Timnit Gebru was wary of being labelled an activist. As a young, black female computer scientist, Gebru – who was born and raised in Addis Ababa, Ethiopia, but now lives in the US – says she’d always been vocal about the lack of women and minorities in the datasets used to train algorithms. She calls them “the undersampled majority”, quoting another rising star of the artificial intelligence (AI) world, Joy Buolamwini. But Gebru didn’t want her advocacy to affect how she was perceived in her field. “I wanted to be known primarily as a tech researcher. I was very resistant to being pigeonholed as a black woman, doing black woman-y things.”
That all changed in 2016 when, she says, two things happened. She went to Nips, the biggest machine learning conference, held that year in Barcelona and counted herself among just four other black people at an event attended by an estimated 5,000. That same year, Gebru read a ProPublica story on the use of algorithms in Broward County, Florida, to predict the likelihood that someone arrested would reoffend. “The formula was particularly likely to falsely flag black defendants as future criminals, wrongly labelling them this way at almost twice the rate as white defendants,” the journalists wrote. That investigation became a touchstone example of algorithmic bias.
The ProPublica piece alerted Gebru to a reality she had not yet considered, and to the bias present in her own research. “That was the first time I even knew that these algorithms were being used in high-stakes scenarios,” Gebru admits. “I was absolutely terrified. With my own work, we were trying to show we could predict a number of things using images and one of them was crime rates. I realised that the [data] that we had didn’t tell us who committed a crime, but which crime was reported or who was arrested.” In a context where black people are more likely to be deemed suspicious and arrested, Gebru realised that her dataset was biased, and, as a result, so too would be any algorithms trained on that data.
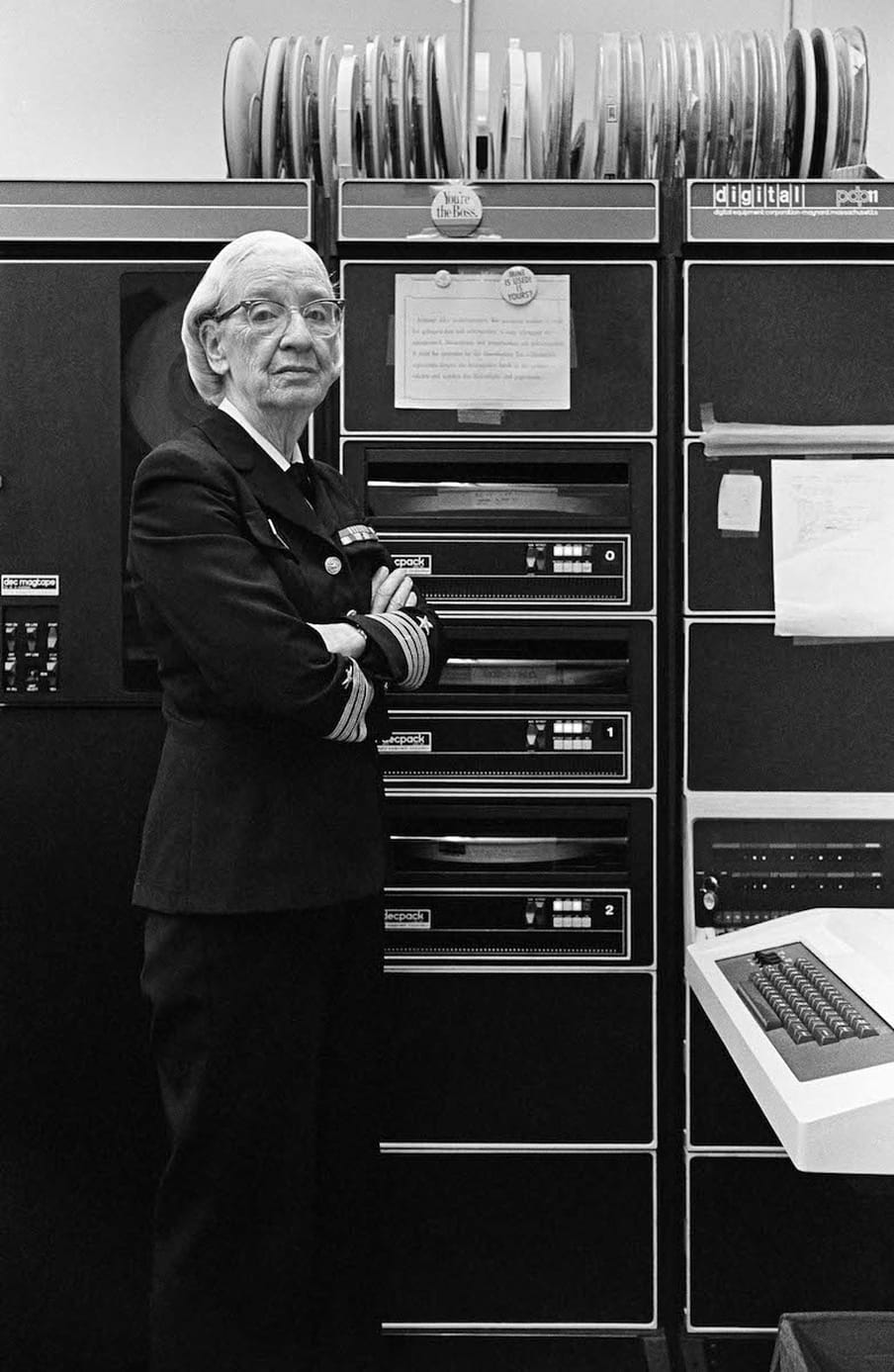
Put very simply, algorithms are the instructions that tell a computer what to do. I first started to think deeply about them when I listened to Cathy O’Neil’s talk in late 2017 about how these things I understood so little were “everywhere” – sorting and separating “the winners from the losers”. I was a little late to the party. O’Neil had published a book, Weapons of Math Destruction, the year before, giving that name to all algorithms that were “important, secret and destructive".
It turns out that those are more common than one might think. From determining whether your content is visible on social media platforms, or whether you get a loan and how much you pay for your insurance, to recommending films you should watch, what you should read, and even deciding the sentencing for someone convicted of a crime, algorithms were enabling computers to make increasingly complex decisions – decisions that affect both our lives and our democracies.
Learning this didn’t shock me. I have long understood as a woman, a black person, an immigrant (categories that are often spoken about and done for, rather than spoken to and done with) that developments that are brandished wholesale as “good”, “beneficial”, or “efficient” are often less so when your perspective shifts – that is, if you find yourself (again quoting Buolamwini) “on the wrong side of computational decisions”.
Harms that remain unnamed are always rendered invisible, so I started to seek out people naming the harms caused by algorithms – and working to prevent them, whether through advocacy, research or practice. Gebru, who is the co-lead of the Ethical Artificial Intelligence team at Google and one of the founders of Black in AI, may stand out in computer science’s "sea of dudes", but she’s certainly not alone. In fact, many of the people leading the charge to make algorithms and the data scientists more accountable seem to be women.
AI’s ‘white guy problem’
Kate Crawford isn’t convinced that somehow by virtue of their “femaleness”, women are drawn to working on bias in AI. “I’m always wary of essentialising gender,” cautions Crawford, co-founder of the interdisciplinary AI Now Institute at New York University, and an eminent voice on data, machine learning and AI. “Instead, what I tend to think of is that the people who have been traditionally least well served by these systems or experienced harm through these systems are the ones who are going to see the limitations earliest. ”
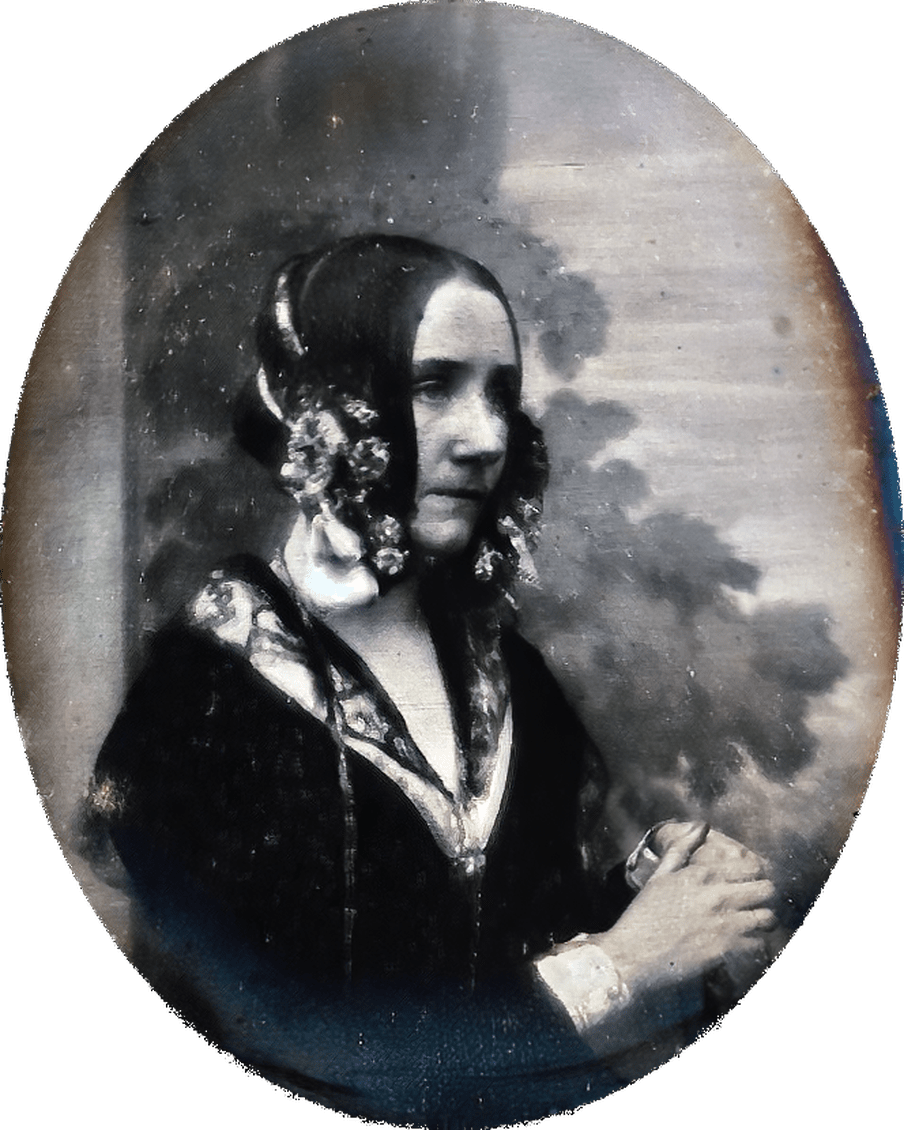
Crawford’s career is a thing of wonder and includes a stint in the Canberra electronic duo B(if)tek, which released three albums between 1998 and 2003. In 2016, she wrote for the New York Times about “artificial intelligence’s white guy problem”, having noticed that the imminent threat from machines wasn’t that they’d become more intelligent than humans (a theory known as singularity) but that human prejudices could be learnt by intelligent systems, exacerbating “inequality in the workplace, at home and in our legal and judicial systems”. She would also suggest then, as do all the women in this story, that the solution to this problem would have to be more than just technical.
“There are issues with how training data is collected and how it can replicate forms of bias and discrimination, and there are issues [with] the industry itself – who gets to design these systems and whose priorities are being reflected in the construction of these systems,” says Crawford in a soft Aussie twang. “Both of these issues are incredibly important, but are dealt with in very different ways.”
‘Society has so many inequalities. Anything not trying to mitigate them will simply reflect them’ - Zara Rahman
“It’s really important that as a research space we’re developing really granular and specific techniques for contending with training data. Separately, we need to talk about the industry: looking at the statistics of who is graduating in computer science and who’s being hired into the major tech companies tells a terrifying story of what’s been happening in terms of under-represented minorities. This isn’t simply a question of: ‘We need to change the demographics in undergraduate education.’ We also need to think about practices of inclusion within tech companies.”
Zara Rahman very much agrees. The deputy director at the nonprofit organisation The Engine Room is keen to stress that the problems made visible in algorithmic bias are all systemic in nature. “Society has so many inequalities. Anything not trying to mitigate them will simply reflect them. And AI reflects the biases of the past into the future,” Rahman says.
But how do these systemic issues play out? What exactly does she mean when she says AI bias is connected to systems of exploitation as old as slavery and as enduring as patriarchy?
“Tech being built in the US is pushed out to poorer countries with an imperialist attitude that doesn’t take into account different cultural or social contexts,” Rahman explains. She gives the example of Facebook in Myanmar, where the tech giant was accused in 2018 by the United Nations (UN) of doing little to tackle hate speech. The UN said “the extent to which Facebook posts and messages have led to real-world discrimination must be independently and thoroughly investigated”.
How is this an AI problem? Well, Facebook’s algorithms determine what you see on the platform, as well as what meets or doesn’t meet the social network’s moderation standards. If – as a Buzzfeed investigation found – “lawmakers from the home state of Myanmar’s persecuted Rohingya minority regularly posted hateful anti-Muslim content on Facebook and, in some cases, explicitly called for violence”, there is a failure in the algorithm to spot and treat these posts as hate speech. Rahman believes that failure happens because Facebook is so disconnected from the places where the platform is being used.
“For all the [tech] we rely on, all the decisions are being made in [Silicon Valley] a very small area of the world that then affects the entire world … Of course bad decisions [are being made]!”
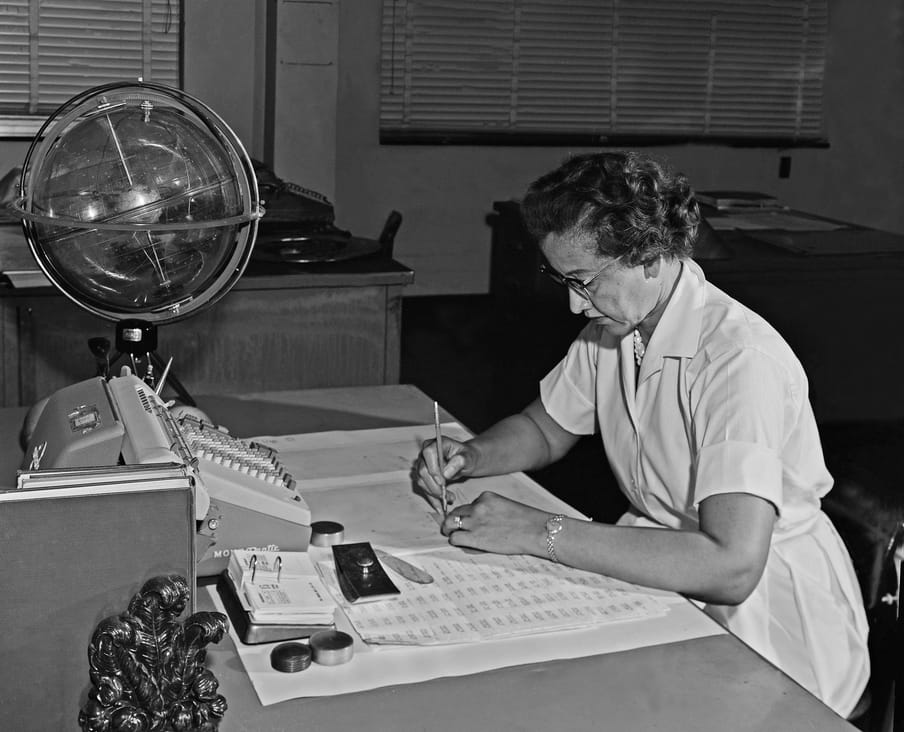
Mathwashing: enabling tech companies to get away with murder
For Madeleine Elish, the increasing use of algorithms by the public sector and the visibility of controversial cases, such as the one covered by former ProPublica reporter Julia Angwin and her team, highlight the challenge of trying to translate a social problem (such as who is likely to reoffend, or who should have access to social benefits) into a technical problem with a technical solution. Doing so will “involve a lot of trade offs which will surface biases, conscious and unconscious,” says the cultural anthropologist, whose work examines the social impacts of machine intelligence and automation on society.
“There is no neutral translation,” Elish adds. "The question is now what is fair, and can you have a statistical definition of fairness?”
While the number of articles on AI bias has grown exponentially – with headlines that read “AI is sending people to jail – and getting it wrong” – so too has interest from policymakers to regulate the field. But the quality of public discourse lags behind, in part because we cannot tell when algorithms are being used.
Another reason we ask so few questions about the nature, purpose and impact of algorithms is due to a phenomenon known as “mathwashing”. Coined by data scientist and former Kickstarter executive, Fred Benenson, mathwashing is “the widely held belief that because math is involved, algorithms are automatically neutral”. Similarly, Elish has written about how the media’s tendency to call AI systems “magic” renders invisible the human labour that makes these computer systems work. “We often overestimate the capacities of machines,” Elish says, “and underestimate the capacities of humans”.
To make this point, she gives the example of the test subjects in a study carried out by Georgia Tech, who still followed an “emergency guide robot” towards a fire rather than away from it, even though it had already proved itself unreliable.
‘We often overestimate the capacities of machines and underestimate the capacities of humans’ – Madeleine Elish
Mathwashing is itself possible because tech companies are evasive about how their hardware and software work. Both the language and the design make them difficult to scrutinise, explains Rahman. Saying that today’s tech is impenetrable isn’t just a nice metaphor. Increasingly devices are literally sealed shut, or tech infrastructure spoken of in ways that give us, the general public, no clear sense of what it is and how it works.
“Take the cloud,” says Rahman. “No, it’s not stored ‘up there’, it’s stored in very real, concrete data centres. The ‘cloud’ metaphor allows us to forget the often personal nature of the data, and the physical or environmental consequences.”
“We’re presented with a shining interface and can’t go any further. It’s like having a car,” she adds, “you don’t need to build one yourself but you do need to know how to change the oil or, at the very least, know that there is oil and petrol and that’s what makes the car run. Companies want us to leave how to run the car to the tech experts.”
We need better conversations about where, when and how to use algorithms
Immunologist Stephanie Mathisen wasn’t about to leave anything to the experts when she persuaded the UK House of Commons Science and Technology Committee to take a closer look at the use of algorithms for decision-making. “I wrote something for the sci-tech committee about how – without debate – algorithms had just come to make decisions about people’s lives: your credit score that factors into whether you get a loan, a mortgage, maybe even a job. These are massive points in people’s lives and totally impact the paths people can take,” she says, leaning forward as she talks, quickly, passionately, holding my gaze.
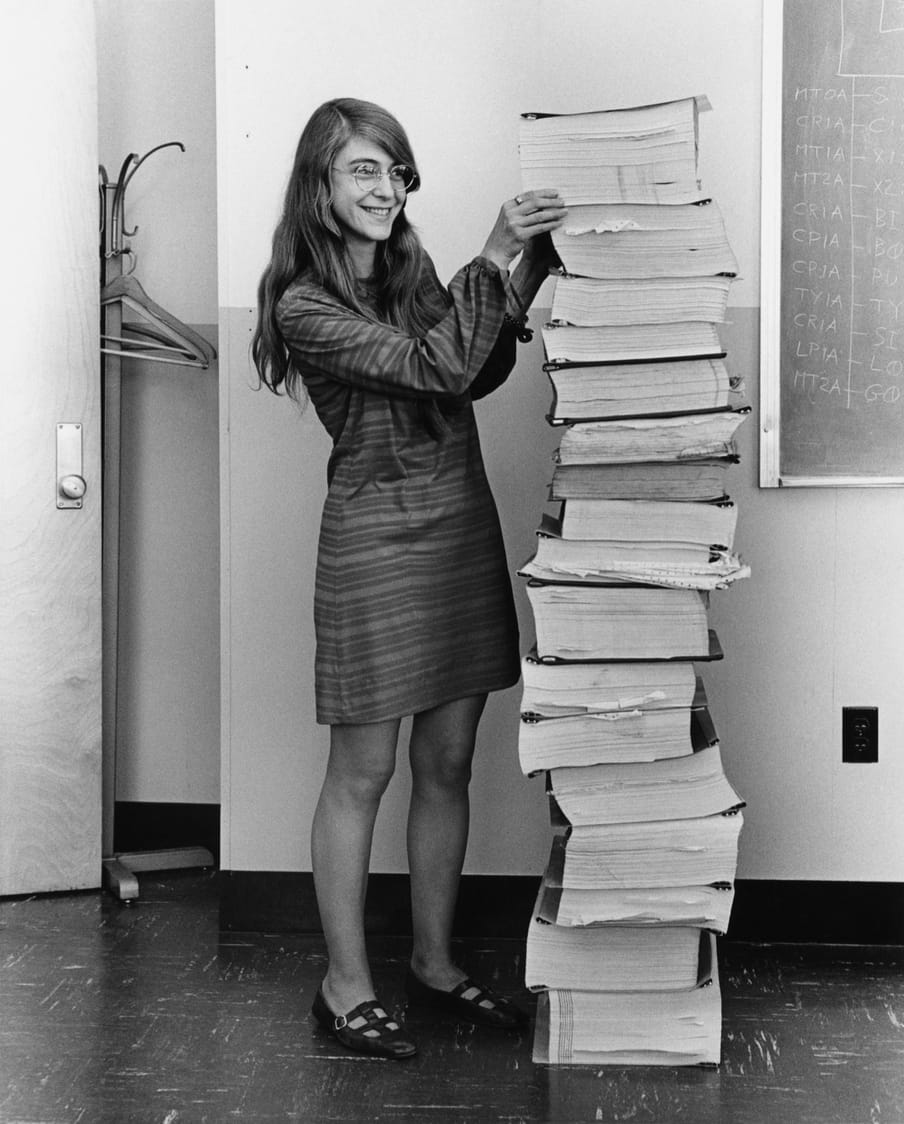
“We all know we have a credit score but who knows exactly how it’s calculated? You can guess at a few things, but don’t really know. I just don’t know that that’s acceptable. Committees are powerful and I want citizens to see parliament committees, and their scrutiny of government function, as the line of accountability,” says Mathisen who, when we met, led the evidence-in-policy work at the campaigning charity Sense about Science.
Mathisen agrees that public discourse around algorithms is sorely lacking. Meeting late morning, she initiates a search for the holy grail: a cafe which is both relatively quiet and has good coffee near her office in London’s trendy Farringdon neighbourhood. We end up in a spot that delivers on the latter but fails spectacularly at the former. Between the banging of metal as coffee grounds are emptied, the purring sound of frothing milk, the pop music playing on the sound system and the sounds of other people’s conversations, she and I are enveloped in cacophony. It all feels too much like another metaphor for talking about AI: a couple of people trying to say sane, measured things, in a room full of buzz about AI as the fix for everything from journalism to banking.
“There’s so much hype [about data and algorithms] but none of us,” she says, gesticulating as she looks around the cafe, “know what [politicians, the AI evangelists and the media] are talking about. We’ve gone past the point where people feel able to stop and say: ‘Wait, what is big data?’”
So what would doing this debate right look like then? “Three-parent babies,” comes the answer.
Mathisen cites the public consultation around mitochondria transfer as an example of how complex, emotive subjects can, and should be open to public scrutiny. After researchers developed a procedure by which the DNA transferred from a third person could prevent mothers passing on genetic disorders – hence the name “three-parent babies” – a proposal to allow doctors to carry out the procedure was opened to public consultation. In 2015, it was voted into law.
“That discussion was perhaps second to none in terms of public engagement. Involving researchers and experts, patients (in the form of parents), the public and politicians, that standard of debate meant that Britain is the first country to legalise having that technique available.”
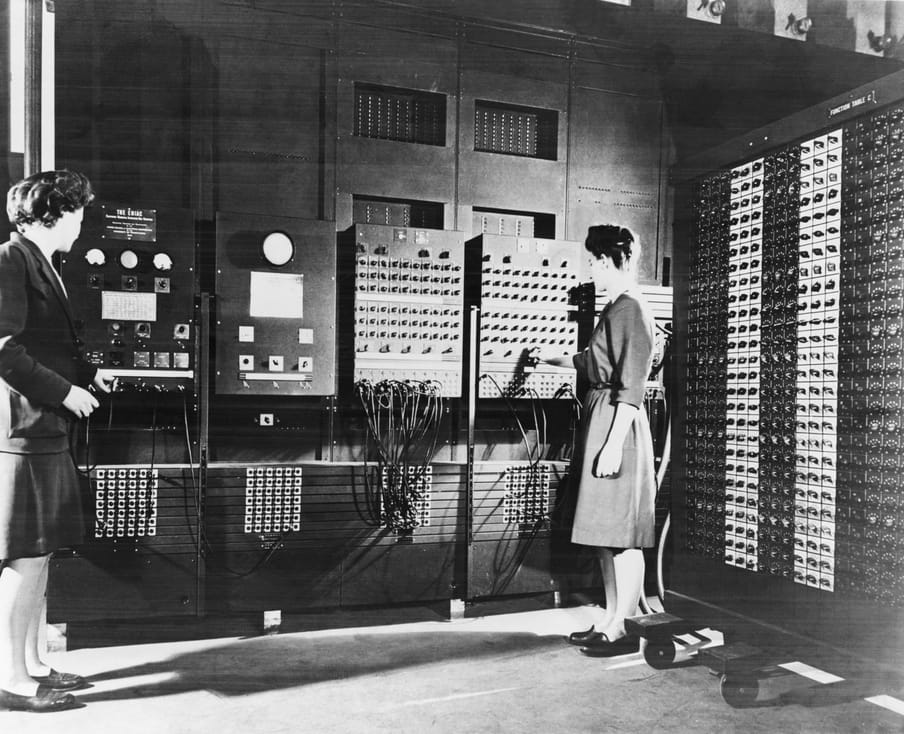
Whodunnit? Figuring out responsibility and accountability in an age of AI
There are many questions that remain unanswered as AI moves us collectively into new economic, social, cultural and even political territory. Questions around responsibility are some of the knottiest to disentangle: who is responsible when you are discriminated against by a machine and not a man? The organisation that deployed the algorithm or the one that developed it? The person who enforced the decision reached by the machine or the one who signed up the organisation for its use in the first place?
“True fact: there is not a single proven case to date of discrimination by machine learning algorithms,” tweeted Pedro Domingos in September 2017. Challenged to explain himself in the face of story after story of algorithmic bias, the professor of computer science added: “I meant discrimination proved in court where the culprit was the [machine learning] algorithm, not the data or the people using it.” This may seem like semantics, but Domingos gets at an important point: if it is easier to blame the technology, the problem with the people who build the technology will never be addressed – and for that there is no quick fix.
Who is responsible when you are discriminated against by a machine and not a man?
Still, much progress has been made.
AI Now developed Algorithmic Impact Assessments (AIAs), which Crawford describes as “a clear step-by-step system that public agencies can use to really dig into whether or not an algorithmic system is something they should deploy and, really importantly, how do you begin to include communities into this decision-making process.” AIAs have since been discussed or used by policymakers from the European Union to Canada.
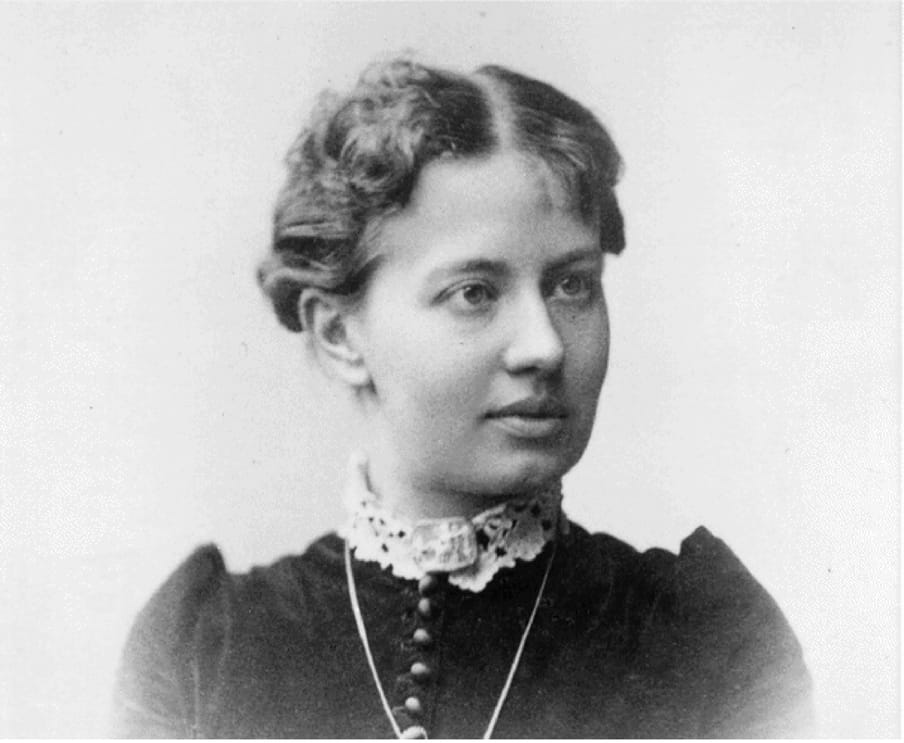
After Mathisen left, Sense About Science has continued its work to help the British public understand AI – “particularly empowering people to ask about its reliability,” the organisation’s director Tracey Brown writes to update me. Brown talks about creating “a confident culture of questioning”.
Taking a leaf from the electronics industry where each component is accompanied by usage information, Gebru proposed datasheets for datasets. Compared to nutrition labelling for food, these datasheets show “how a dataset was created, and what characteristics, motivations, and potential skews it represents”. The aim? To make the AI community more transparent and accountable for the things it creates. Elsewhere, with their work on Black in AI, Gebru and co-founder Rediet Abebe have been able to facilitate access to the largest academic machine learning conference, NIPS, for more black people – increasing the number to about 500, compared to a pitiful half dozen four years ago.
For someone who says she sometimes just wants to sit in a corner writing code, Gebru is rather busy. “The people in the bias and fairness community are mostly women and people of colour because it affects us,” she says, echoing Crawford. “I liked building things and solving technical problems, but if we don’t do it, who else is going to look out for us?”
Dig deeper
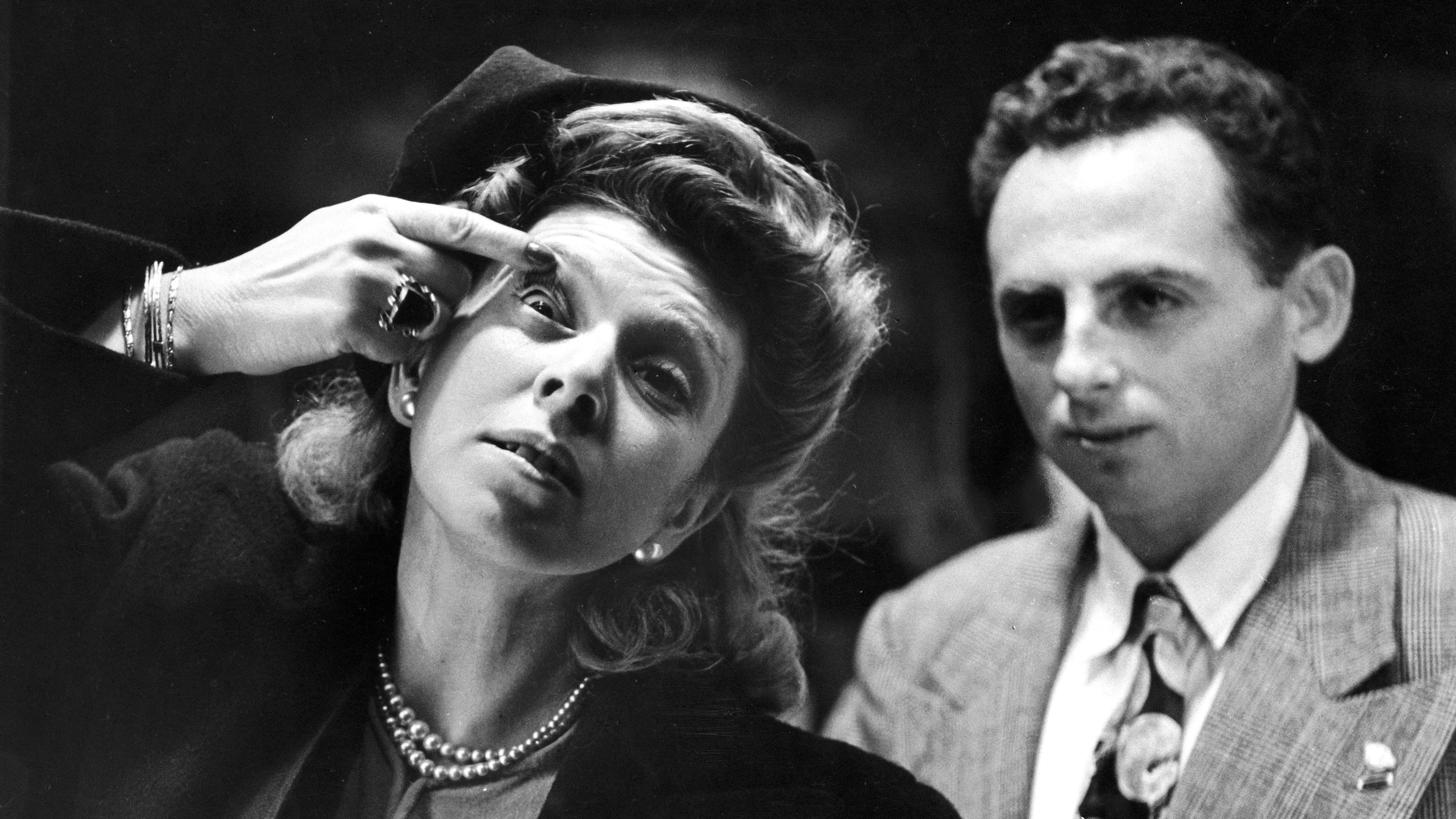