Hi,
"It’s Orwellian when it works, it’s Kafkaesque when it doesn’t." That’s what Frederike Kaltheuner, an expert on technology policy, said at a meeting at the European parliament last week about automated discrimination. She was talking specifically about facial recognition.
Nakeema Stefflbauer spelled out the Kafkaesque side of technology during the session. After being offered a pre-approved credit card by her bank, she had to identify herself. She sent a scan of her driver’s license, which didn’t work. Then a selfie. Again nothing. After she had finally sent a scan of her passport, she was told: "We can’t identify you." Nobody knew why, but it didn’t work out.
Stefflbauer also referred to the example of Amara K Majeed, a student accused of involvement in bombings in Sri Lanka. The government put her photo online to track down the perpetrators. But next to her photo was not her own name. Instead, it said: Fathima Qadiya, the name of a suspected bomber. Facial recognition software had wrongly recognised her. The police admitted the mistake, but it was already too late. Majeed received numerous death threats.
But if facial recognition does work, it can be just as frightening. For example, the Chinese government uses technology to follow Uighurs. Cameras are used to keep track of where Uighurs go and where they stand. "A new era of automated racism,", according to t he New York Times .
Second wave
I myself was also on a panel during the session in the European parliament. One of my points was that we should not only want to make technology fairer but also ask ourselves whether we want to use certain technology in the first place.
Facial recognition is a good example. The software is currently struggling with black faces, which it often does not recognise. Is it necessary to supplement the unrepresentative databases with photos, so that a dark complexion can also be recognised?
This sometimes leads to strange practices, such as a company that had to collect photos of dark-skinned people for Google. They reportedly mislead the homeless, among others, to have their faces scanned.
But even if the material is collected ethically, it can be used for immoral purposes. This can be seen in the example of the Chinese government. So do you want to use facial recognition at all?
Frank Pasquale recently published a beautiful blog post about "the second wave of algorithmic accountability". In the first wave, scientists, journalists and lawyers wondered how algorithms could become fairer. The second wave is about whether you want to use them at all.
"Though at first besotted with computational evaluations of persons, even many members of the corporate and governmental establishment now acknowledge that data can be biased, inaccurate, or inappropriate."
And now we have to keep going, according to Pasquale. He quotes Julia Powles and Helen Nissenbaum: "What systems deserve to be built? What problems need to be solved? Who can best build them? And who decides?"
In 2020, the European commission will present a plan for artificial intelligence. Let’s see what they come up with.
Why are so many AI systems named after muppets?
Elmo, Grover, Big Bird, two Ernies, plus an Ernie 2.0 ... There are a lot of AI systems named after muppets. But why? I read a nice article on that topic on the Verge.
It’s about machine-learning models that do something with language. It all started with "Embeddings from Language Models" from the Allen Institute in 2017. With just a little bit of imagination, you can shorten that to Elmo.
The following year, Google released Bidirectional Encoder Representations from Transformers. Yup, there is your Bert. And so it continued in the years that followed. This type of AI is now called "Muppetware".
Is it just a joke? No, says James Vincent, the author of the piece. It shows how different teams rely on each other’s work in developing AI and refer to each other by giving their own work a Muppet name as well.
I’m looking forward to Oscar the Grouch.
Before you go...
Economist Sendhil Mullainathan wrote an op-ed that makes you pause for thought: "Biased Algorithms Are Easier to Fix Than Biased People".
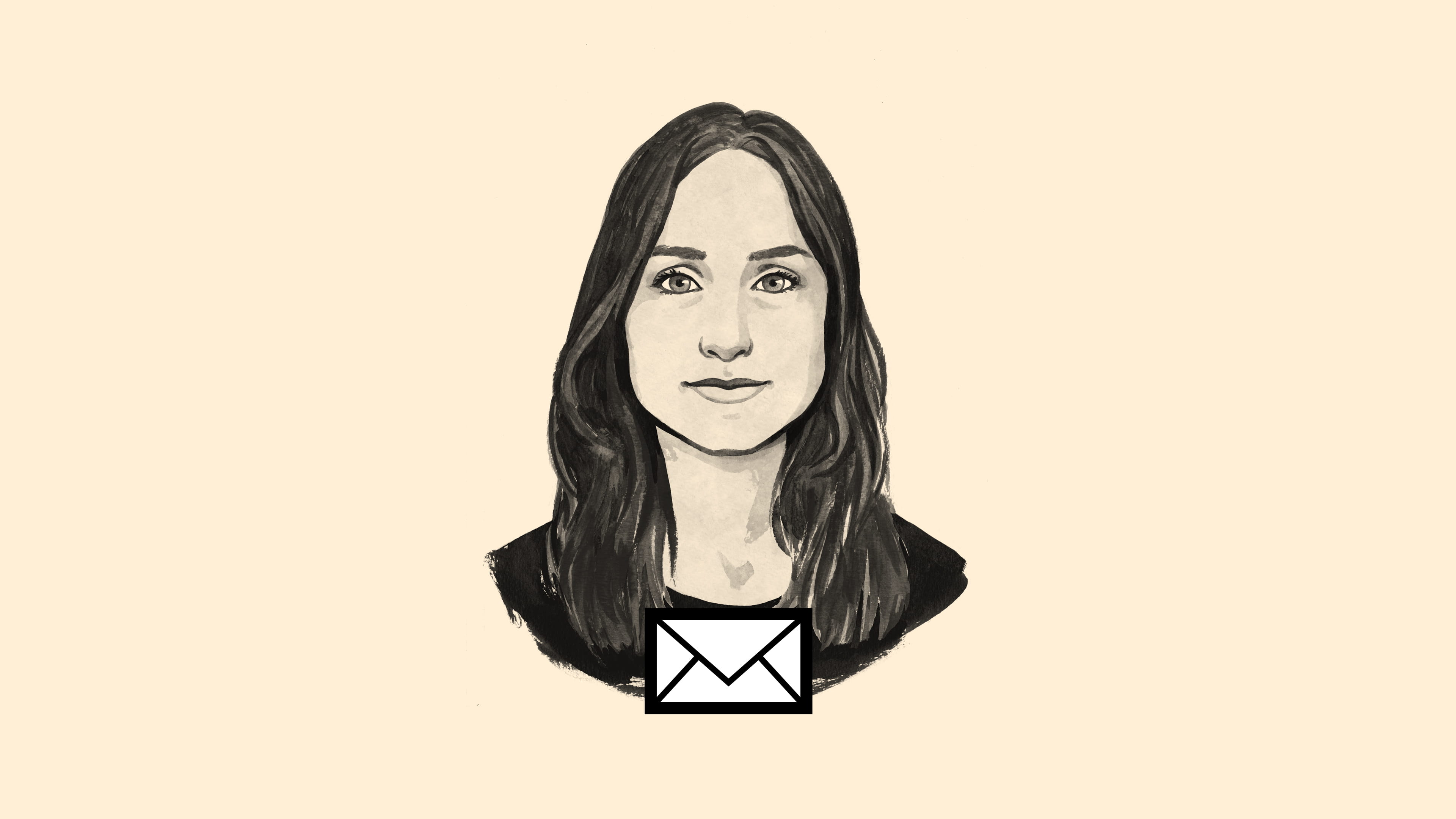