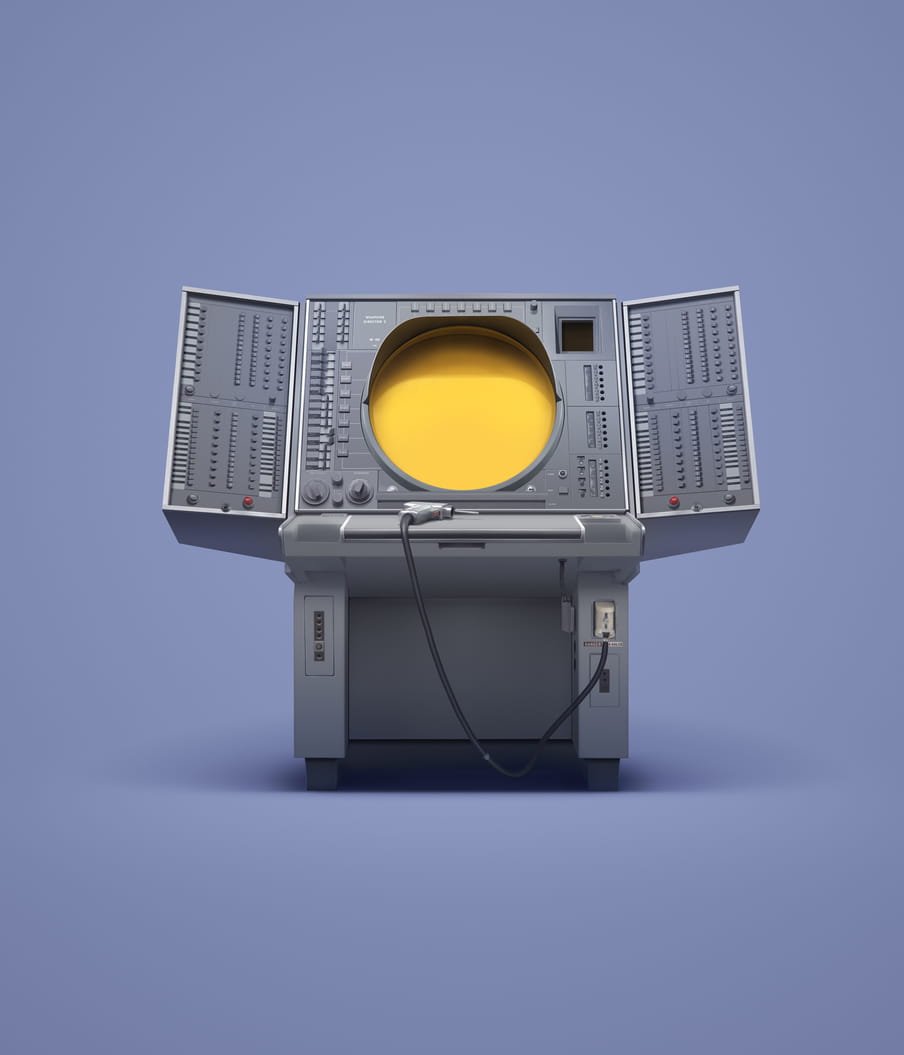
Annelot Vijn, information manager at the archives of the Dutch city of Utrecht, offered one such example. "HTR!" she emails. What? "Handwritten Text Recognition, or automatic transcription of old manuscripts." It used to be a painstaking task to copy this kind of text by hand, now it’s converted to Times New Roman in no time. That makes it searchable, which makes historical research a lot easier.
The Utrecht archives use the software package Transkribus to digitise handwritten texts, Vijn explains. It was developed with the support of the European Union (EU) and is intended to make archive documents accessible. The EU’s project coordinator, Günter Mühlberger, says that this is the first platform of its kind that can also be used by a non-technical crowd. At time of writing, some 27,000 users had registered.
One of them is Henri Brandenburg, who also emails me about Transkribus. I remember him from my home town Middelburg, where I often saw him transcribing documents from the Admiralty of Zeeland on Saturday mornings with a cup of coffee in the local bookstore.
Brandenburg explains how he trained Transcribus. First, you enter photos of the old text, after which the programme indicates with green boxes where the text is. You then transcribe those lines yourself, so that it’s clear which line belongs to which piece in the picture.
After you have done about 50 pages – still a monumental task – you can let the programme train itself. Brandenburg: "In that time Transkribus builds a ‘model’ that you can use on new pictures ... Depending on the consistency of the script of the original scribe and the transcription, you can achieve a word error rate of less than 2%!"
"The models are getting better and better," says Vijn, "and this makes (unreadable) treasures accessible."
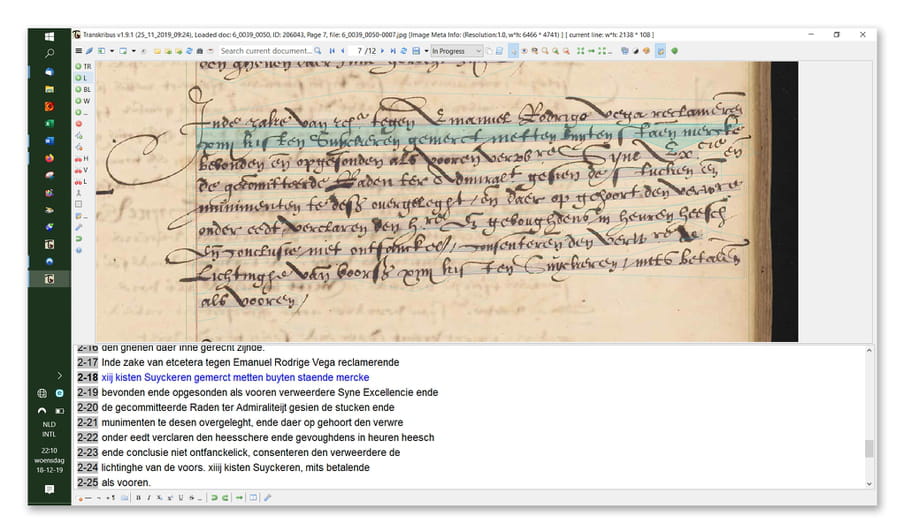
AI for now, not for the distant future
The AI world is full of great promise. Take Elon Musk – not exactly a man of small ideas – who said during a presentation of his company Neuralink this year that he wants to achieve "a sort of symbiosis between human and artificial intelligence".
The idea: wires with electrodes, thinner than human hair, are implanted in the brain to, for example, control a prosthesis or cure a neurological disease. According to Musk, Neuralink is already testing the technology on rats and wants to work with humans by the end of 2020.
Brain-machine interfaces (BMIs), as envisaged by Neuralink, give consultant Jos Gubbels hope. He says in his contribution that they can help to give people with severe disabilities hope of a full(er) life.
He shares a video by Thi Nguyen-Kim – "one of my favourite science journalists" – in which she explains what BMIs are and what is already possible. TL; DR : cool scientific breakthroughs, but still many issues. In short, it is still a long way off.
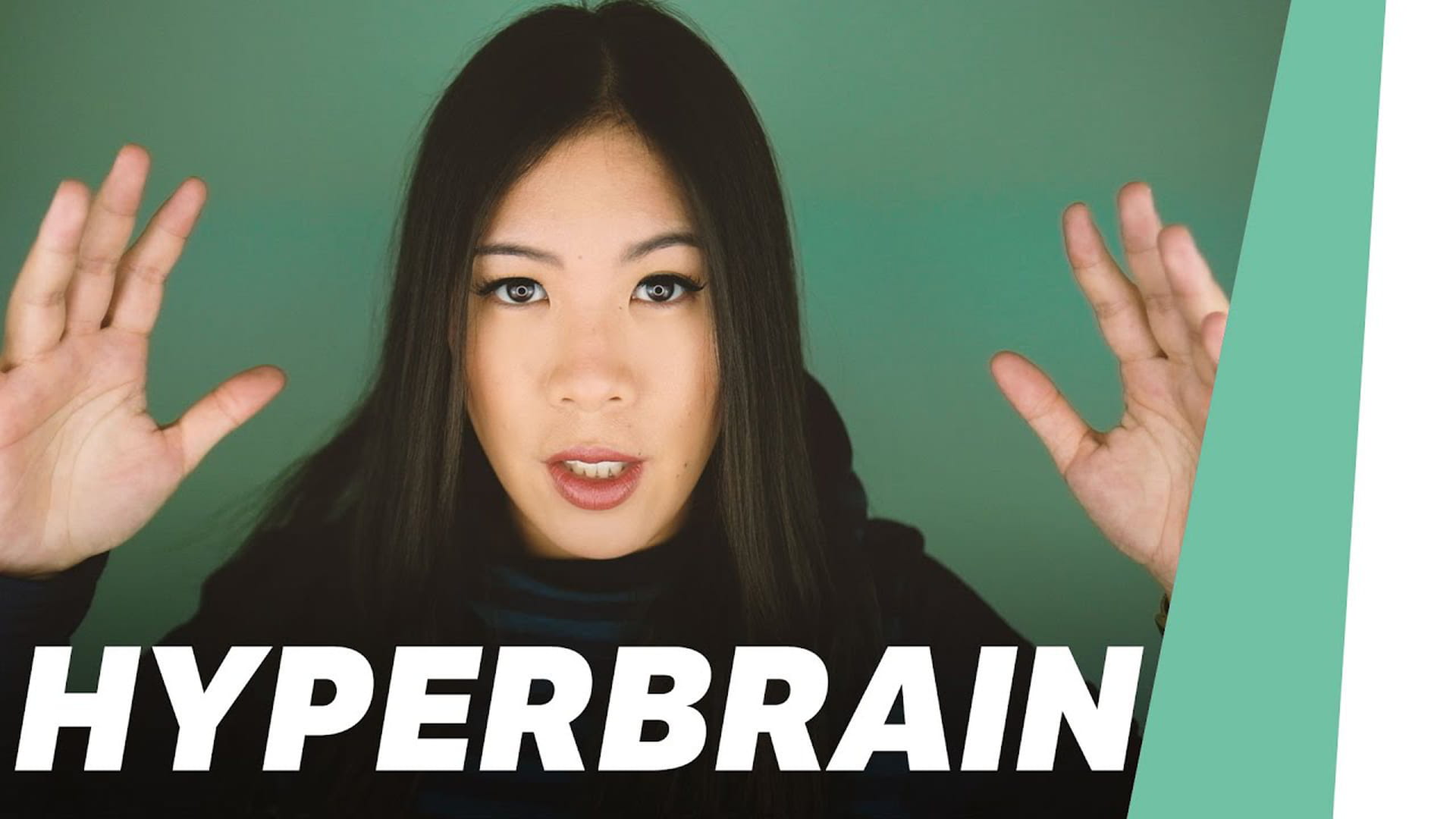
What makes me more hopeful are applications that can change lives right now. Lieke Scheewe – who advocates for the rights of people with disabilities – gives one such an example: Seeing AI, by Microsoft.
This app helps visually impaired people by, for example, reading text, describing objects and recognising banknotes if you want to pay with cash. All with the help of neural networks, the big hit in AI country.
Scheewe herself is in a wheelchair and finds technological developments for disabled people hopeful, but adds: "The disability remains, as does the need to ensure that society is organised in such a way that everyone can participate on an equal footing."
Framing is also important, she writes. Often it’s "‘look how this disabled person with her pathetic life can now do something she couldn’t do before, and see how she laughs now (or see those tears of happiness)!’ Behind that are all kinds of assumptions and prejudices."
By contrast, Microsoft presents in a beautiful and down-to-earth way what the app means to people, allowing them to decide for themselves how they use it in their lives. The tech company recently announced that the Seeing AI app is now available in five additional languages.
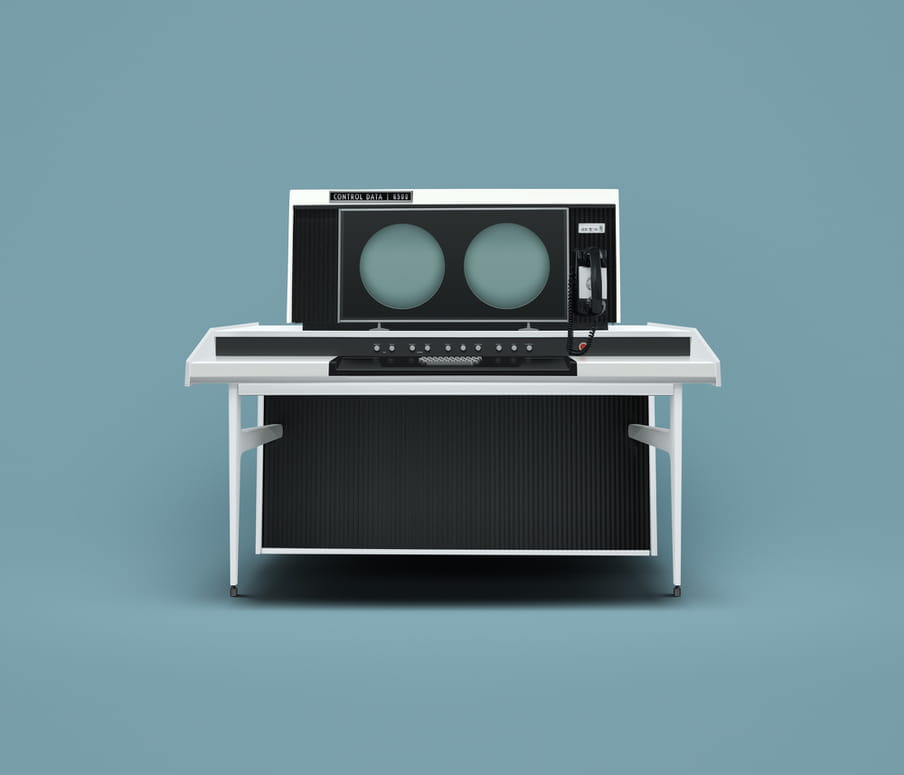
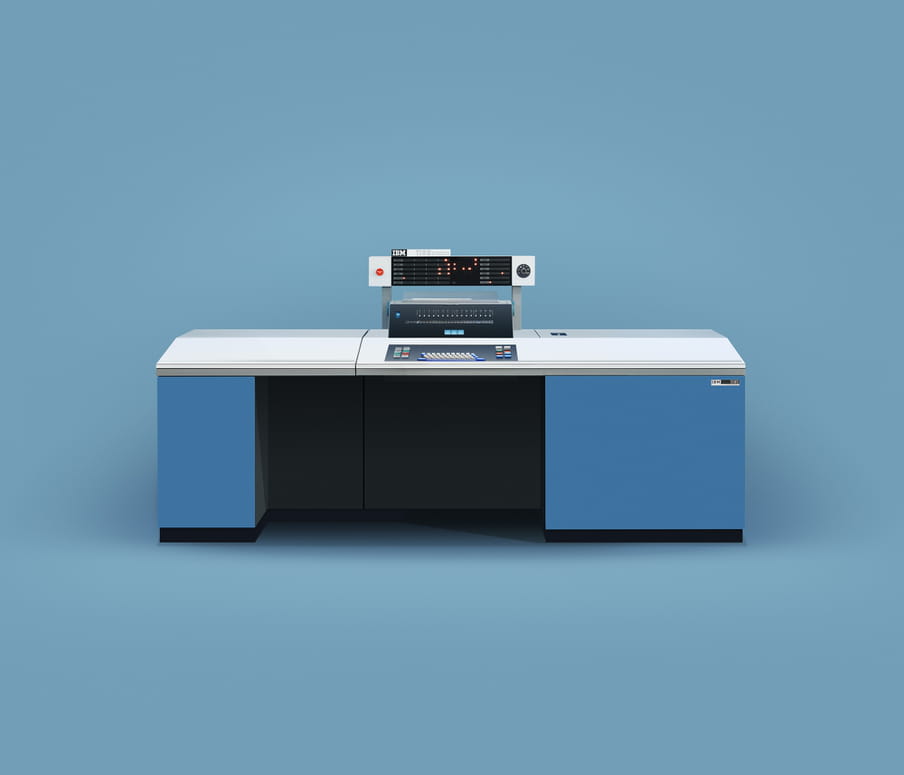
AI on behalf of experts, not charlatans
There’s a lot of bullshit being sold when it comes to AI. In his presentation "How to recognise AI snake oil", computer scientist Arvind Narayanan shows a screenshot of the promotional material of a human resources company that claims to be able to estimate whether someone will be a good employee based on a 30-second video: a certain DuShaun Thompson would be a "bottom line organiser" who "changes the status quo". He gets a score of 8.98 on the HR company’s 10-point scale.
"Common sense tells you this isn’t possible, and AI experts would agree," writes Narayanan. "This product is essentially an elaborate random number generator." Narayanan shows with his research that it is very difficult to predict ‘social outcomes’ with data – think of recidivism of a convicted person or the development of a child.
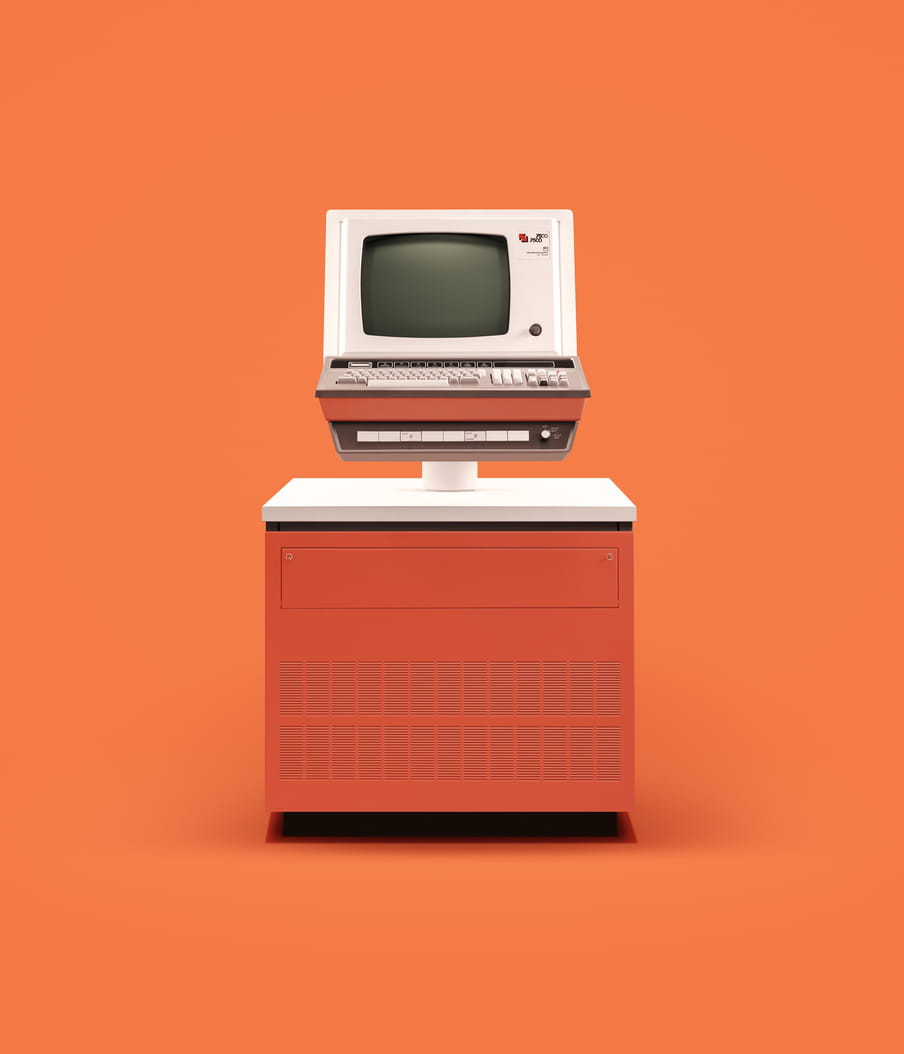
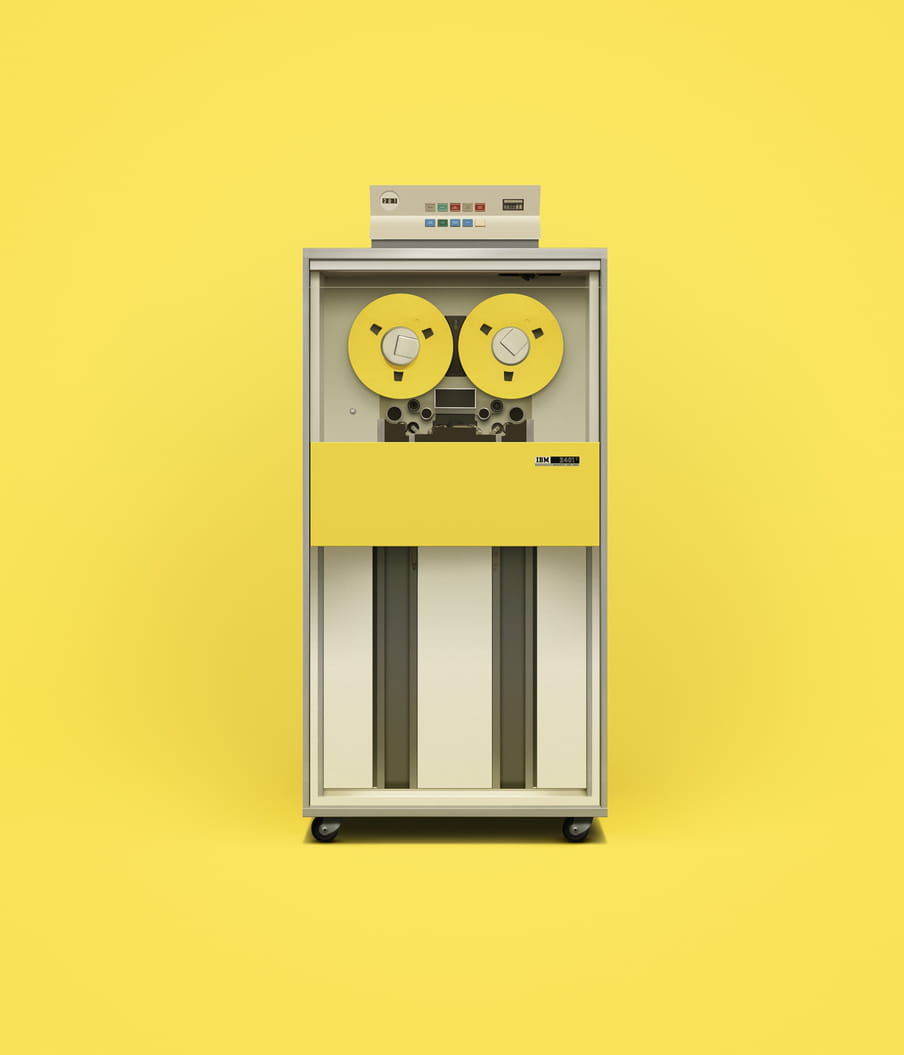
For hopeful developments, I prefer to look at people who really understand the technology, not just those who can deliver a good sales pitch. And in 2019, many renowned AI researchers focused on one specific theme: climate. An impressive list of scientists – including Google Brain founder Andrew Ng and Turing Award winner Yoshua Bengio – published a catalogue of ways in which machine learning can help combat climate change.
It’s not a panacea, they admit, but in some places it can help. For example, by improving climate models. One thing I learned from the paper is the greatest source of uncertainty in those models: clouds. Bright clouds block sunlight, while dark clouds keep the heat on the earth. Current physics models cost a lot of computational power, but research into neural networks shows that it is possible to achieve similar results for much less money.
Deforestation is another theme that comes up in the article. In his contribution, Leon Overweel, himself a "deep learning engineer" at a company called Plumerai, gives a concrete example of an organisation that uses data analysis to combat illegal logging: Rainforest Connection. The nonprofit hangs up old smartphones, connected to solar panels, in the rainforest to record noise. The technology recognises the sound of a chainsaw or a gunshot and signals park rangers.
Scientists are also looking at possibilities to use machine learning to deal with the consequences of climate change. Daniela Gawehns, a doctoral student in data sciences at Leiden University, e-mails me about a team at the Dutch Red Cross that is working on this. "510" estimates the damage after a natural disaster, for example after hurricane Irma on St Maarten. There they took pictures with drones and, with the help of volunteers, marked which houses were damaged. They are now working on machine-learning models so that they can work even faster and on a larger scale.
"They are using image recognition to do real good," writes Gawehns. "Like saving lives (and not to develop self-driving-cars … who needs those anyway?)"
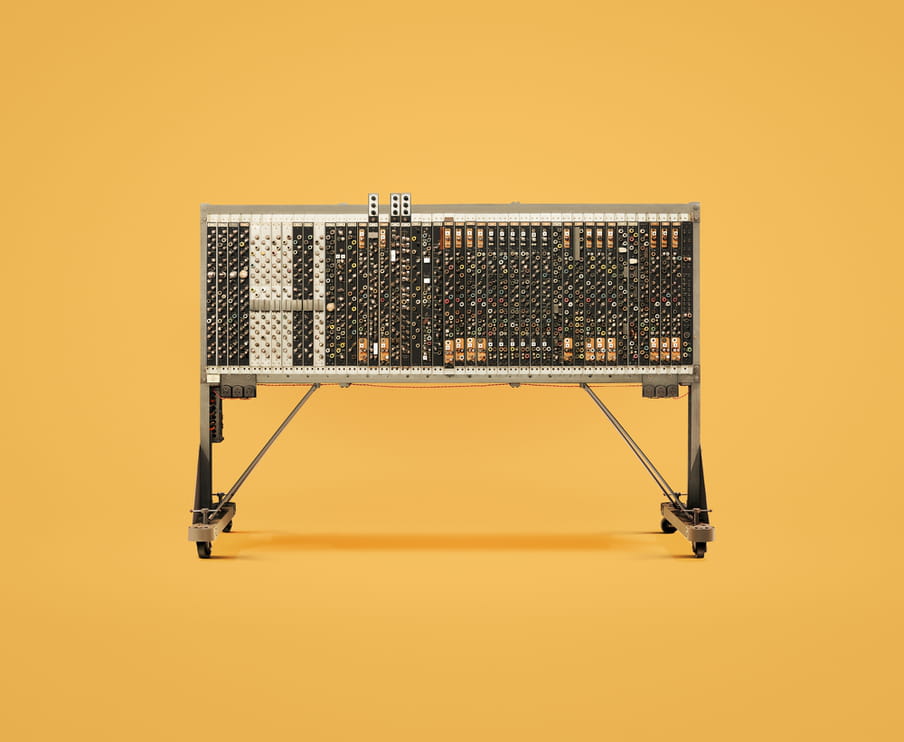
AI for good
"Something to be hopeful about is the mature, critical attitude of the people behind AI," says data scientist David van de Merwe. He mentions people such as Holger Hoos with the European research network Claire; Sennay Ghebreab of the Civic AI Lab; and the team at iCog Labs in Addis Ababa, Ethiopia. All are working on AI and ethics in their own way.
Van de Merwe is one of the many people who contacts me about a hopeful development, namely, the discussion about the shortcomings and the ethical issues which has gotten off to a good start in recent years. That conversation has not only been about making the AI systems themselves fairer, but has also prompted the question: do we want such technologies in the first place? In 2019, the answer has sometimes turned out to be "no".
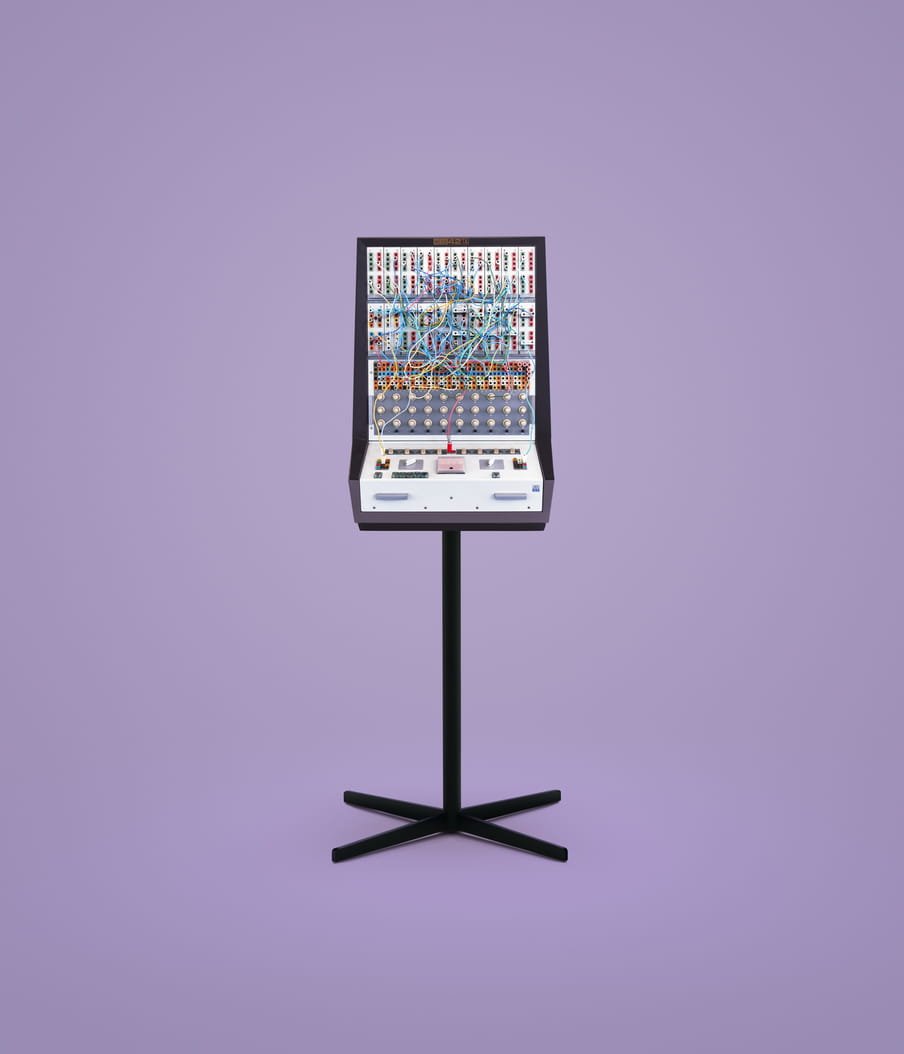
Late in 2019, the Australian tax authorities turned back Robodebt, a controversial system that automatically gave people an unjustified tax debt; Portland, Oregon and other American cities are planning to ban facial recognition software; and a court case is pending against the Dutch "system risk indication" (SyRI), in which all kinds of personal data is used to predict, for example, welfare fraud.
While these measures are reactive – cleaning things up after the shit’s hit the fan, there are voices calling for legislation so that bad AI ideas can be blocked before they inflict harm.
The European commission’s incoming president, Ursula von der Leyen, has promised to come up with an AI law. It’s expected to be published in February 2020. At the moment, technology companies are still too often expected to self-regulate, and government regulation can change that. But it remains to be seen which direction the commission will move towards.
The beautiful applications of AI, such as those in this article, deserve a chance. But that does not mean that everything with the label "AI" is a good idea, or that AI can solve every problem. The fact that there is a counter-movement that dares to ask critical questions gives me hope.
On to a critical 2020.
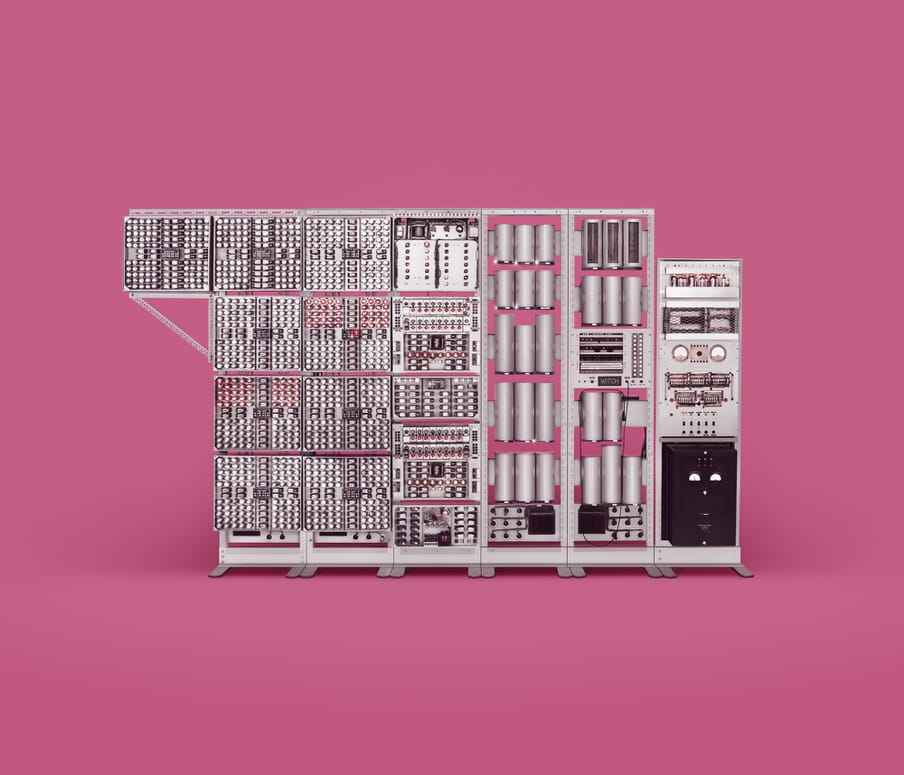
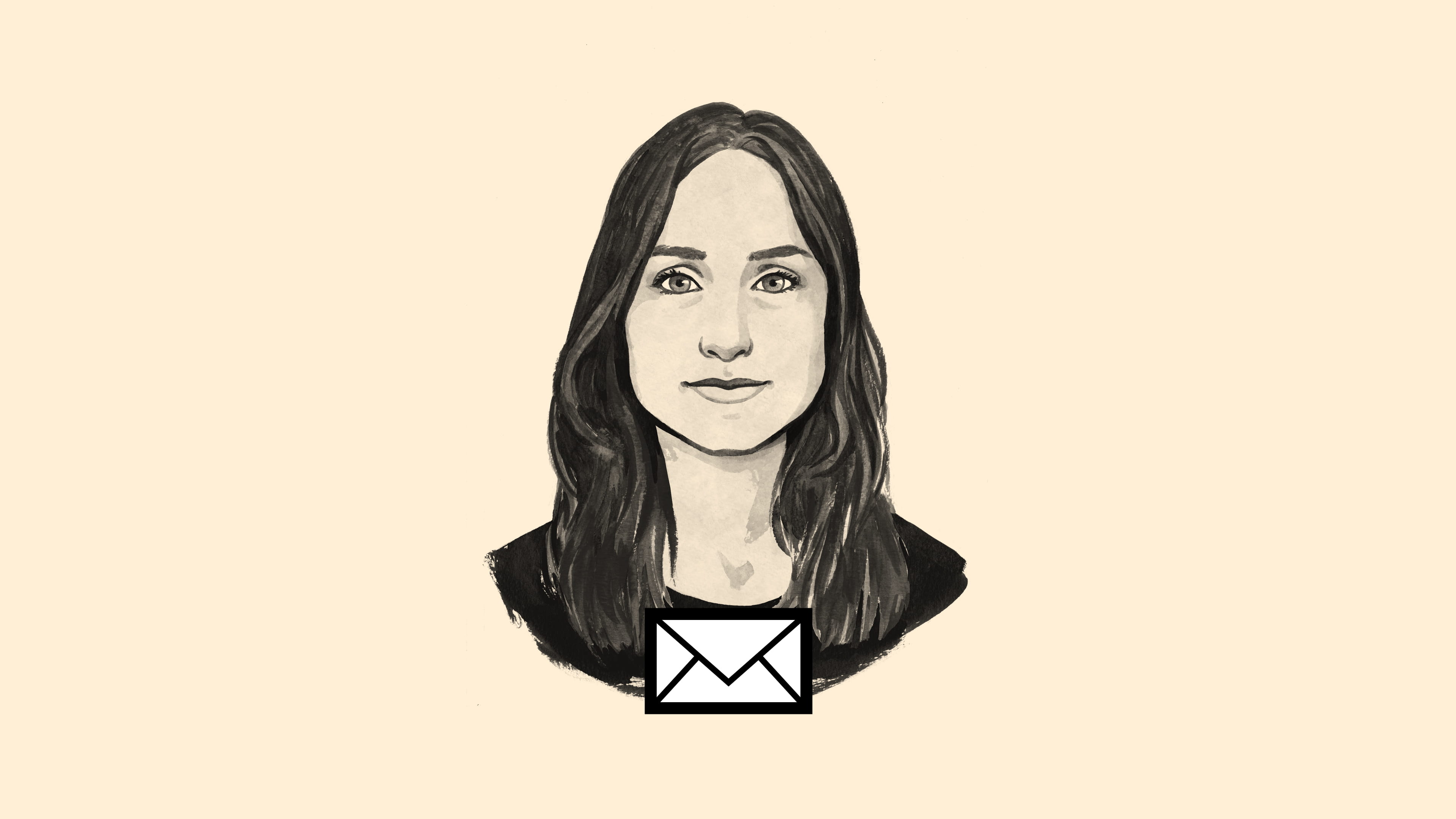