The piece you’re reading was translated with technology.
I first wrote it in Dutch, then ran it through DeepL, a translation tool we’ve recently subscribed to at The Correspondent. Everything I write in Dutch also appears in English, so I’ve become very familiar with the technology lately.
After running the text through the software, I checked the translation and adjusted a few things. For example, a Dutch idiom came out of DeepL as "I do speak a little word about the border". (Even advanced technology still translates idioms literally.)
When I was done, I forwarded it to my colleague Shaun, the copy editor at The Correspondent (although DeepL promoted "copy editor" to “editor-in-chief”). He polished up some sentences, came up with a witty headline and – voilà – the piece was ready.
But aren’t we forgetting someone? Oh yeah, the translator.
And who created a piece of translation technology like this? That’s right – translators. After all, translation tools “learned” the work from them. So, ironically, it’s because of translators that we won’t be hiring a translator for this piece.
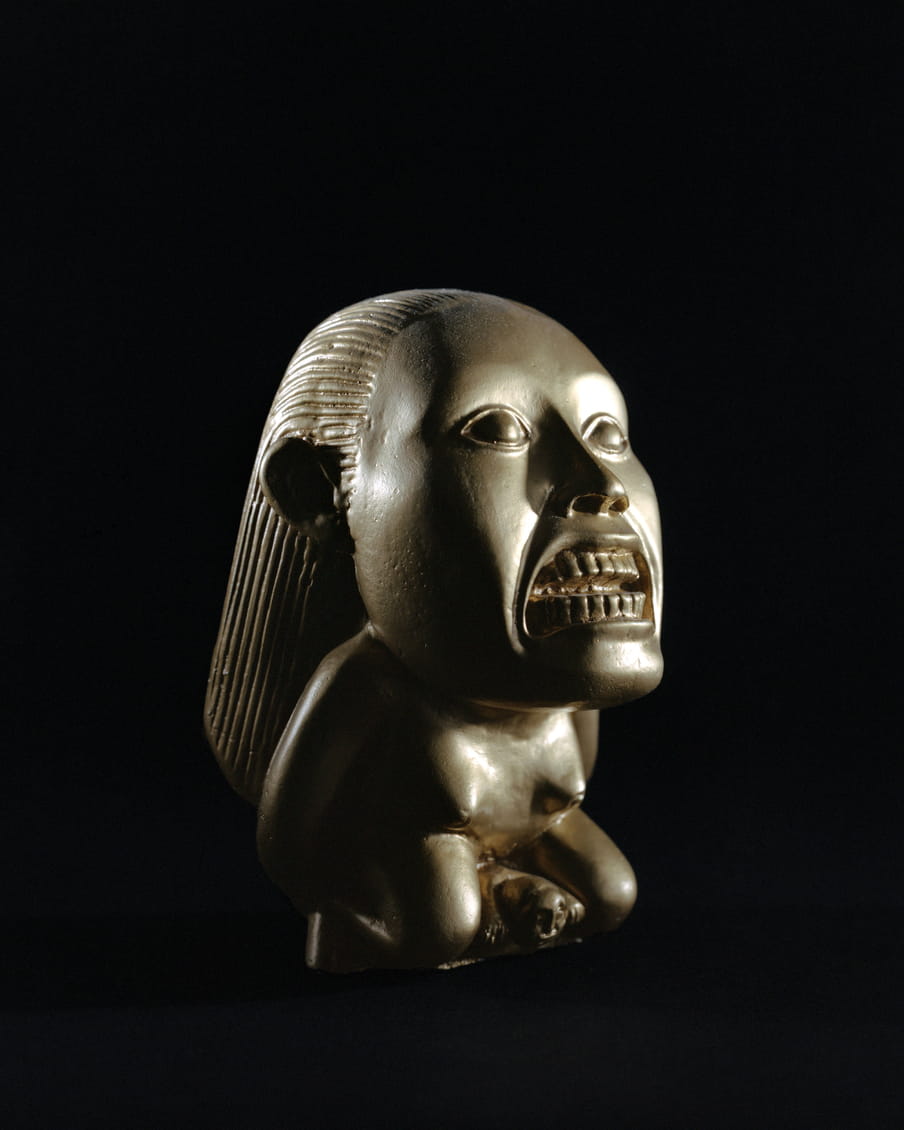
This is not only bad for them, but potentially for us as well. A translator filters information and, as such, influences our understanding of the world.
If a translation tool makes a mistake – whether in a manual, a contract, or a piece of literature – then that has consequences.
So is it OK for me to use artificial intelligence (AI) to translate my pieces?
Translation software has come of age
Translation tools have taken off in recent years. While in the past you could laugh heartily at Google Translate’s clumsy texts, the translations are really not that bad nowadays.
For example, say I run the first line of Leo Tolstoy’s Anna Karenina through DeepL: “Все счастливые семьи похожи друг на друга, каждая несчастливая семья несчастлива по-своему.” Translator Constance Garnett came up with: “Happy families are all alike; every unhappy family is unhappy in its own way.”
And here is what DeepL gives me: “All happy families are alike, every unhappy family is unhappy in its own way.”
This is an extremely close rendition – only the word “all” is in a different location and the semicolon became a comma. Pretty impressive, right?
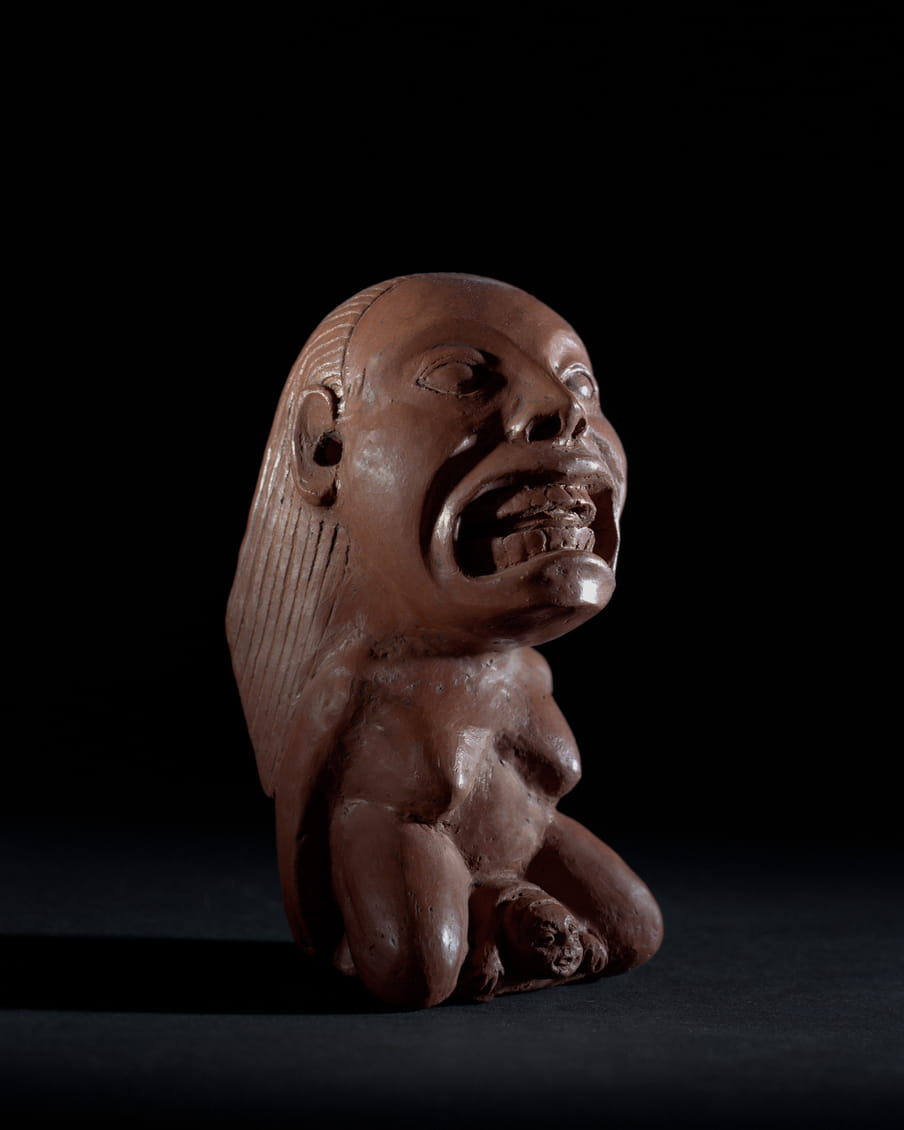
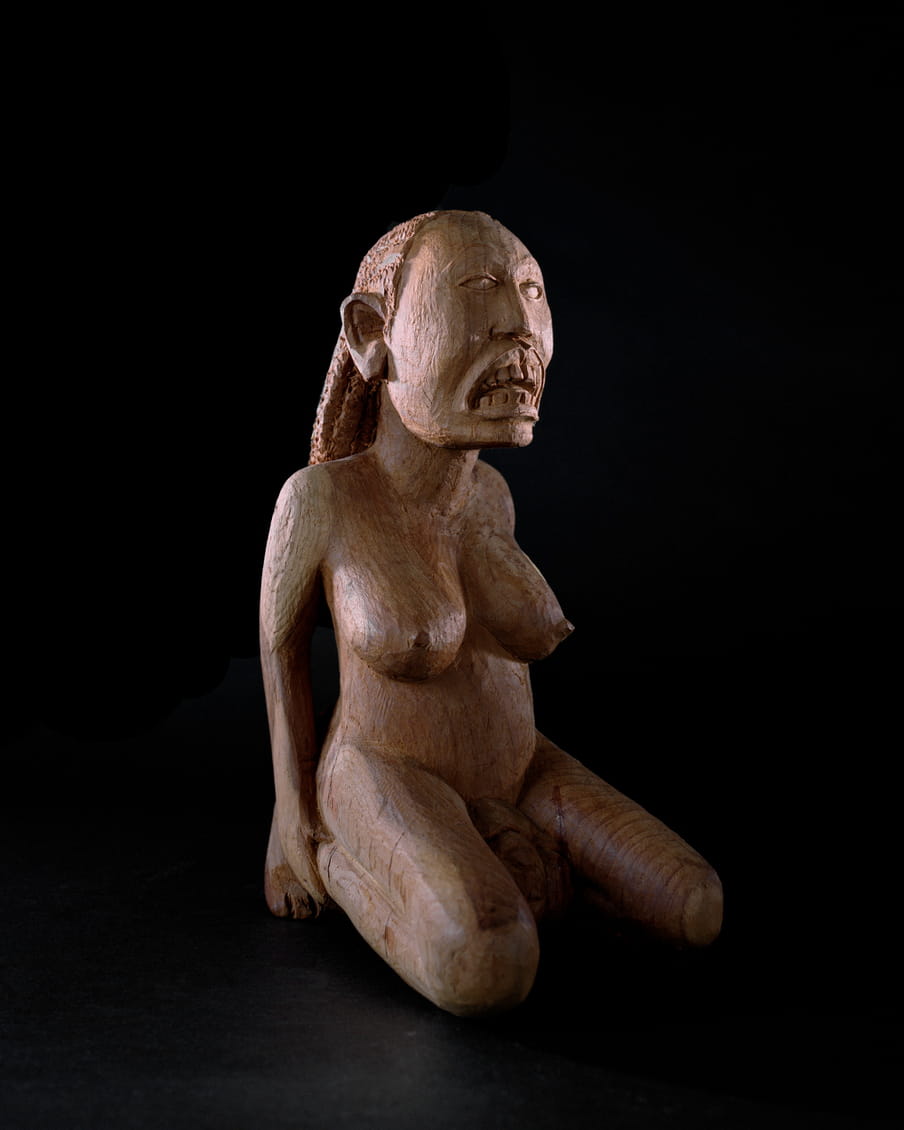
This progress is not just based on a feeling – there’s also data to quantify it. When Google introduced a new translation method in 2016 – Google Neural Machine Translation (GNMT) – the company showed that the gap between translations by humans and Google had narrowed significantly. Bilingual test subjects invariably gave the new model a better score than the old one.
Even as you read this, the data will have changed again because translation technology is constantly improving. So the fact is, it’s getting better and better. How did the programmes get so good? And do they pose a threat to translators?
Why translation tools don’t work word for word
To answer that question, we need to take a look under the hood. How does a translation programme work?
Let’s start with the simplest version. You could have the computer pick up the dictionary entry for each word and write down the translation of that one word. But even in a simple sentence, that goes wrong.
Take the first sentence of Dutch novelist Herman Koch’s international best-selling book The Dinner. In Dutch it’s: “We |gingen |eten |in| het | restaurant”, which means “We went out to eat at a restaurant.”
But that’s not what you get when you translate word by word: "We|went| food|in|the|restaurant.”
It goes wrong because the software does not understand grammar. First of all, in this context, “in” should be translated as “at” when it comes to a restaurant. But, even worse, “eten” in this context is not a noun (“food”), but a verb (“to eat”).
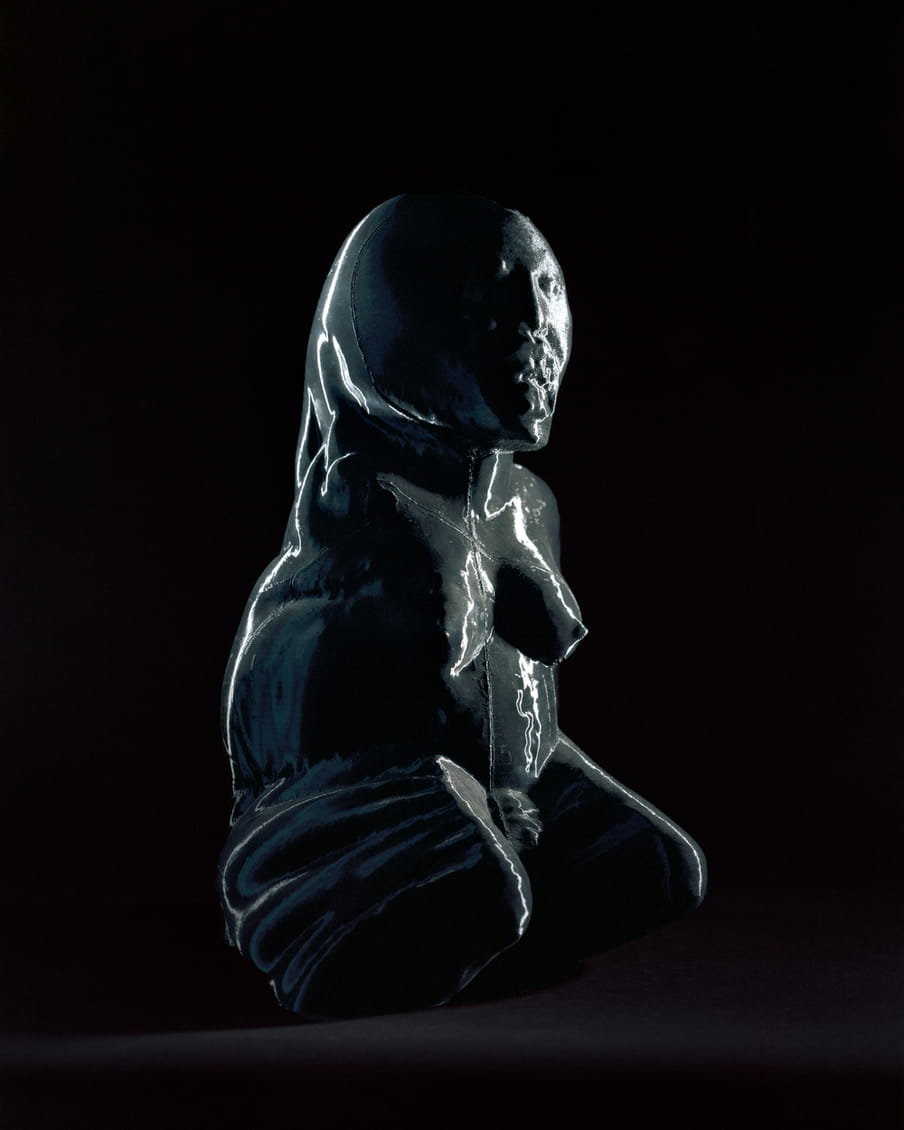
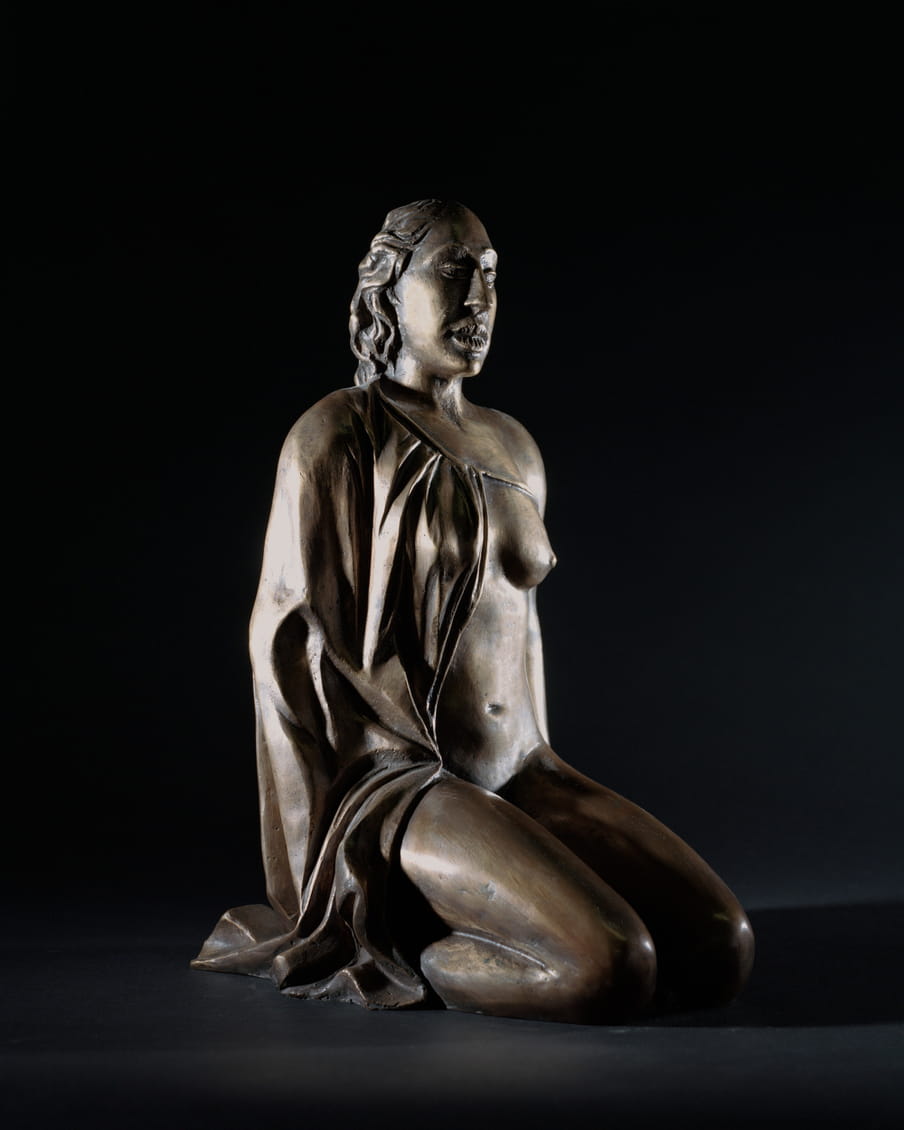
So you’d need to give the programme an idea of how language works. You could write down specific rules: for example, that a word after "gingen" is probably a verb. Then again, it’s pretty easy to come up with an example that disproves that. Maybe we should add that if you have "gingen" before and a preposition after, a word is probably a verb.
You see, it gets complicated pretty quickly. It is difficult – if not impossible – to write down all those grammatical rules.
And then language is also subject to change. Rules change. New phrases or words such as “to Tinder” and “cannabusiness” throw a spanner in the works.
Enter machine learning
Enter machine learning. Instead of spelling everything out, you give the computer millions of sets of translations:
- La penna é sul tavolo. / The pen is on the table.
- Volevo un gatto nero. / I wanted a black cat.
- Quando andiamo in vacanza? / When are we going on vacation?
From all these combinations, a machine learning programme learns how the language works and gives – you hope – a correct translation of a new sentence.
What exactly does such a programme look like? First, there’s a “neural network”, a complex and self-learning computational system that converts a sentence into numbers so that the computer can read it. This network is called the “encoder” because it encodes the words. Another neural network, the “decoder”, converts that code into the words of another language.
Until recently, such an encoder-decoder programme was the best of the best. Still, it didn’t work perfectly. If a sentence was too long, for example, the programme would get confused.
One of the reasons was that the programme only reads one way, from front to back. So when translating a sentence, it looks back at the words that came before and, based on that, comes up with the best translation of the next word.
The only thing is, language also depends on the word that comes next, not just the one that comes before. That’s why the first improvement was a programme that looks both forwards and backwards – a neural network that is bidirectional.
But there’s another problem: it’s not always clear which other words from a sentence are important for the translation of a word. If you want to translate “restaurant”, it doesn’t seem so important what the other words in the sentence are. A restaurant is a restaurant, regardless of whether you are going there to eat or to kill someone, or whether you’re describing a restaurant in Wollongong or Wichita.
But as we already saw, the meaning of the Dutch word "eten" was influenced both by the word that came before and the one after. That’s why you want to place an attention mechanism between the encoder and the decoder. Again, this is a neural network, which this time is trained to recognise words that are also important in the translation of a certain word.
And there you have, roughly speaking, a system such as Google Translate: an encoder, a decoder, and, in between, an attention mechanism.
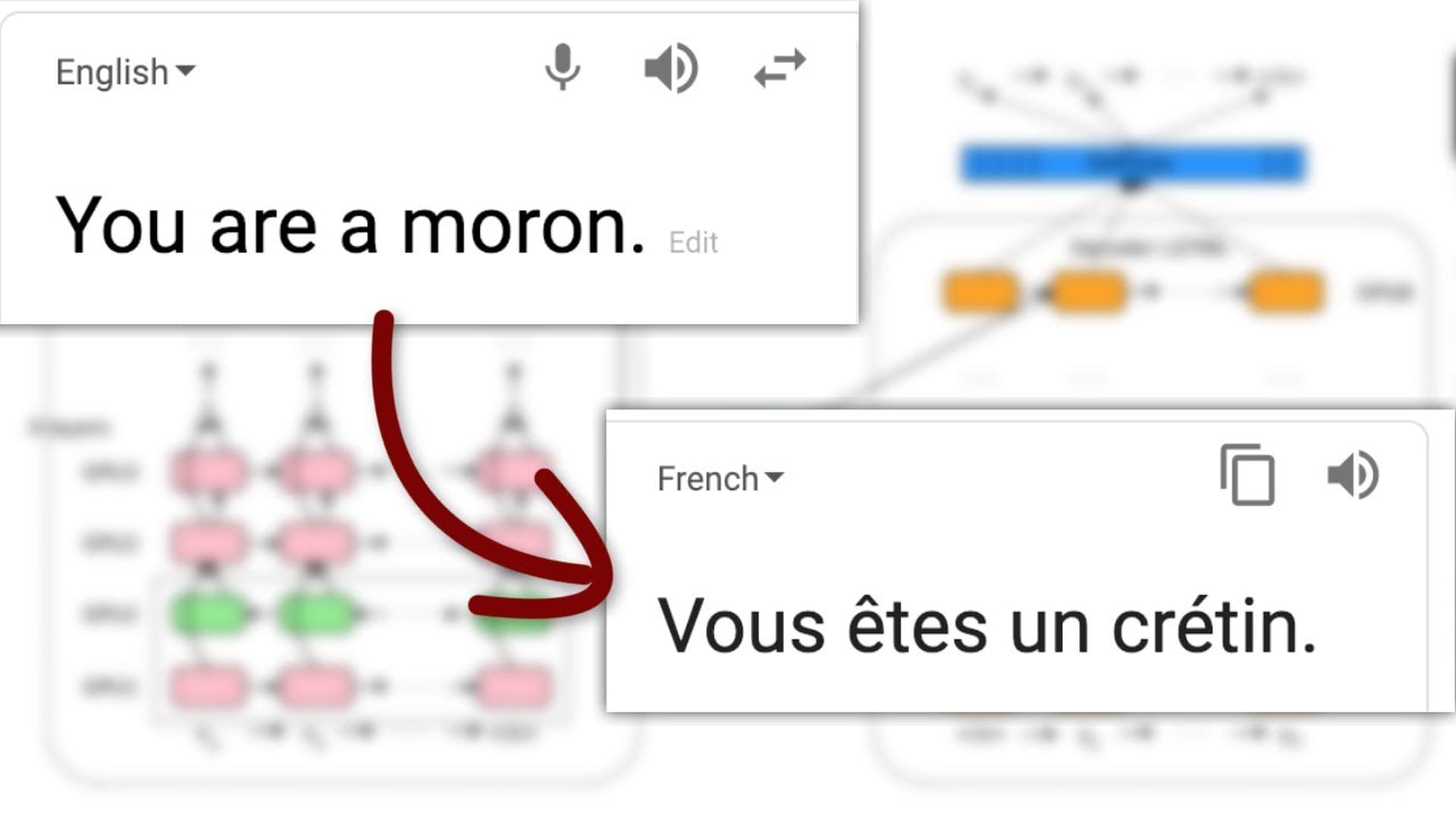
Where’s the data coming from?
But now comes the catch. The model is beautiful, but it only works if you have data. Heaps of data. After all, the machine has to learn from something.
Official texts from international organisations lend themselves perfectly to this. At the European commission, for example, texts are translated into the 24 official languages of the European Union by professional translators. And at the United Nations, documents appear in Arabic, Chinese, English, French, Russian and Spanish.
So there are “parallel corpora”, large collections of the same sentences in two languages. There’s a Bible corpus, a news corpus, a Wikipedia corpus. And Google, it won’t surprise you, also uses data collected by the company itself.
To give you an idea of how big such datasets are, an English-German dataset that Google used to train the system in 2016 counted five million pairs of sentences. For English-French, it was as many as 36 million. This would allow you to fill almost 5,500 books, if you take the first Harry Potter novel, which has 6,619 sentences.
But behind each of those millions of sentences is a human being, the translator. Someone who has waded through boring government documents, who has scratched their head pondering how to translate a tricky sentence from the Bible, or who has consulted a style guide to know whether to use “average” or “mean”.
And those translators could now lose their jobs.
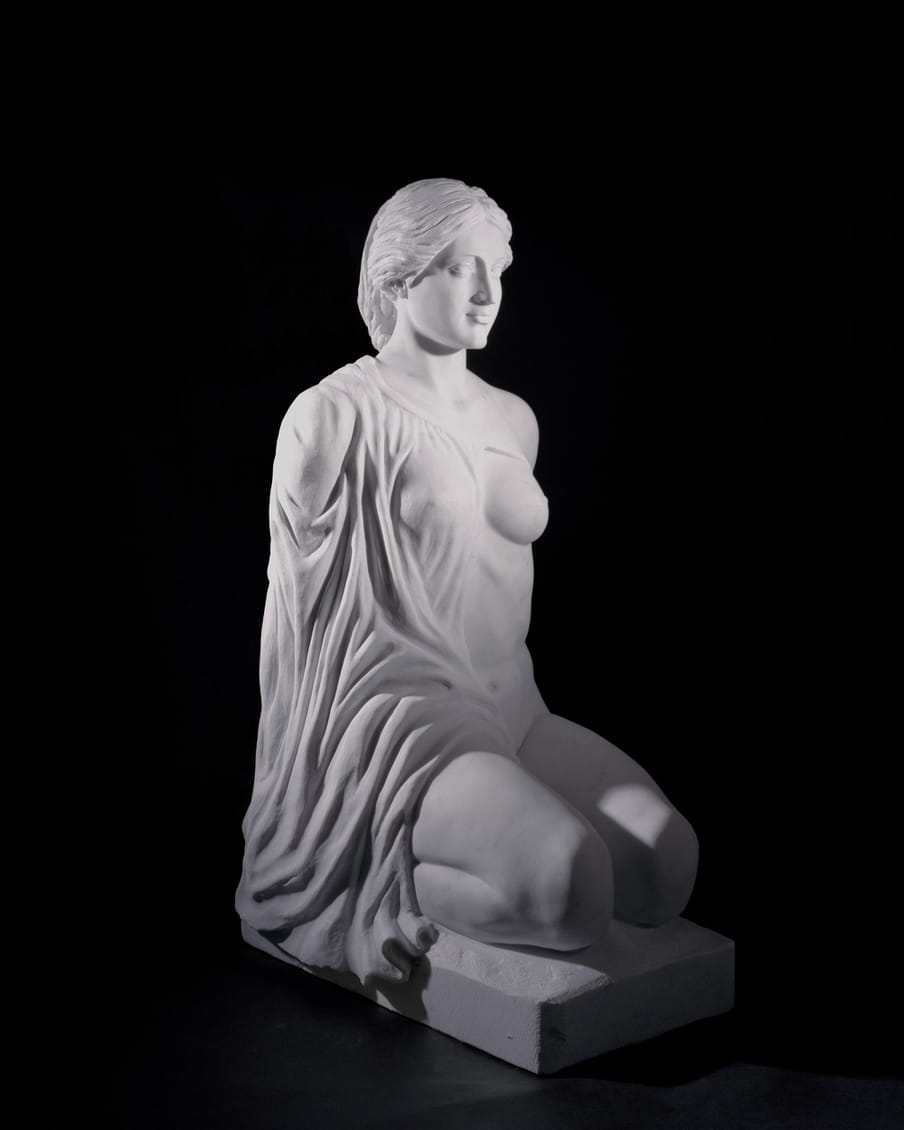
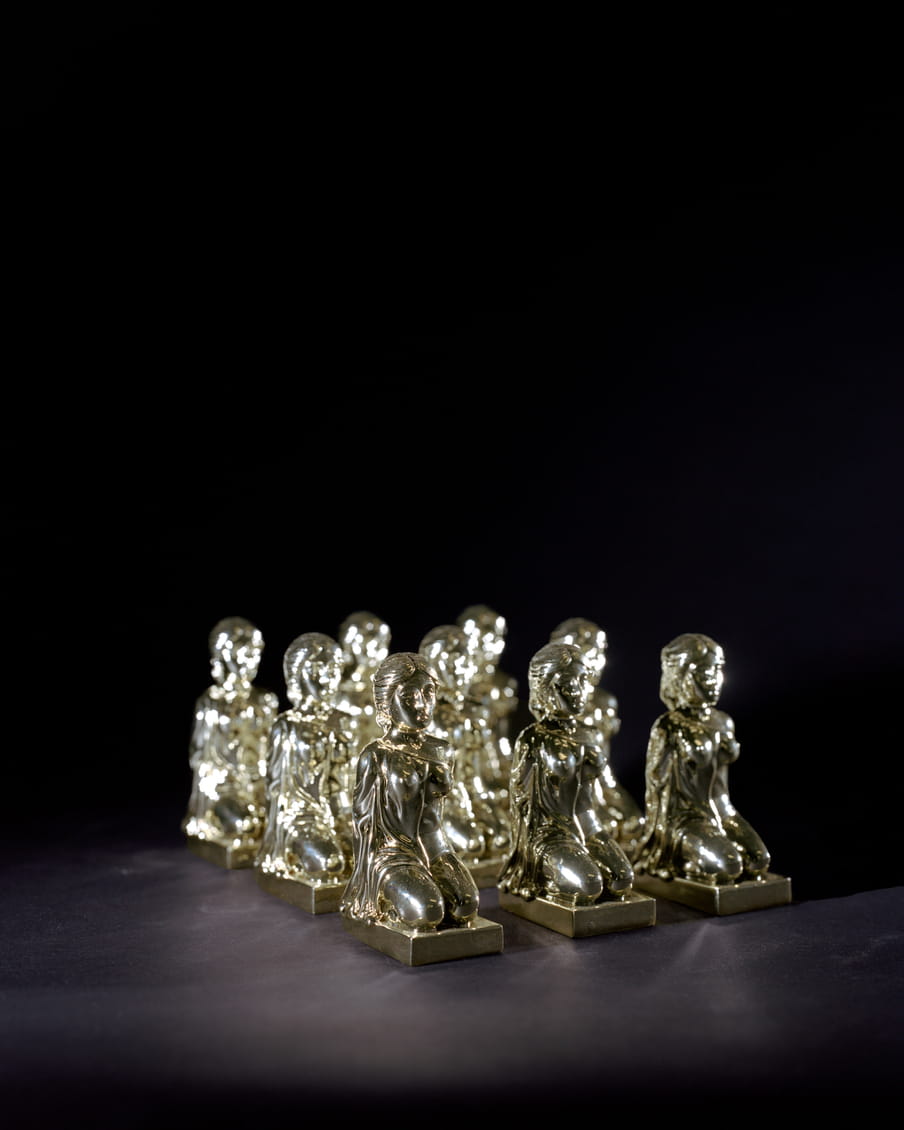
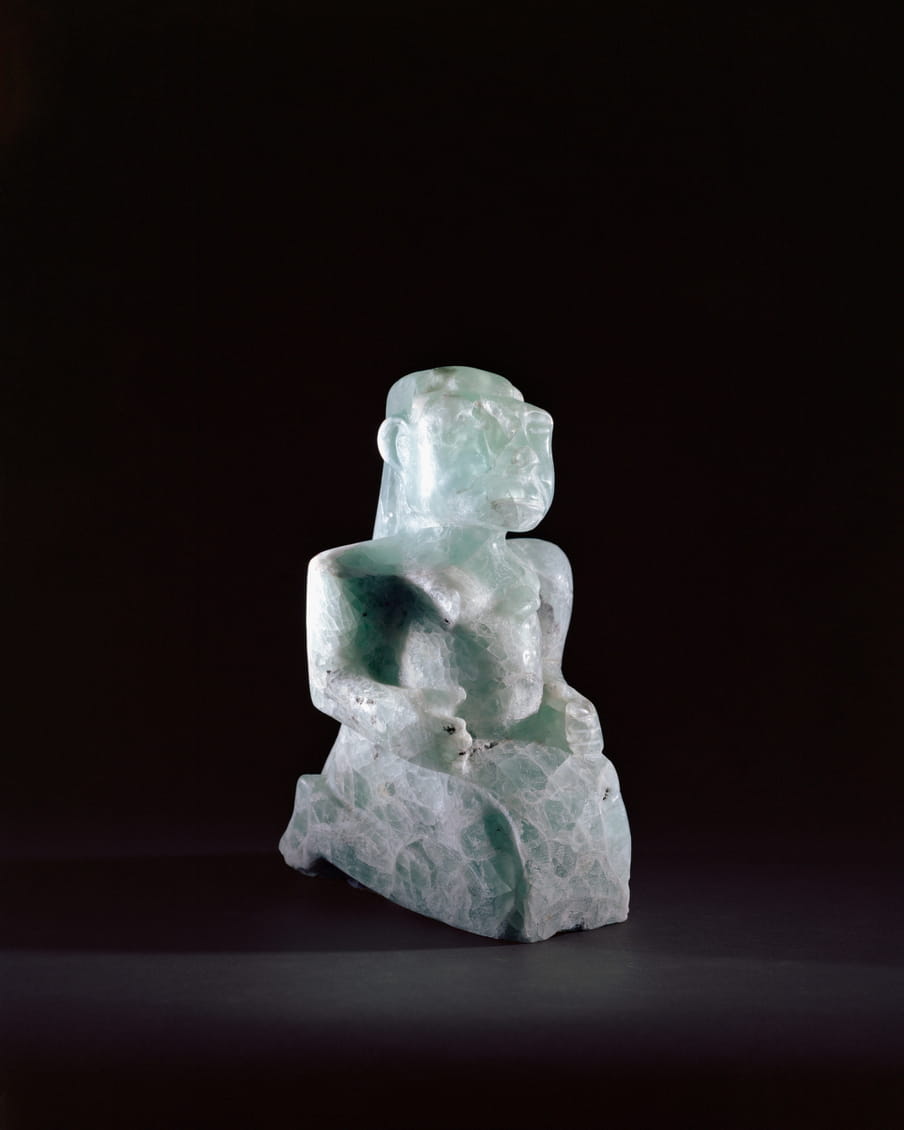
Translating Harry Potter
How threatening is translation technology to translators? Let’s take a look at the first Harry Potter book, which has been translated into 80 languages, including Scots (Harry Potter and the Philosopher’s Stane) and Latin (Harrius Potter et Philosophi Lapis).
In its original English, the book opens: “Mr. and Mrs. Dursley, of number four, Privet Drive, were proud to say that they were perfectly normal, thank you very much.” I ran that English first line of the book through DeepL into Dutch and it came out pretty much the same, except it was “thank you” instead of “thank you very much”.
Grammatically it’s fine, but that doesn’t mean the translation is good. “Thank you very much” is not so much the standard “thank you”, but rather it’s a way of setting the tone. You immediately feel a kind of measured, somewhat hostile atmosphere. And if you know the books, you know that suits the Dursleys.
Grammatically it’s fine, but that doesn’t mean the translation is good.
Speaking of, the translation technology simply translates Dursley as Dursley. Writer JK Rowling named the family after a place in Gloucestershire, near where she grew up. Only, just like “Privet Drive”, “Dursley” doesn’t say much to a Dutch reader.
For "Privet Drive", the Dutch translator Wiebe Buddingh uses “Ligusterlaan” (because “Privet” is the English name for the Liguster plant and "laan" which comes close to "Drive") and “Duffeling” for “Dursley” (which sounds like Dursley and has “duf” in it, the Dutch word for “dull”).
How about "thank you very much"? Buddingh doesn’t translate it directly, but does glue some sentences together.
By using the words “that kind of nonsense” in combination with a long sentence, Buddingh achieves the stiff, haughty and unfriendly tone of the original. Reading this translation, you can see immediately that there’s a human at work.
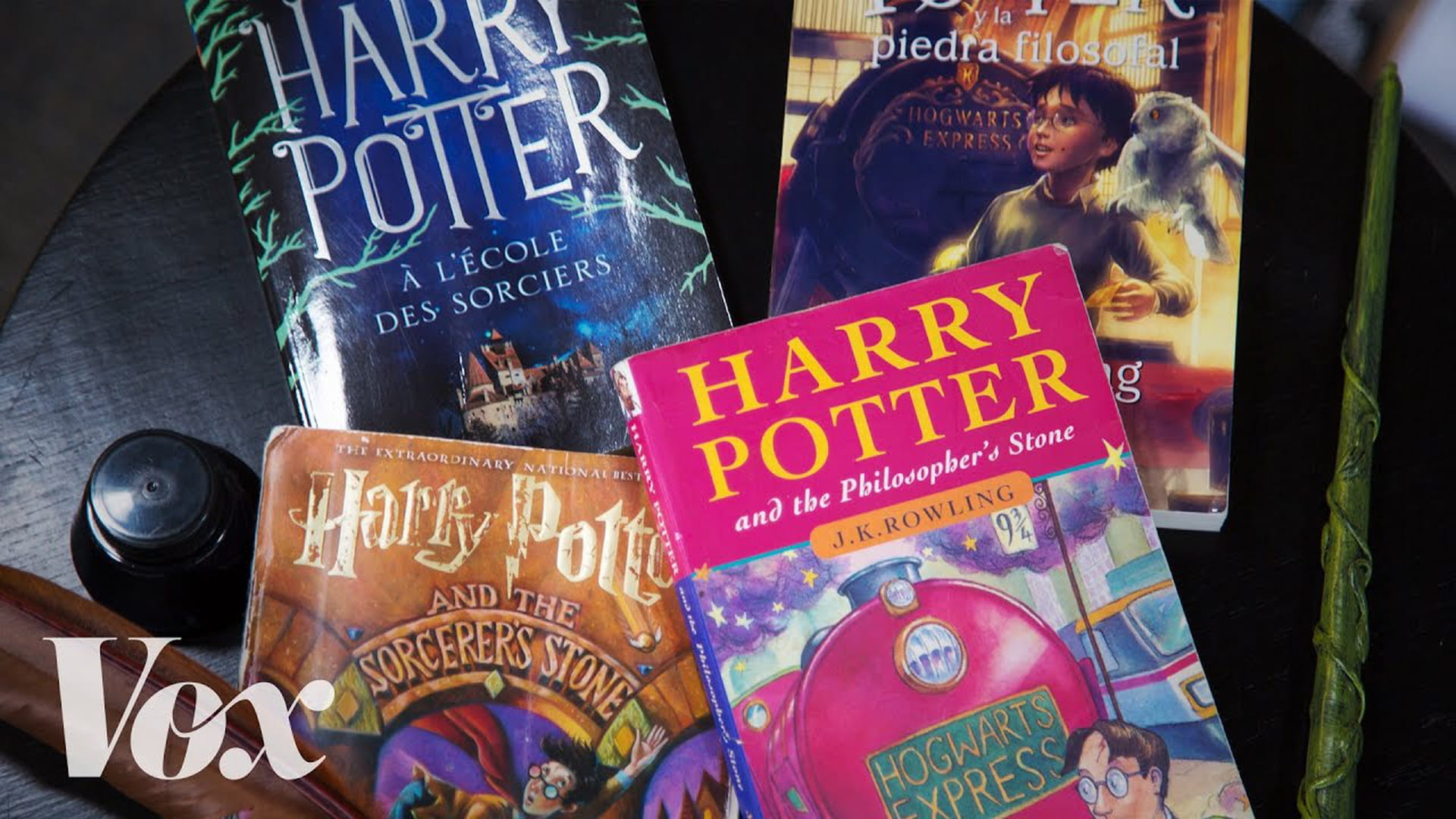
Programmes process, but they don’t understand
When you hear “artificial intelligence”, you’d be forgiven for thinking the programmes could actually think. But nothing could be further from the truth. They are models that calculate which sentence will be the best. No more, no less.
Programmes process, but they don’t understand. They don’t understand what rhythm is, what the reader knows, how to recognise sarcasm. For something as standard as a weather forecast or a government document, machine learning can still work quite well, but after that it quickly goes downhill.
AI programmes don’t understand what rhythm is, what the reader knows, or how to recognise sarcasm.
Programmes are great for making a rough version of a text. Translators, the human kind, sometimes use such software themselves. They produce a text and then get down to work on the fine details.
This is exactly what I’ve done with this piece. It’s not like I’m using software to translate it to Chinese and then publish it unchecked. I read it myself and then had it checked by an English-speaking colleague with a good understanding of the language. And we’ve thought long and hard on how to convey many parts of this piece to an international, non-Dutch-speaking audience.
In short: for mechanical parts of translation, AI programmes do pose a threat to the profession. But for the other, more creative part, we can’t lose translators, thank you very much.
This article was updated to give the correct title of the first Harry Potter novel in Latin.
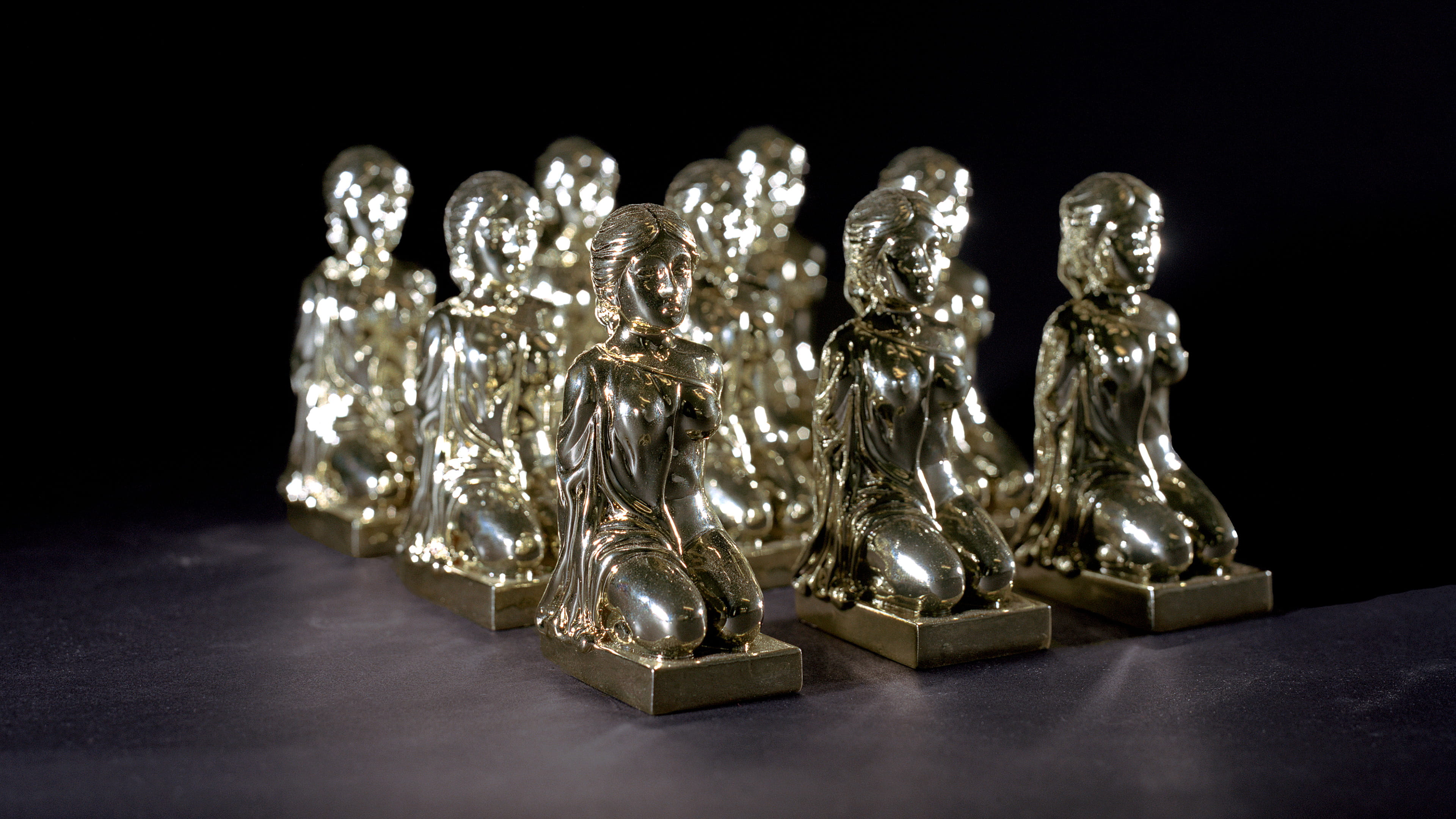
Dig deeper
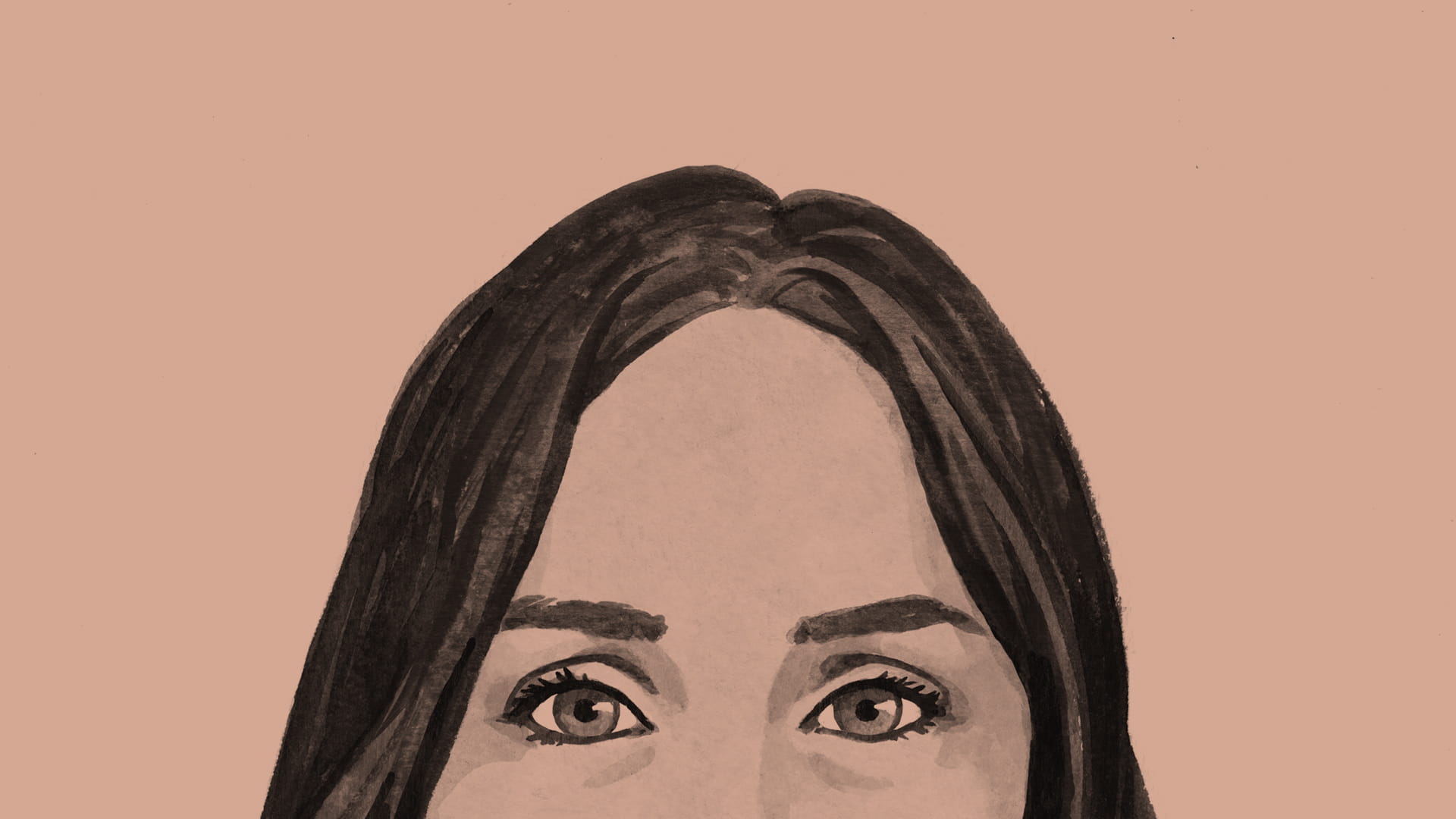