I have to tell you how the debunking of an important theory about migration was itself debunked. You probably had to read that sentence twice, and I get that. It’s been a major mindfuck.
But one I learned a lot from. About how science works, and how we as journalists contend with that. About what expertise actually is, and why it is so limited. And about certainty, doubt and being right.
So buckle in and brace yourself for a story about that time I said I was wrong – and turned out to be mistaken.
How it all started: the migration hump
It all started a few months ago when I read a new study about the migration hump. I was immediately interested, since “the hump” is a well-known, very influential theory about the relationship between migration and development.
Basically, the theory states that as poor countries become richer, outward migration increases rather than decreases. This may seem counterintuitive: we might expect that when countries get richer, reasons to leave will diminish because life there is better now, right? But the migration hump shows that this is only the case above a certain income level, starting from about $7,000 to $10,000 per person per year.
Many poor countries are a long way away from that, which means that economic development in those countries will lead to more migration, not less. That’s because migration costs money, and when people who were previously very poor have some, they are more likely to leave.
Come up with a graph comparing income and emigration, and you’ll see a more or less hill-shaped curve showing the lowest rate of emigration in poor countries, the highest rates in middle-income countries, and falling rates for rich countries: the migration hump.
I frequently reference the migration hump in my articles, especially to criticise European migration policy. And there’s a reason for that: the European Union (EU) is spending more and more money on development aid to reduce migration. But the migration hump shows that this policy is based on a misconception: if more aid leads to more development in poor countries, that funding will cause net migration to increase, not decrease.
And then that new study came across my desk, released under the MEDAM research project. The researchers were quite blunt: their analysis of migration data showed that the migration hump was an oversimplification. In actual fact, their models produced opposite results. They calculated that when a poor country becomes richer, emigration to rich countries goes down.
Their explanation was that their method was different: instead of comparing emigration in poor and rich countries, they compared countries with themselves, over time. Why? Because a comparison between poor and rich countries overlooks the differences between those countries: differences that can affect income as well as migration.
I had colleagues and migration experts with more knowledge of econometrics take a look at the new paper; I spoke to the researchers, and then decided to write an update.
The research looked convincing, and I wanted to hold myself accountable because a theory I had often cited in my pieces did not seem to hold up.
I thought that was the end of my hump saga.
But then I was tagged in a Twitter thread by Michael Clemens, a leading development economist at the Center for Global Development. The new research, he tweeted, was based on a statistical error.
Clemens and his calculations
There was nothing wrong with my article as such, Clemens told me in a private message. “The problem is with the research itself.”
All very friendly, of course. But I wasn’t so sure. Could I have seen this coming? Should I have done something differently? What could I learn from this?
I took another in-depth look at the paper, and delved into Clemens’s criticism. I looked at his charts, tables, formulas.
The only slight problem was I didn’t understand any of it.
That wasn’t really all that strange because Clemens’s criticism targets researchers’ statistical methods. If you don’t have a degree in econometrics, the analysis is almost impossible to follow. In fact, it’s almost impossible for people who have studied advanced statistics. My colleague Sanne Blauw – PhD in econometrics – called me after spending three hours analysing both papers: “I think I more or less understand Clemens’s criticism.”
I asked more experts for assistance: professors and PhD students who could explain the statistics to me, who had experience with time series and cross-sectional panel data, who knew more about spurious regressions and non-stationary variables. I had long phone calls with Michael Clemens and Claas Schneiderheinze, one of the researchers who authored the original MEDAM paper.
I can’t say I’ve completely mastered the maths. But here’s what I now understand of the discussion.
Would you like to skip the detailed explanation of the criticism and jump straight to what I learned from this experience as a journalist? Click here.
How can both of these things be true?
The reason Michael Clemens took a deep dive into the statistics of this paper was simple: the researchers’ findings diverge dramatically from his own observations in the real world. That raised his suspicions.
In principle, Clemens says, it is a good idea to look at changes in income and migration for individual countries, rather than comparing countries to each other. That approach does in fact, as the researchers state, prevent us from incorrectly assuming causal connections that are actually due to other fundamental differences between countries (such as geographic location or political climate).
The model shows a strong negative correlation between income and emigration
But, Clemens asserts, if you look at those changes per country, you see the exact opposite. The paper finds a strong negative correlation between income and emigration: if a country’s per capita income doubles, emigration is halved. That is a massive effect – and one not reflected at all in countries that have actually seen their per capita income double in recent years. Take a look at the graphs Clemens generated to show the correlation between income and migration in all these countries:
None of the countries in these graphs experienced a long-term drop in emigration when their GDP per capita rose. The data from these countries shows what the migration hump predicts: if the income of a poor country goes up, emigration also rises. The MEDAM paper would only be correct, Clemens explains, if the poor countries of today were diametrically different from the poor countries of a few decades ago. Could that be the case?
Possibly.
But if you look at the poor countries of today, you can see that the course of their development over the past 50 years has also been very different than the MEDAM researchers would expect. In the graph below, Clemens shows the correlation between income and migration from all developing countries to rich countries, from 1970 to 2019. Brace yourself, it’s a bit messy.
Each arrow represents a single country, pointing from emigration in 1970 to emigration in 2019. Although the graph is almost impossible to read, we can see one thing clearly: the arrows for almost all countries tilt upwards as income rises. In other words, based on this graph, which also includes today’s poor countries, you should still believe that the migration hump exists.
So how can the MEDAM researchers’ model produce such different results? That was the question Clemens was trying to answer when he dug deeper into their statistical model.
Too many details? Click here to jump to my conclusion!
Is this a good dataset?
And there, Clemens found two things that he views as problematic: the statistics and the data.
Let’s start with the data, since that’s the easiest part to understand.
The researchers use data from the OECD. They looked at how many people of different nationalities received a residence permit in each OECD country each year. These numbers were then added up by country of origin. This, of course, does not include all migration: people who do not migrate to OECD countries and people who never report to the authorities are not found in this dataset.
But more fundamentally, says Clemens, people who receive a residence permit have often been living in an OECD country for years, if not decades. In the United States, for example, half of the people who get a green card have already been in the US for a long time. If you compare the increase in Mexico’s income in any given year to the increased number of Mexicans in the US in that year according to this dataset, your results are distorted.
In actual fact, the number of Mexicans arriving in the US has been decreasing for years, but this dataset makes it look like it’s actually increasing – because Mexicans who have been living in the US for many years are finally getting a green card.
It would be better, Clemens believes, to look at the dataset provided by the UN and the World Bank, which counts what’s known as the international migrant stock: the number of people from a country who are currently living abroad. If there is an increase in the international migrant stock as a percentage of the population, then you know that there has been an increase in migration flows, not in residence permits.
Click here to jump to my conclusion!
Are the statistics accurate?
So here’s where it gets difficult: the statistics. The MEDAM researchers take two non-stationary variables on one side of their regression that may potentially be co-integrated, thus removing the long-term trend of both variables, thereby only measuring the effects of economic shocks.
Everyone got that?
All right, let’s try that in layman’s terms.
In econometrics, there are series of variables that are stationary and non-stationary. That means that they either do or do not exhibit a trend over time. For example, a stationary variable could be the location of a country. That never changes. A non-stationary variable could be income. That changes over time.
If you want to know whether two non-stationary variables are affecting each other, you can’t find out simply by plotting them on a graph. Even if they aren’t directly related, chances are there will be some sort of correlation between the two. An example: as a city grows larger, more storks are seen in the city (non-stationary variable 1) and more children are born (non-stationary variable 2). If you plot those two variables on a graph, the result is statistically significant nonsense: more storks and more children follow the same time trend – so clearly storks must be delivering babies.
The normal econometric trick to solve this problem is to remove the time trend. By doing that, you look at how the variables change compared to the trend. So for example: you look at periods when the number of storks is increasing faster than the expected trend, and in that same period you look at whether the number of children is also growing faster than average. If this is not the case, you can show that there is no causal connection – and that babies aren’t delivered to your doorstep by a large bird.
It’s a neat trick, but the disadvantage is that your results only tell us about short-term deviations; the long-term trend disappears from your analysis.
What this paper doesn’t describe, according to Clemens, is the correlation between migration and economic development
Back to migration: according to Clemens, this is approximately what happened in the MEDAM paper. I’m saying approximately here, because there is one more complicated step missing: how this error could have crept into the paper indirectly.
Ready? Let’s dig in. The researchers don’t eliminate the long-term income trend from their analysis, but they do correct for population growth. Since the long-term trend of population growth runs parallel to income growth, the long-term trend of income also disappears from their analysis. If you correct for one variable, then you also correct for all the variables that are closely related to it.
This probably isn’t entirely clear to you – and I don’t fully understand it either. A clear case of taking the professors of econometrics’ word that this is how statistics work. Based on this, Clemens concludes that the MEDAM researchers only compare income shocks to emigration to rich countries. They essentially ignore the long-term trend of income growth.
Their conclusions, he emphasises, are still very interesting: the paper teaches us about the correlation between economic shocks (an oil boom, for example, or a currency crisis) and emigration. If incomes suddenly drop much lower than the normal trend, which recently happened in Venezuela, we can expect emigration to rise more rapidly.
But what this paper doesn’t describe, according to Clemens, is the correlation between migration and economic development. That’s because the long-term trend in income, which he says has been removed from this analysis, is in fact economic development.
That’s not just semantics, Clemens stresses: “There is no meaningful definition of ‘economic development’ that does not centre on incomes rising sustainably over time. For GDP per capita to be above the long-term trend for a few years is not development. Development is the unfolding of a prosperous economy; that is totally unrelated to short-term shocks.”
Of course there is plenty to debate about the definition of economic development. But I have to agree with Clemens here: it’s really not possible to come up with a good definition that doesn’t include a country’s long-term income growth.
Click here to jump to my conclusion!
How the MEDAM researchers responded to Clemens’s criticism
But we’re not done yet. Because the debate continues in an extensive discussion with the MEDAM researchers. Their response: wait a minute there, Clemens; we’re happy to take a look at your critical comments, but don’t toss out our entire analysis so quickly.
The researchers are still actively working to check and double-check their analyses against Clemens’s critique and will definitely be publishing more on that. And while I feel that Clemens makes some persuasive points (a feeling corroborated by the input and explanation from other econometrists I interviewed), talking to the researchers gave me several important nuances to Clemens’s criticism.
First of all, Clemens’s interpretation of the MEDAM results is somewhat exaggerated. For example, he tweeted that the researchers claim that when a country’s per capita income doubles, emigration is halved. But the researchers don’t make any such solid predictions in their work. Rather, they nuance their findings, by saying that actual migration figures are a factor of much more than just income: changing migration policies, conflicts, improved infrastructure – all these factors influence real-world migration.
Then there is Clemens’s criticism that the researchers only measure economic shocks. The researchers themselves are not convinced of this. Right now, they are using artificial data to get a better idea of possible errors in their model. Meanwhile, they have already checked one important thing: the solution that Clemens suggests in his paper. They say that applying that solution still gives them the same results. For econometric novices this becomes a he-said-she-said story. And since the MEDAM data is not public, even econometric experts would have a hard time checking who’s right here.
The heart of the problem seems to be this: exactly what question are the researchers answering? The MEDAM researchers say that they’re interested in the short to medium-term effects of income growth on migration. That’s because that is how policymakers currently view that correlation: can we use development aid to create jobs in poor countries and thus prevent people from migrating in the next five years or so? And no, they admit, that approach doesn’t measure development. But it is the question that is important for migration policy right now.
And that also brings us to the choice of dataset. The data that Clemens uses covers longer periods of time: the data points are 10 years apart. That longer interval is less useful for investigating this question, since the effects of income growth on an annual basis is what the researchers are interested in.
Considering all this, I have to conclude that the MEDAM researchers have misframed their paper: this study does not contradict the hill-shaped curve of the long-term correlation between economic development and migration. In other words, it doesn’t debunk the migration hump. But the paper does show that the same correlation does not hold true for the short-term plans that European governments are devising to combat migration.
The analyses done by Clemens and MEDAM differ in many respects: data, duration, regression model. But the most important thing here is: they start out with a different question.
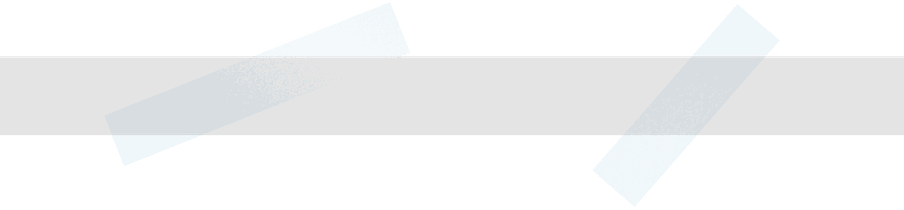
What I learned from this
Whether or not this paper is based on a statistical error (this discussion will probably be settled in academic journals in the next few months), all this commotion makes me wonder about my relationship with science as a journalist: what it is – or what it should be.
Every single person – including a journalist – has a limited framework that shapes their ability to understand something. I went to university, but I never took advanced statistics. Nor do I understand topics like the nitrogen cycle, Japanese grammar or the mathematics behind climate models. There is simply so much more that we don’t know than what we do.
Sometimes that doesn’t matter. I don’t have to understand Newton to say something meaningful about poverty alleviation. But often it does matter, even if we don’t realise it. As journalists, when our own knowledge and skills fall short, we rely on experts to fill in the gaps. But even for those experts, what they don’t know extends far beyond what they do know.
The mathematical calculations behind the models are so far removed from reality that results roll out like a rabbit out of a top hat
Especially when it comes to statistics. Many biologists, medical professionals, psychologists, economists or social scientists hire specialised colleagues to run their statistical analyses. And those specialists design models that are so complicated that only a handful of people can really understand them, or provide critical commentary. The mathematical calculations behind the models are so far removed from reality that results roll out like a rabbit out of a top hat: we have no idea how it works, but the outcome is self-evident.
Who knows how the statistical stage magic actually works? We can draw an obvious parallel to the epidemiological models being used to predict the course of the coronavirus pandemic: who has any idea exactly how those models work?
And that’s how a journalist – or policymaker – can end up in a tricky situation when two experts are making contradictory claims. Can you place two non-stationary variables on one side of a panel data regression without losing the long-term trend? Yes you can; no you can’t! How on earth can a journalist possibly figure out who is right?
The only solution seems to be cumulative knowledge: asking all the smart people you can find to give it their best shot too. At its very best, that’s how science should work.
And when that happens, it often turns out not to be about what’s true or false. Instead, it’s about which question we want to answer. The MEDAM paper answers an interesting question – just not the question of whether or not the migration hump holds true. And maybe the researchers subconsciously fell into a pitfall that science has created for itself: contentious studies that debunk something major are considered more prestigious than studies that confirm the prevailing assumptions. Just think about it: this was a study that I (a journalist) decided to focus attention on. I probably wouldn’t have taken such a close look if their model had once again supported the famous migration hump.
This discussion shows that the best thing we can do is to keep being critical: constantly doubting, questioning and admitting that what we know – and what experts know – is limited. Had I dug deeper I might have been able to raise some questions about the dataset used in the MEDAM paper. But then again: there is no such thing as an unproblematic dataset when it involves something as complicated as migration figures.
And the concept that two non-stationary variables cannot be regressed if you are controlling for a co-integrated third variable – that’s not a question I could even have imagined asking in the context of this paper. And neither have many, many scientists, because the MEDAM paper has been read and widely acclaimed by lots of other smart people.
Actually, I’ve started thinking that journalists, scientists and policymakers are all in the same boat here: we would love for the world to be simpler than it can be. We want to be able to capture it in a nice, neat model, and then wrap it all up in a nice, neat article. But reality is so much more capricious and complex than any model can capture.
Seeing more shades of grey is also a way to understand the world better – but it’s not quite as simple to put into a pithy headline.
It’s easier to just say: I was right after all.
Many thanks to Monique de Haan, economics professor at the University of Oslo; Bas van der Klaauw, economics professor at Vrije Universiteit Amsterdam (VU); Quint Wiersma, PhD researcher studying economics at the VU; Benjamin Wache, PhD researcher studying economics at the VU; and Maarten Lindeboom, economics professor at the VU, for reviewing the papers and patiently explaining the underlying econometric principles.
This article first appeared on De Correspondent. It was translated from Dutch by Joy Phillips.
Dig deeper
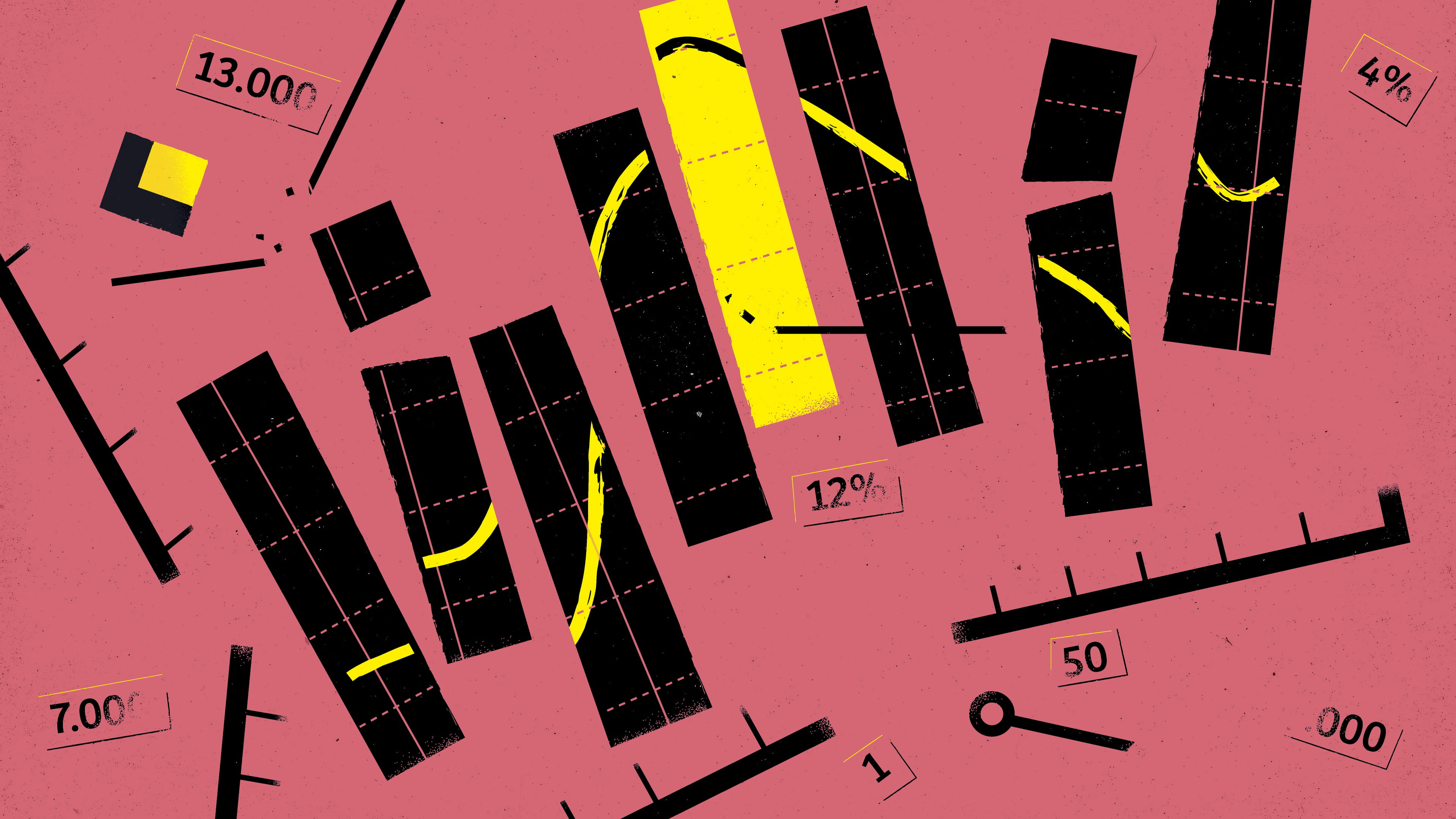